Maximum Entropy Inverse Reinforcement Learning Using Monte Carlo Tree Search for Autonomous Driving
IEEE TRANSACTIONS ON INTELLIGENT TRANSPORTATION SYSTEMS(2024)
摘要
Autonomous vehicles must be capable of driving safely and having some level of social compliance with human drivers. Acting egoistically can make other drivers to take undesirable actions, such as performing hard brakes to avoid collisions. Designing a proper behavior involves dealing with antagonistic objectives, such as increasing speed and avoiding rear-end collisions. However, weighting those objectives in a reward or cost function is an error-prone and time-consuming task, and can become very hard as more features are added to the problem. In this regard, the main objective of this paper is to use learning from demonstration to compute trajectories that mimic human expert behavior without the need of manually tuning a reward function. On this subject, we present a variation of the well-known Maximum Entropy Inverse Reinforcement Learning algorithm in order to deal with continuous state spaces: instead of exactly computing the gradients, they are estimated by sampling trajectories in regions with higher rewards using a Monte Carlo Tree Search based sampler. The sampler is applied to solve an interaction-aware Markov Decision Problem capable of dealing with the inherent interaction and uncertainty present in surrounding vehicles motion. Experiments are performed in a merging scenario considering real data, showing that the proposed method can generate trajectories similar to the ones executed by human drivers. Additionally, favorable results are achieved when compared to traditional baseline methods and also to a variant of Inverse Reinforcement Learning that uses a polynomial-curve trajectory sampler.
更多查看译文
关键词
Trajectory,Behavioral sciences,Entropy,Autonomous vehicles,Vehicles,Cost function,Task analysis,merging,interaction-aware decision-making,MCTS,IRL
AI 理解论文
溯源树
样例
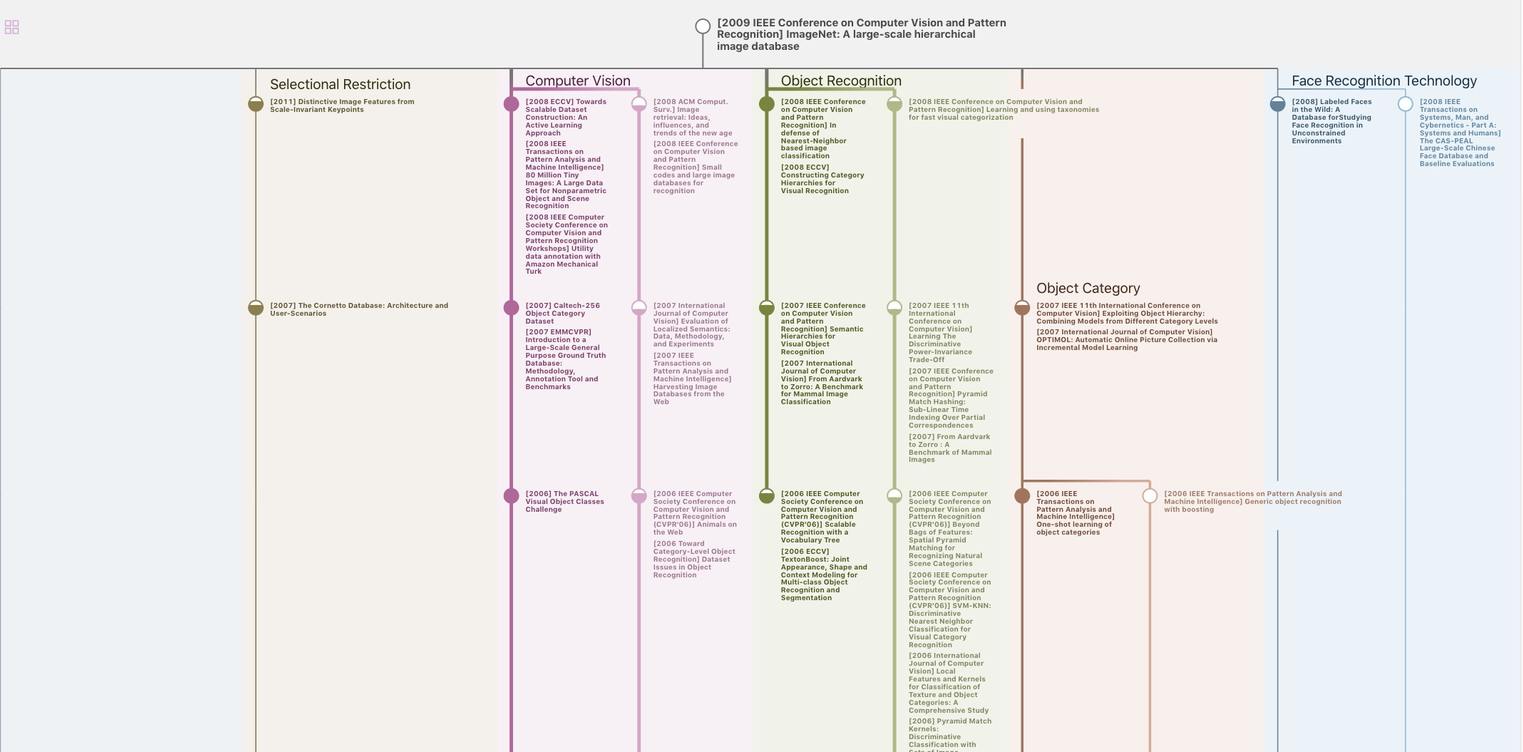
生成溯源树,研究论文发展脉络
Chat Paper
正在生成论文摘要