LCFNets: Compensation Strategy for Real-time Semantic Segmentation of Autonomous Driving
IEEE Transactions on Intelligent Vehicles(2024)
摘要
Semantic segmentation is an important research topic in the environment perception of intelligent vehicles. Many semantic segmentation networks based on bilateral architecture have been proven effective. However, semantic segmentation networks based on this architecture has the risk of pixel classification errors and small objects being overwhelmed. In this paper, we solve the problem by proposing a novel three-branch architecture network called LCFNets. Compared to existing bilateral architecture, LCFNets introduce compensation branch for the first time to preserve the features of original images. Through two efficient modules, Lightweight Detail Guidance Fusion Module (L-DGF) and Lightweight Semantic Guidance Fusion Module (L-SGF), detail and semantic branches are allowed to selectively extract features from this branch. To balance the three-branch features and guide them to fuse effectively, a novel aggregation layer is designed. Depth-wise Convolution Pyramid Pooling module (DCPP) and Total Guidance Fusion Module (TGF) enable the aggregation layer to extract the global receptive field and realize multi-branch aggregation with fewer calculation complexity. Extensive experiments on Cityscapes and CamVid datasets have shown that our family of LCFNets provide a better trade-off between speed and accuracy. With the full resolution input and no ImageNet pre-training, LCFNet-slim achieves 76.86% mIoU at 114.36 FPS and LCFNet achieves 77.96% mIoU at 92.37 FPS on Cityscapes. On the other hand, LCFNet-super achieves 79.10% mIoU at 47.46 FPS. Source code can be found from the:
https://github.com/yiwen-bai/LCFNets
.
更多查看译文
关键词
Compensation strategy,Multi-branch aggregation,Real-time semantic segmentation,Autonomous driving
AI 理解论文
溯源树
样例
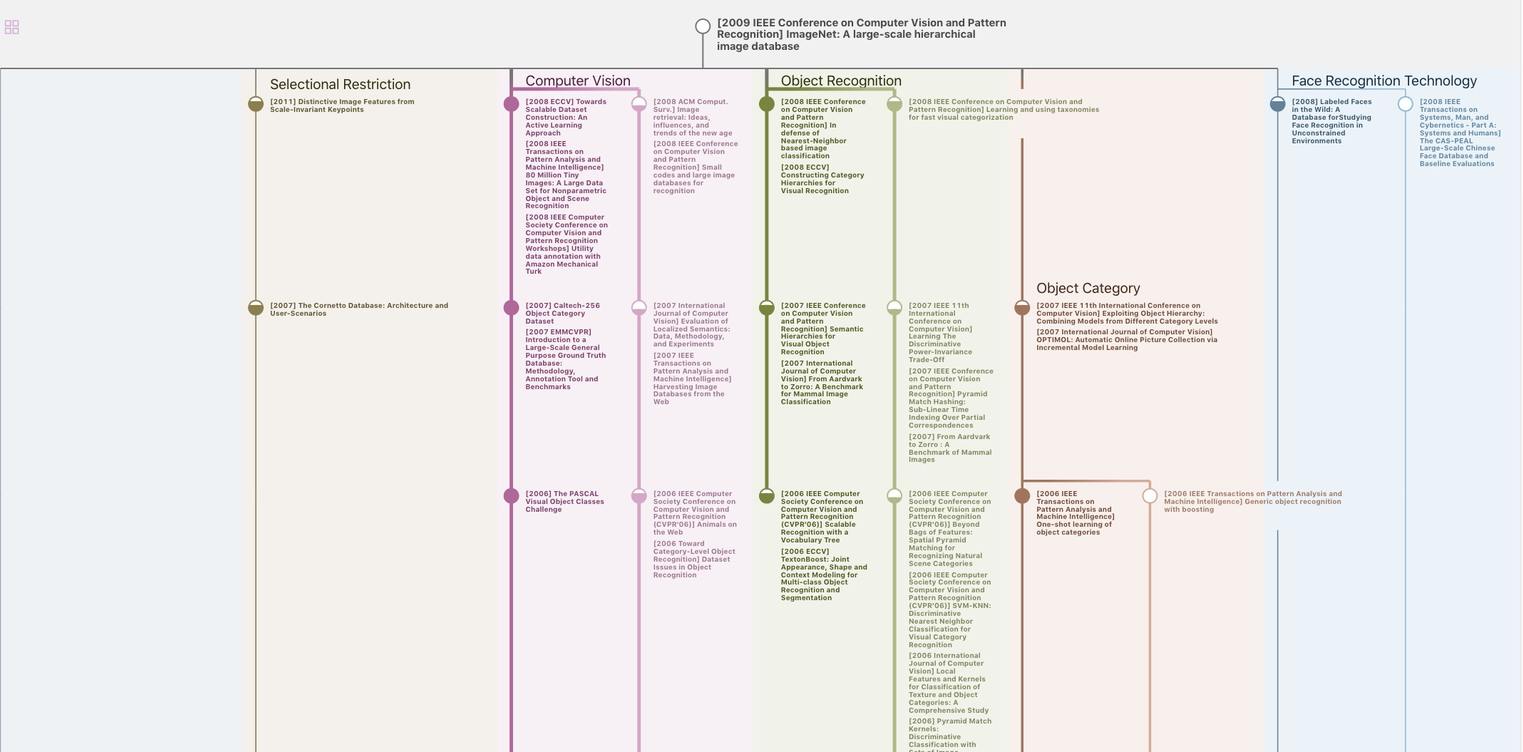
生成溯源树,研究论文发展脉络
Chat Paper
正在生成论文摘要