IM-META : Influence Maximization Using Node Metadata in Networks With Unknown Topology
IEEE Transactions on Network Science and Engineering(2024)
摘要
Since the structure of complex networks is often
unknown
, we may identify the most influential seed nodes by exploring only a part of the underlying network, given a small budget for node queries. We propose
IM-META
, a solution to influence maximization (IM) in networks with unknown topology by retrieving information from queries and node metadata. Since using such metadata is not without risk due to the noisy nature of metadata and uncertainties in connectivity inference, we formulate a new IM problem that aims to find both seed nodes and queried nodes. In
IM-META
, we develop an effective method that
iteratively
performs three steps: 1) we learn the relationship between collected metadata and edges via a
Siamese neural network
, 2) we select a number of inferred confident edges to construct a
reinforced
graph, and 3) we identify the next node to query by maximizing the inferred influence spread using our
topology-aware
ranking strategy. Through experimental evaluation of
IM-META
on four real-world datasets, we demonstrate a) the speed of network exploration via node queries, b) the effectiveness of each module, c) the superiority over benchmark methods, d) the robustness to more difficult settings, e) the hyperparameter sensitivity, and f) the scalability.
更多查看译文
关键词
Influence maximization,network inference,node medadata,node query,Siamese neural network,topologically unknown network
AI 理解论文
溯源树
样例
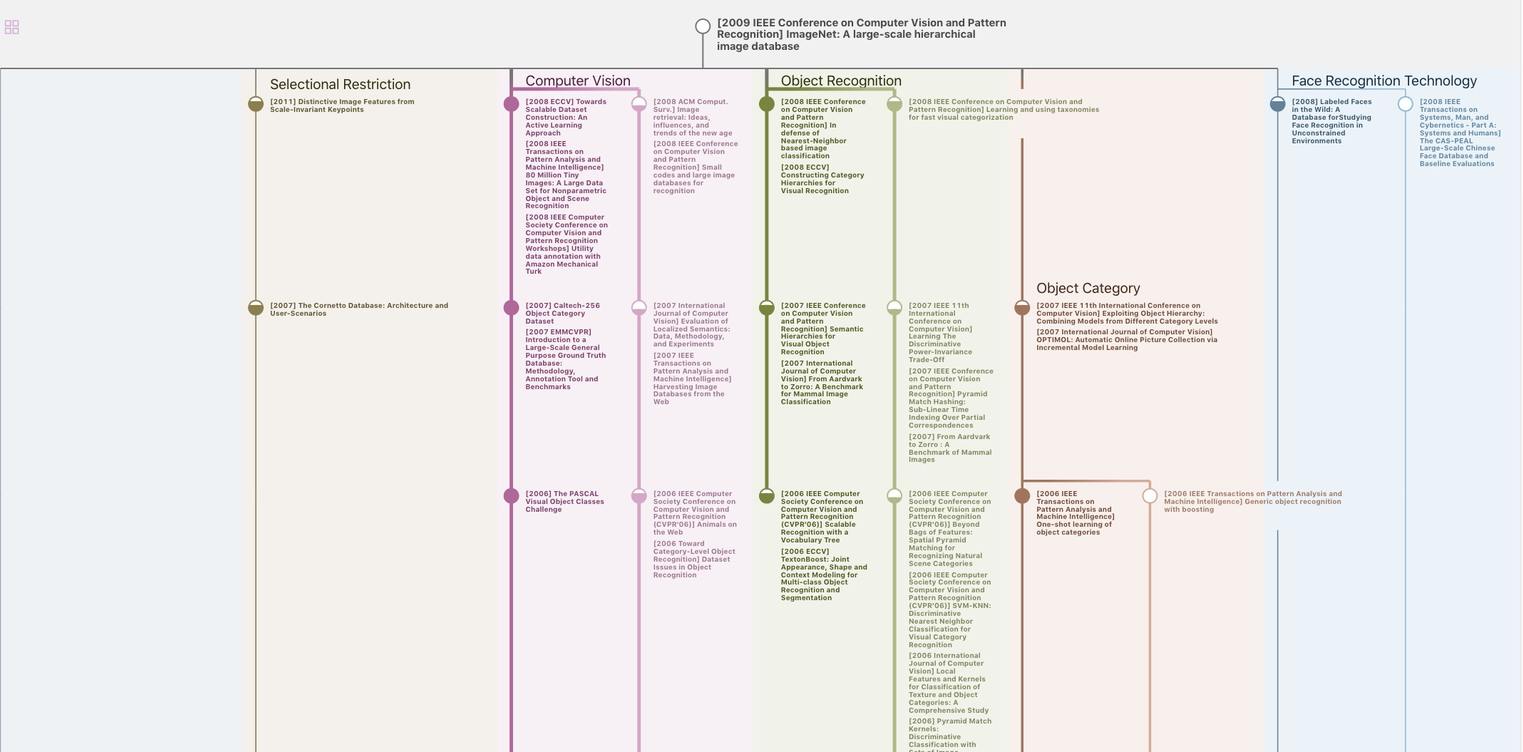
生成溯源树,研究论文发展脉络
Chat Paper
正在生成论文摘要