PolarPoint-BEV: Bird-eye-view Perception in Polar Points for Explainable End-to-end Autonomous Driving
IEEE Transactions on Intelligent Vehicles(2024)
摘要
End-to-end autonomous driving has attracted great attentions in recent years. Compared to traditional modular methods, end-to-end methods are more scalable in complex traffic environments, but they lack explainability. Many methods have been proposed to increase the explainability for end-to-end autonomous driving, such as using semantic bird-eye-view (BEV) maps. BEV maps can explain the outputs of end-to-end methods by showing how the networks perceive and understand surrounding traffic environments. However, there are some limitations in traditional semantic BEV maps. For instance, all regions of traffic scenes are treated equally, but the fact is that the regions near the ego vehicle are normally more critical to vehicle safety. Moreover, traditional BEV maps represent traffic scenes in the fine-grained pixel-level mode, which leads to much computational cost. To address these issues, we introduce a novel lightweight BEV perception method, PolarPoint-BEV, which prioritizes the regions according to object distances to the ego vehicle. Furthermore, we propose an explainable end-to-end autonomous driving network to investigate the influence of our PolarPoint-BEV in terms of driving performance. Experimental results demonstrate that our PolarPoint-BEV improves both the driving capability and explainability of the network.
更多查看译文
关键词
End-to-end Autonomous Driving,BEV Perception,Explainable AI (XAI)
AI 理解论文
溯源树
样例
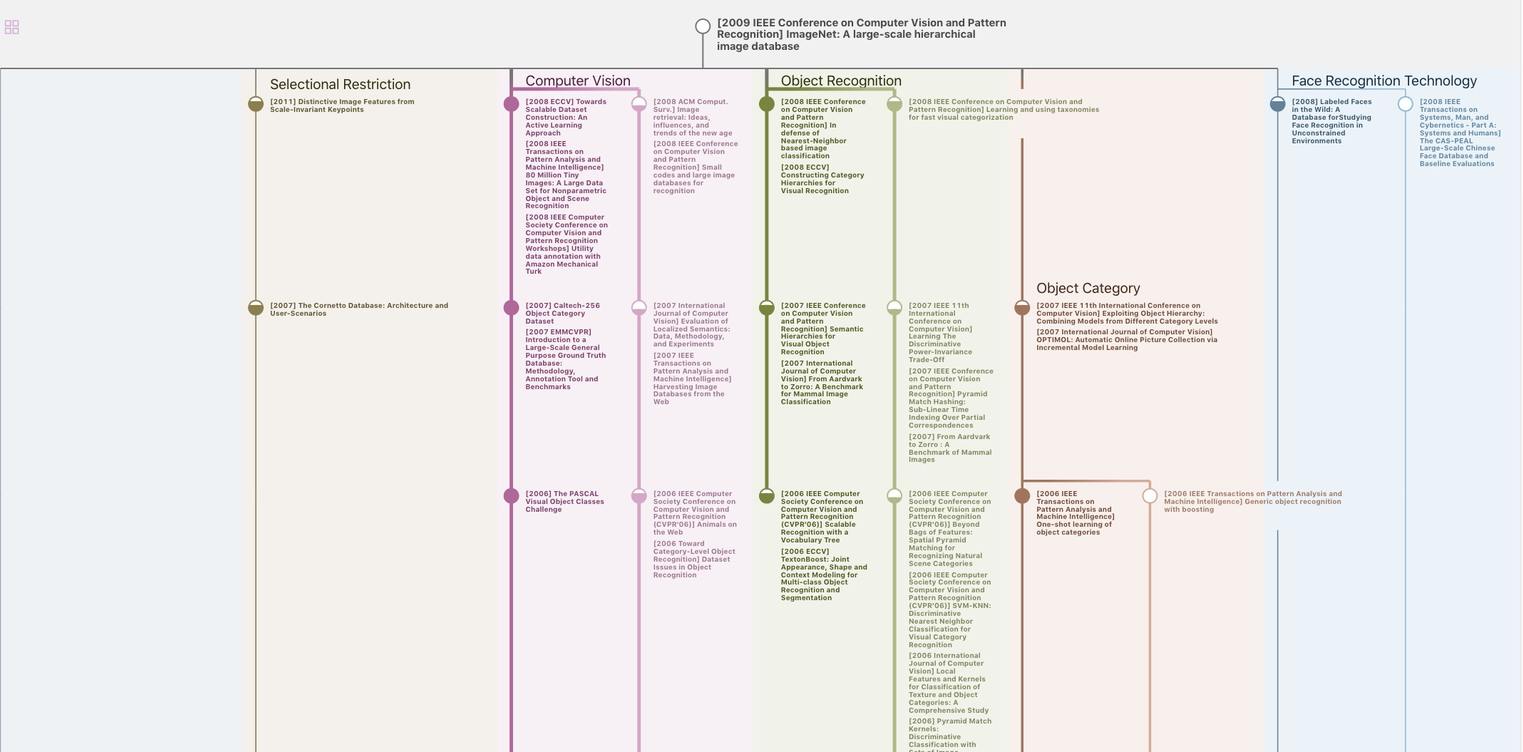
生成溯源树,研究论文发展脉络
Chat Paper
正在生成论文摘要