Transferable Deep Slow Feature Network With Target Feature Attention for Few-Shot Time-Series Prediction
IEEE TRANSACTIONS ON INDUSTRIAL INFORMATICS(2024)
摘要
Data-driven methods for predicting quality variables in wastewater treatment processes (WWTPs) have mostly ignored the slow time-varying nature of WWTP, and they are data-consuming that need a large amount of independent and homogeneously distributed data, which makes it difficult to collect. To address this issue with few-shot and inconsistent distribution, a transfer learning method called transferable deep slow feature network (TDSFN) for time-series prediction is proposed by leveraging the knowledge of relevant datasets. TDSFN extracts nonlinear slow features of WWTP with inertia from the time series through a deep slow feature network and constructs the domain invariant features based on them. Target feature attention is designed in TDSFN to enhance the predictor adaptability to the target domain by assigning weights to the source features based on their similarity to target features. Furthermore, a variational Bayesian inference framework is introduced to learn the parameters of TDSFN. The effectiveness of TDSFN is verified through prediction experiments based on WWTP.
更多查看译文
关键词
Feature extraction,Time series analysis,Training,Probabilistic logic,Bayes methods,Probability distribution,Monitoring,Bayesian inference,gate recurrent unit,slow feature analysis (SFA),transfer learning (TL),wastewater treatment process (WWTP)
AI 理解论文
溯源树
样例
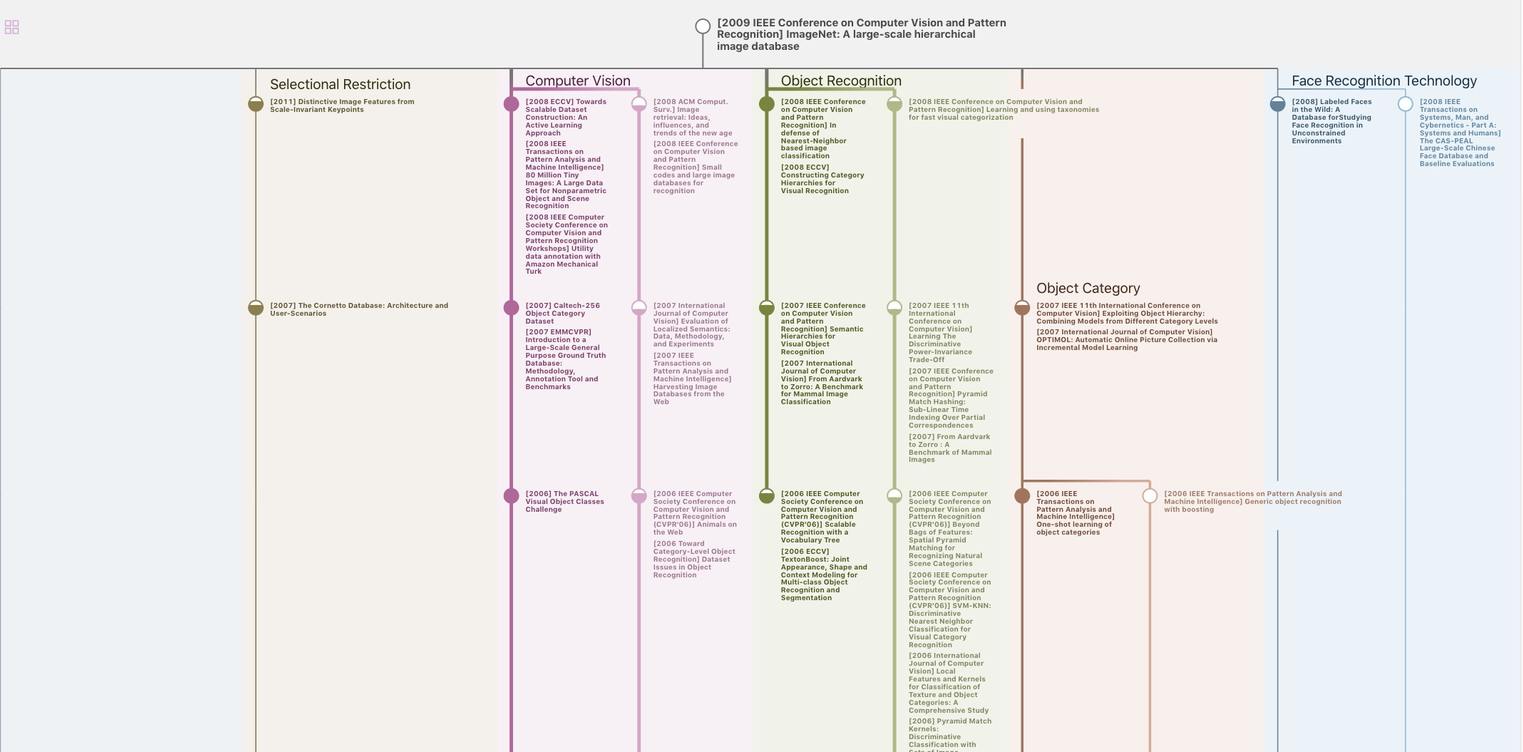
生成溯源树,研究论文发展脉络
Chat Paper
正在生成论文摘要