A Mutually Textual and Visual Refinement Network for Image-Text Matching.
IEEE Trans. Multim.(2024)
摘要
Image-text matching is vital important in the field of multi-modal intelligence. Recently, it is advocated in a way that decomposes images and texts into local fragments and followed by region-word aligning. As a result, the image-text relevance score is given by aggregating semantic similarities between matched region-word pairs. Despite effectiveness, this strategy fails to express data relations exactly. From the perspective of the text side, text words decomposed from a concise language sentence usually have limited contextual information, which can result in semantic identical but actually false text-region alignments. From the perspective of the image side, semantic ambiguity that multiple objects share the same semantic meaning can further exacerbate this problem. In this manuscript, we introduce a mutually Textual and Visual Refinement Network (TVRN), to tackle the inaccurate cross-modal alignment problem. In a nutshell, TVRN improves inter-modal matching by improving contextual information in sentences meanwhile reduces semantic ambiguity in images to capture the maximized relevant relations. More specifically, we develop a new module that integrates visual contextual clues into the text modality to generate informational text features with richer geometric contexts. Mutually, we further design a semantic alignment enhancement module that leverages consensus affinity of local image and text features to guide deeper semantic image embedding with the supervision of global image vectors. At the image-text matching stage, similarities at the local and global levels are integrated to capture coarse-grained and fine-grained interactions between vision and language. A large number of experiments on Flickr30K and MS-COCO benchmarks demonstrate that TVRN is superior to existing methods.
更多查看译文
关键词
Cross-modal retrieval,Image-text matching,Contextual enhancement,Semantic alignment enhancement
AI 理解论文
溯源树
样例
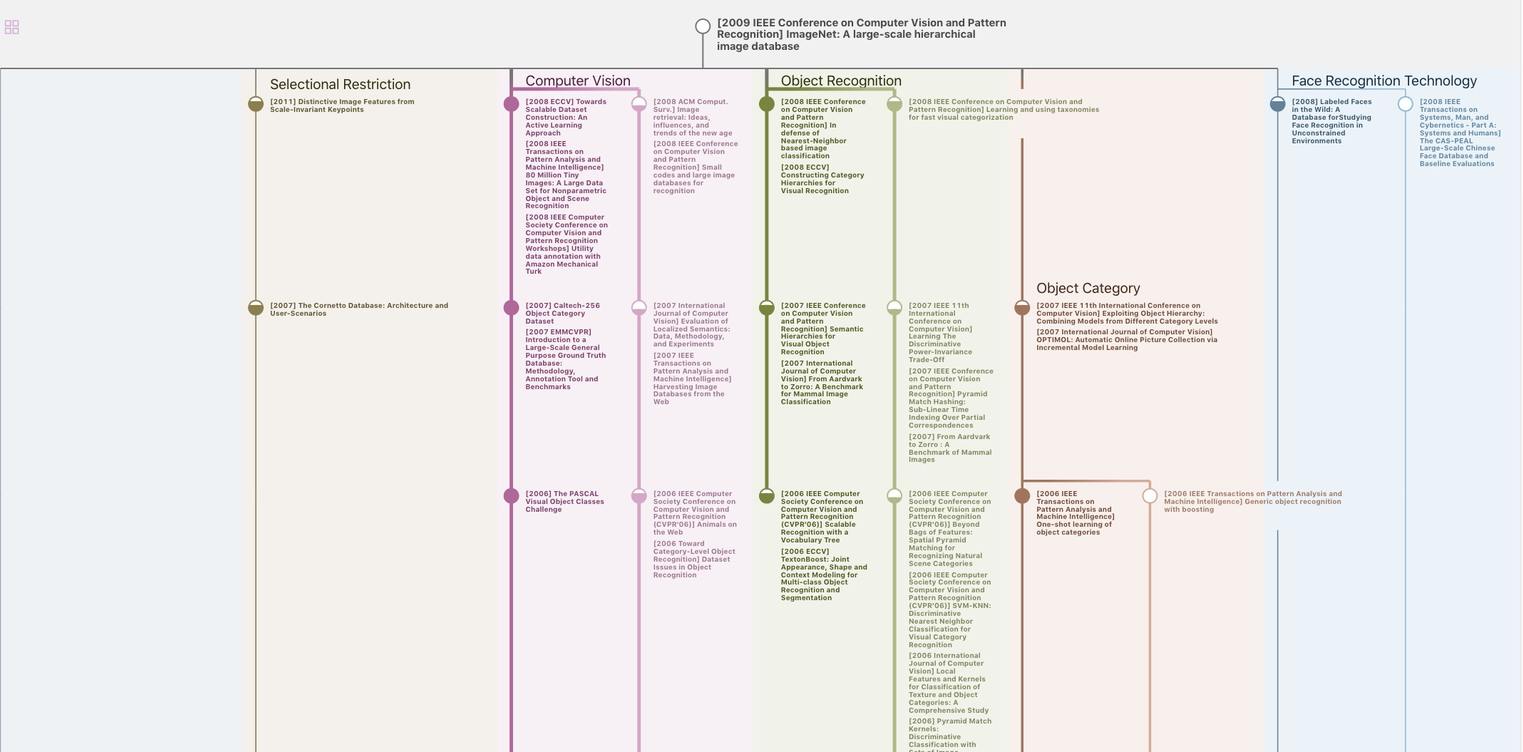
生成溯源树,研究论文发展脉络
Chat Paper
正在生成论文摘要