Intelligent Highway Adaptive Lane Learning System in Multiple ROIs of Surveillance Camera Video
IEEE TRANSACTIONS ON INTELLIGENT TRANSPORTATION SYSTEMS(2024)
摘要
US Department of Transportation (DOT) operators commonly use adjustable surveillance cameras for traffic monitoring and desire to have an automated traffic counting system by lane. To fill this need, this paper describes an automatic, novel, multiple-ROI (Regions of Interest) lane learning (MRLL) system. It detects lane centers, boundaries, and traffic directions, irrespective of zoom or direction. It finds optimal ROIs without user input by analyzing confidence scores from a chosen Machine Learning (ML) object detector. A simple but effective Continual Learning strategy is used to control the MRLL's start and stop that optimizes lane counting performance in various real-world conditions: nighttime, extremely harsh weather, or low traffic flow conditions. Tested on 45 varied videos, it achieves an F1_score above 0.79 for lane center detection, 0.88 for lane boundaries, and 94% accuracy in traffic direction detection. This innovative system, which does not rely on lane markings and adapts to camera views, is currently used by the Indiana Department of Transportation for vehicle counting and flow rate estimation in real-world ITS scenarios. Code is available at https://github.com/qiumei1101/Multiple_ROI_lane_learning_ system_for_Highway.git.
更多查看译文
关键词
Adaptive multiple ROIs-learning system,lane center detection,lane direction detection,lane boundary detection,continual lane learning process
AI 理解论文
溯源树
样例
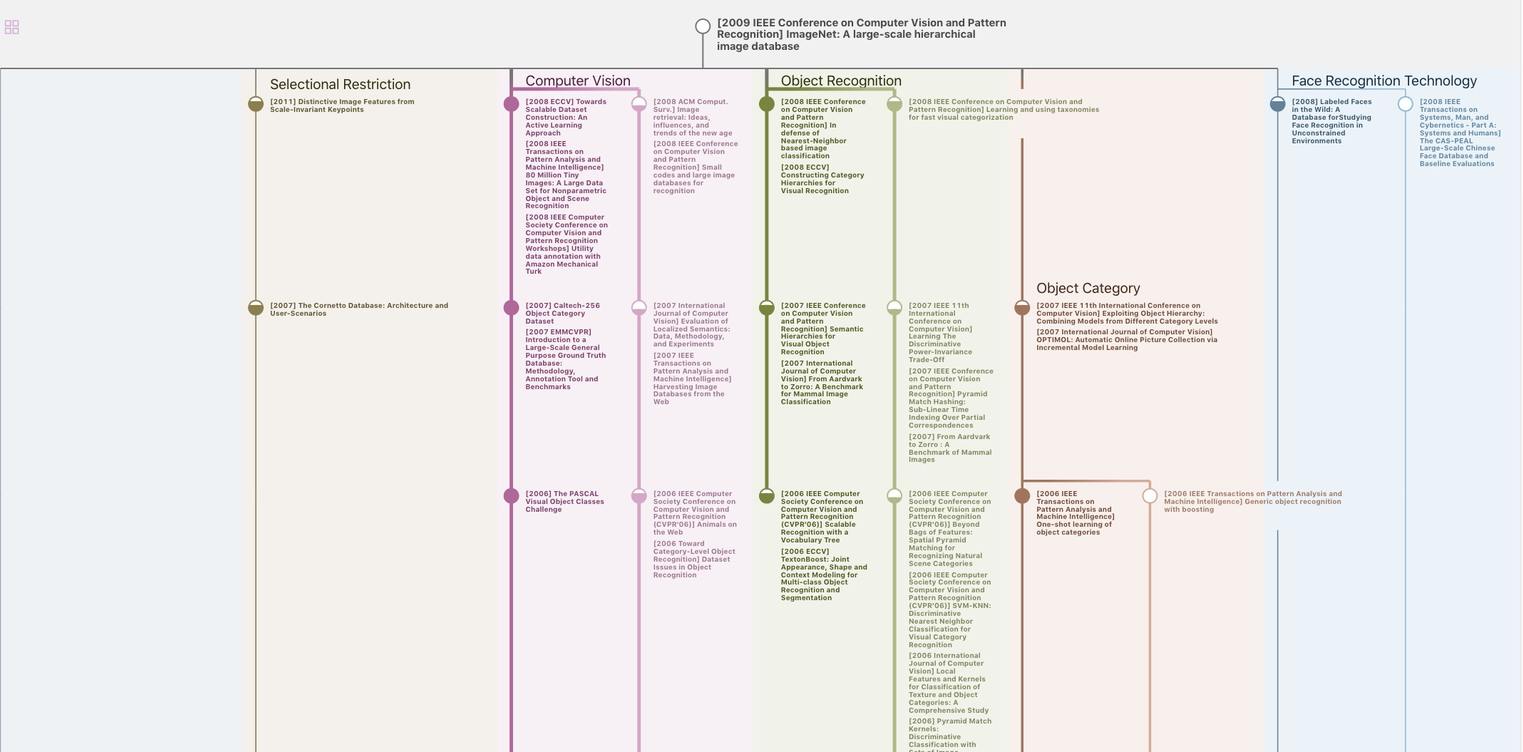
生成溯源树,研究论文发展脉络
Chat Paper
正在生成论文摘要