Runge-Kutta Guided Feature Augmentation for Few-Sample Learning
IEEE Transactions on Multimedia(2024)
摘要
Deep Neural Networks (DNNs) have primarily been demonstrated to be successful when large-scale labeled data are available. However, DNNs usually fail when tasked in few-sample learning scenarios, and the results will be much worse when the limited data show large intra-class variation and inter-class similarity (a.k.a fine-grained classification). To solve this challenging task, the idea of carrying out feature augmentation is visited and better achieved by exploring the merit of the forward Euler method in solving ordinary differential equations (ODEs), and a novel high-order feature augmentation (HFA) model with ResNet is proposed. Specifically, the proposed method leverages the stacked residual structure to model the direction of feature change over the initial state, and uses the triplet loss as constraint to model the step size of change in an adaptive manner. As a result, the initial features can then be augmented by a residual structure with a forward Eulerian form to generate features of the same subcategory with a similar representation as the input image. Furthermore, the proposed augmentation mechanism enjoys two additional benefits: a) it can help avoid the over-fitting issue when learned with insufficient training data; b) it can be used seamlessly with any residual structure-based classification network, and the ResNet used in this paper remains unchanged during testing. Extensive experiments are carried out on fine-grained visual categorization benchmarks, and the results demonstrate that our approach can significantly improve the categorization performance when the training data is highly insufficient.
更多查看译文
关键词
Runge-Kutta method,feature augmentation,few-sample learning
AI 理解论文
溯源树
样例
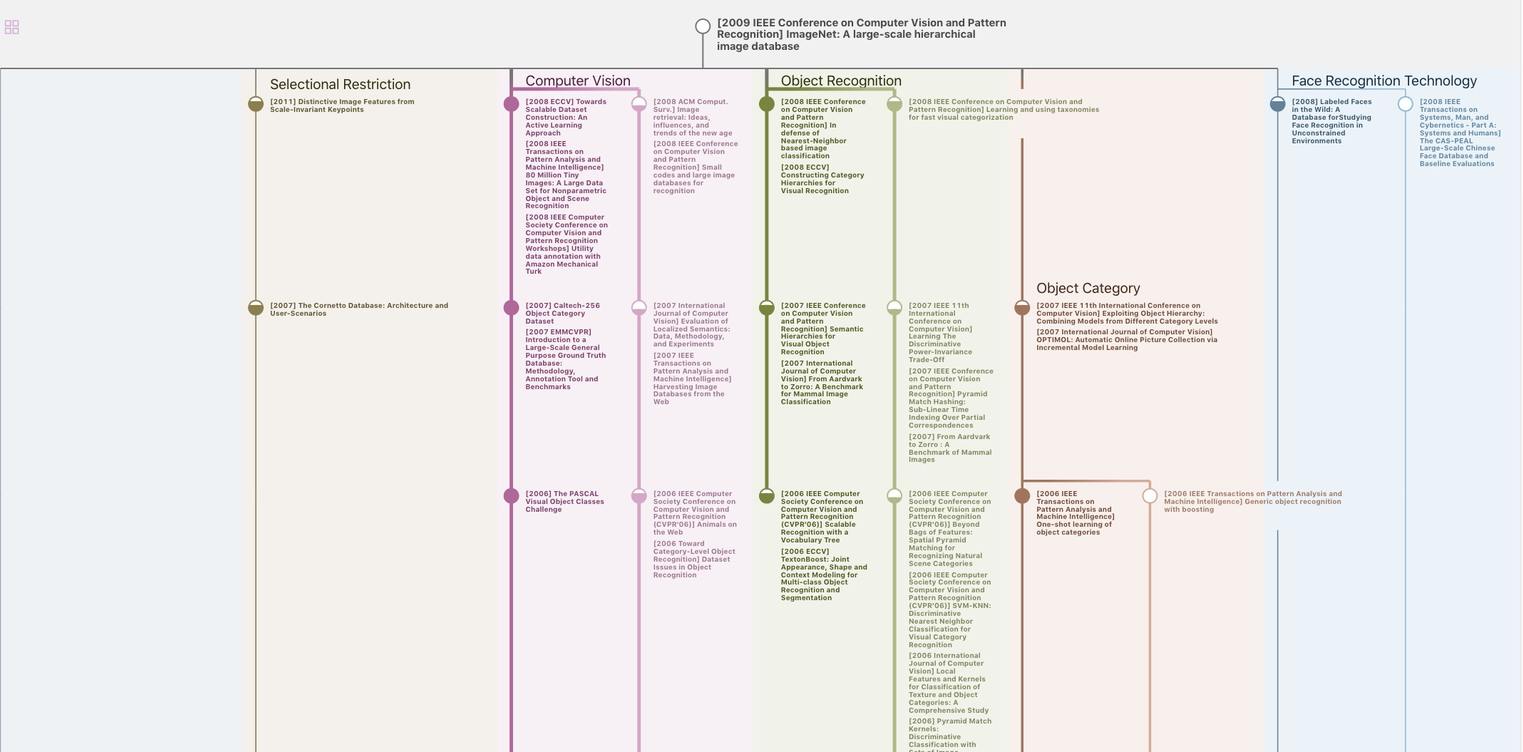
生成溯源树,研究论文发展脉络
Chat Paper
正在生成论文摘要