Ferrari: A Personalized Federated Learning Framework for Heterogeneous Edge Clients
IEEE Transactions on Mobile Computing(2024)
摘要
Federated semi-supervised learning (FSSL) has been proposed to address the insufficient labeled data problem by training models with pseudo-labeling. In previous FSSL systems, a single global model is always trained without an equivalent generalization ability for the clients under the non-IID setting. Accordingly, model personalization methods have been proposed to overcome this problem. Intuitively, seeking labeling assistance from other clients with similar data distribution,
i.e.
, model migration, can effectively improve the personalization on the clients with scarce labeled data. However, previous works require to migrate a pre-fixed number of models among the clients, causing unnecessary resource waste and accuracy degradation due to resource heterogeneity. Considering that the number of model migrations and the quality of pseudo-labels have a significant impact on the training performance (
e.g.
, efficiency and accuracy), we propose a novel personalized FSSL system, called Ferrari, to boost the efficiency of pseudo-labeling and training accuracy through adaptive model migrations among the clients. Specifically, Ferrari first generates the similarity-based ranking using a Gaussian KD-Tree, considering the varied data distributions among the clients. Combined with the ranking and clients' heterogeneous resource constraints, Ferrari then adaptively determines the proper model migration policy and confidence thresholds for high-quality pseudo-labeling and personalized training for clients. Extensive experiments on a physical platform show that Ferrari provides a 1.2
$\sim 5.5\times$
speedup without sacrificing model accuracy, compared to existing methods.
更多查看译文
关键词
Federated Semi-Supervised Learning,Personalization,Model Migration,Edge Computing
AI 理解论文
溯源树
样例
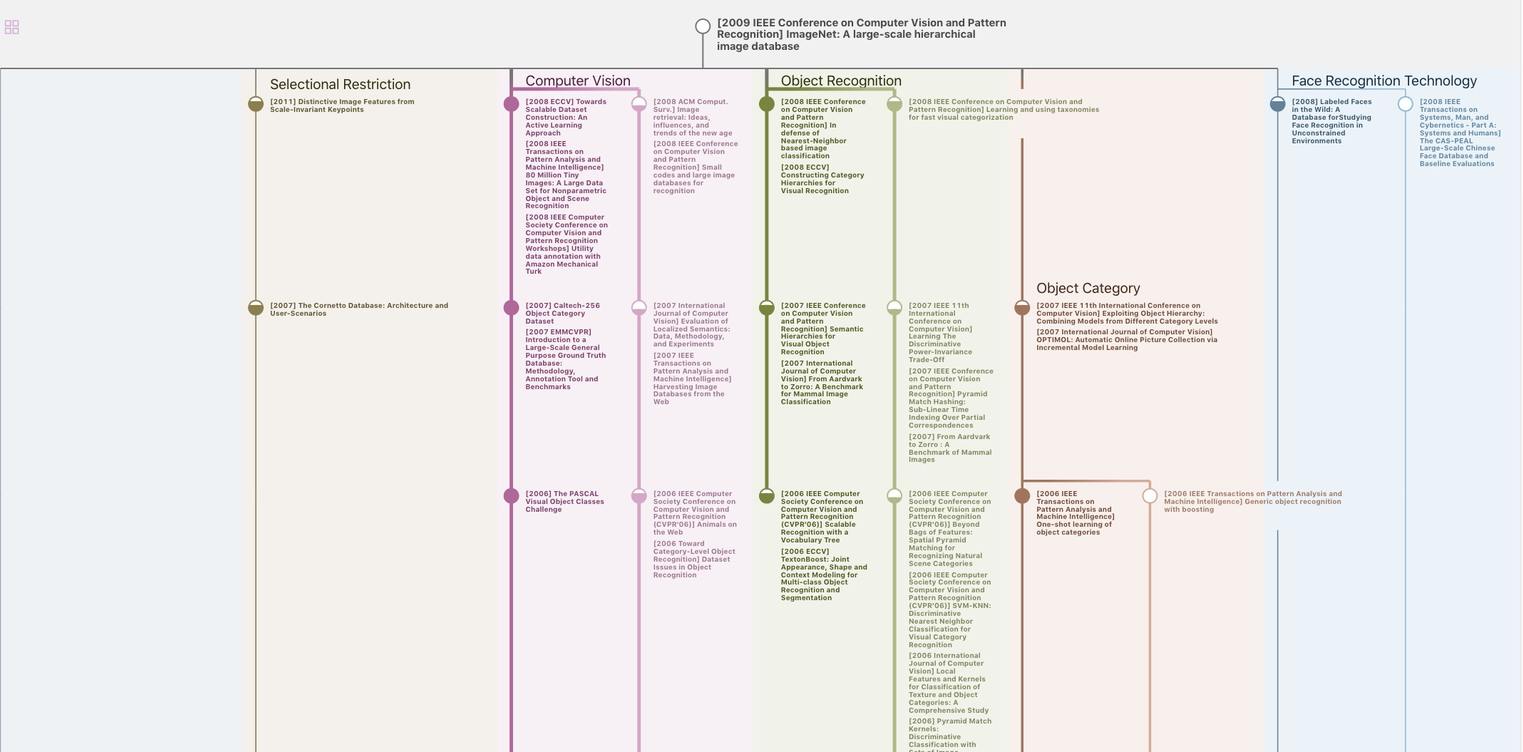
生成溯源树,研究论文发展脉络
Chat Paper
正在生成论文摘要