CDINet: Content Distortion Interaction Network for Blind Image Quality Assessment
IEEE Transactions on Multimedia(2024)
摘要
Perceptual image quality is related to content and distortion. Distortion classification is a common way to learn distortion information. How to extract distortion information consistent with human perception is a problem to be solved. Besides, the joint effect on image quality caused by the interplay of content and distortion has not been fully studied. In this paper, a novel Content Distortion Interaction Network (CDINet) is proposed for blind image quality assessment. Distortion representation are guided by content representation to learn quality-aware representation. CDINet consists of four components: a Distortion-Aware Module (DAM), a Content-Aware Module (CAM), an Asymmetric Content-Distortion Interaction (ACDI) module, and a quality regression module. The content representation and distortion representation are extracted respectively and fused interactively in CDINet. Specifically, with the assistance of image restoration, distortion representation consistent with human perception is learned. To further improve the ability in distortion representation, the DAM is used to construct the differences between the distorted image and its reference image. The proposed ACDI module enables the interaction of content and distortion representations to occur at different levels with less computational cost. Since the proposed CDINet considers the joint impact on image quality caused by the interplay of content and distortion, the predicted image qualities highly align with human perception. Comprehensive experiments on 8 benchmark datasets demonstrate that the proposed CDINet effectively extracts quality-aware representation, achieving state-of-the-art performance in evaluating both synthetically and authentically distorted images.
更多查看译文
关键词
Blind image quality assessment,content distortion interaction,image restoration,deep neural networks
AI 理解论文
溯源树
样例
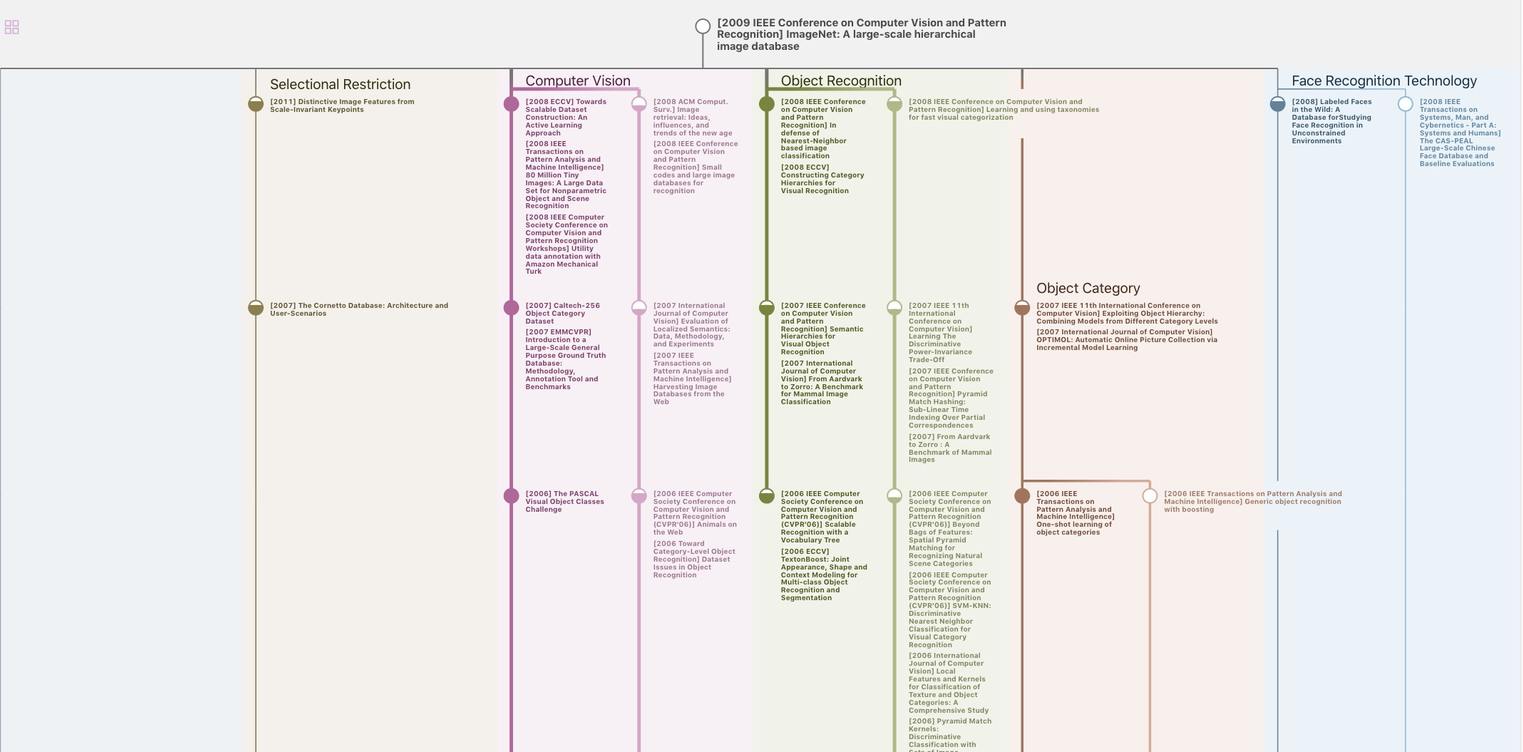
生成溯源树,研究论文发展脉络
Chat Paper
正在生成论文摘要