FDSP-HRID: Few-Shot Detector With Self-Supervised Pretraining for High-Speed Rail Infrastructure Defects.
IEEE Trans. Instrum. Meas.(2024)
摘要
Defect detection for the infrastructure of high-speed rails is key to the rails’ safety. In recent years, such detection has been based on deep learning with a substantial amount of labeled samples. But, the defect samples of high-speed rail infrastructure are few, resulting in insufficient training data. Meanwhile, the features provided by supervised pretraining are not targeted at the complex backdrop of rail operation. To make up for insufficient data and un-targeted features, we propose a few-shot detector with self-supervised pretraining for high-speed rail infrastructure defects (FDSP-HRID). Our approach incorporates three stages. First, we undertake self-supervised pretraining with a large amount of unlabeled infrastructure data. After that, we transfer the backbone network weight onto the few-shot base detector. Then, we use a large amount of defect-free, base class data to train each layer of the detector. We use the multiscale attention mechanism based on squeeze and excitation network (SE-MAM) to improve the network’s recognition of small objects and the model’s sensitivity to channel features. In this case, the context semantic fusion module (CSF) effectively fuses features of different scales and learns global and local features for comprehensive feature representation. At last, we use a small amount of novel class defect data to fine-tune the detector and run the detector on the test set. Experimental results show that our approach achieves mAP50 of 27.47% and 34.08% for 1 and 5 shots on the dataset of drone images captured ourselves, meaning that our approach achieves an obvious advantage over other approaches.
更多查看译文
关键词
high-speed rail infrastructure,self-supervised learning,few-shot learning,drone image,defect detection
AI 理解论文
溯源树
样例
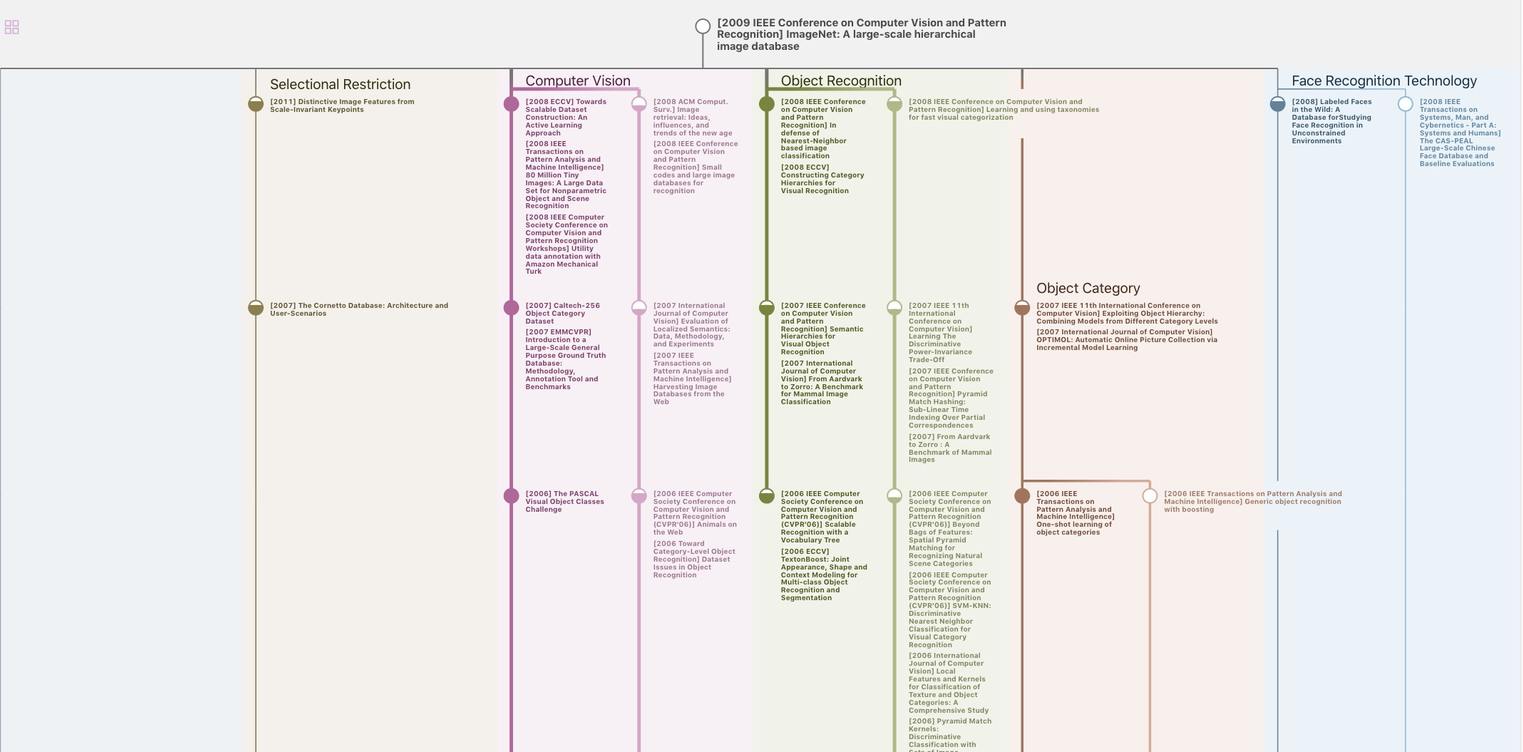
生成溯源树,研究论文发展脉络
Chat Paper
正在生成论文摘要