SELM: Self-Motivated Ensemble Learning Model for Cross-Domain Few-Shot Classification in Hyperspectral Images
IEEE GEOSCIENCE AND REMOTE SENSING LETTERS(2024)
摘要
Hyperspectral image (HSI) classification is a common task in remote sensing that often faces challenges due to limited samples and cross-domain discrepancies between training and test data. This particular problem is termed as HSI cross-domain few-shot classification (HSI-CFSC). To solve this problem, we propose a self-motivated ensemble learning model (SELM). Building upon source pre-trained representations, our end-to-end approach comprises a self-training paradigm to iteratively refine target representations independent of direct source supervision. Moreover, an ensemble classifier suite leveraging diverse decision boundaries is optimized to excavate comprehensive classification cues from limited labeled target data. The overall accuracy (OA), average accuracy (AA), and Kappa of SELM in UP, PC, and Salinas datasets are, respectively, 86.55%, 82.27%, 82.10%, 98.07%, 94.20%, 97.30%, and 91.33%, 94.96%, 90.37%, which achieve the state-of-art performance compared with other classical methods.
更多查看译文
关键词
Feature extraction,Training,Ensemble learning,Adaptation models,Probabilistic logic,Predictive models,Iterative methods,Cross-domain,ensemble learning,few-shot classification,hyperspectral image (HSI),self-training
AI 理解论文
溯源树
样例
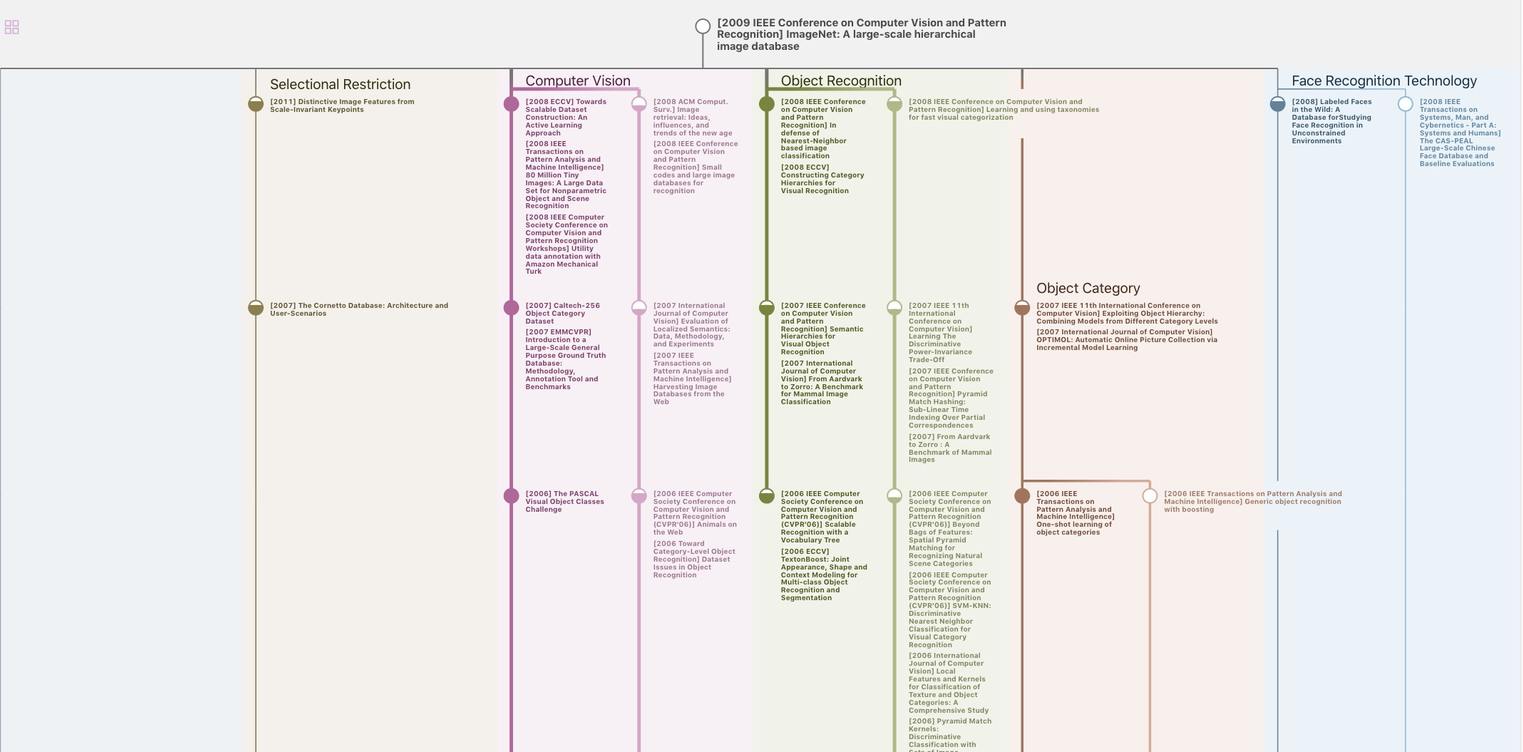
生成溯源树,研究论文发展脉络
Chat Paper
正在生成论文摘要