Global-Context-Aware Visual Odometry System With Epipolar-Geometry-Constrained Loss Function
IEEE TRANSACTIONS ON INSTRUMENTATION AND MEASUREMENT(2024)
摘要
Visual odometry (VO) plays a vital role in simultaneous localization and mapping (SLAM). Most of the current learning-based VO methods utilize convolutional neural network (CNN) as framework. However, CNN is weak in integrating global context information. In the design of loss function, the majority of these methods ignore the restraint relationship between translation and rotation prediction. In this work, we propose an end-to-end global-context-aware visual odometry with epipolar-geometry-constrained loss function (CEGVO) to estimate the relative 6-DoF poses of monocular camera. The proposed scheme designs an augmented-attention-enhanced global context block (GCB) on top of contextual CNN to learn the long-range dependencies and internal correlation. To overcome the problem of mutual restraint between the translation and rotation errors, an epipolar-geometry-constrained loss function is developed to simultaneously improve the prediction accuracy of both translation and rotation. The evaluation results on public datasets and self-collected dataset show that the proposed system outperforms the state-of-the-art (SOAT) learning-based VO methods with a large margin.
更多查看译文
关键词
Deep learning,epipolar geometry,global context information,simultaneous localization and mapping (SLAM),visual odometry (VO)
AI 理解论文
溯源树
样例
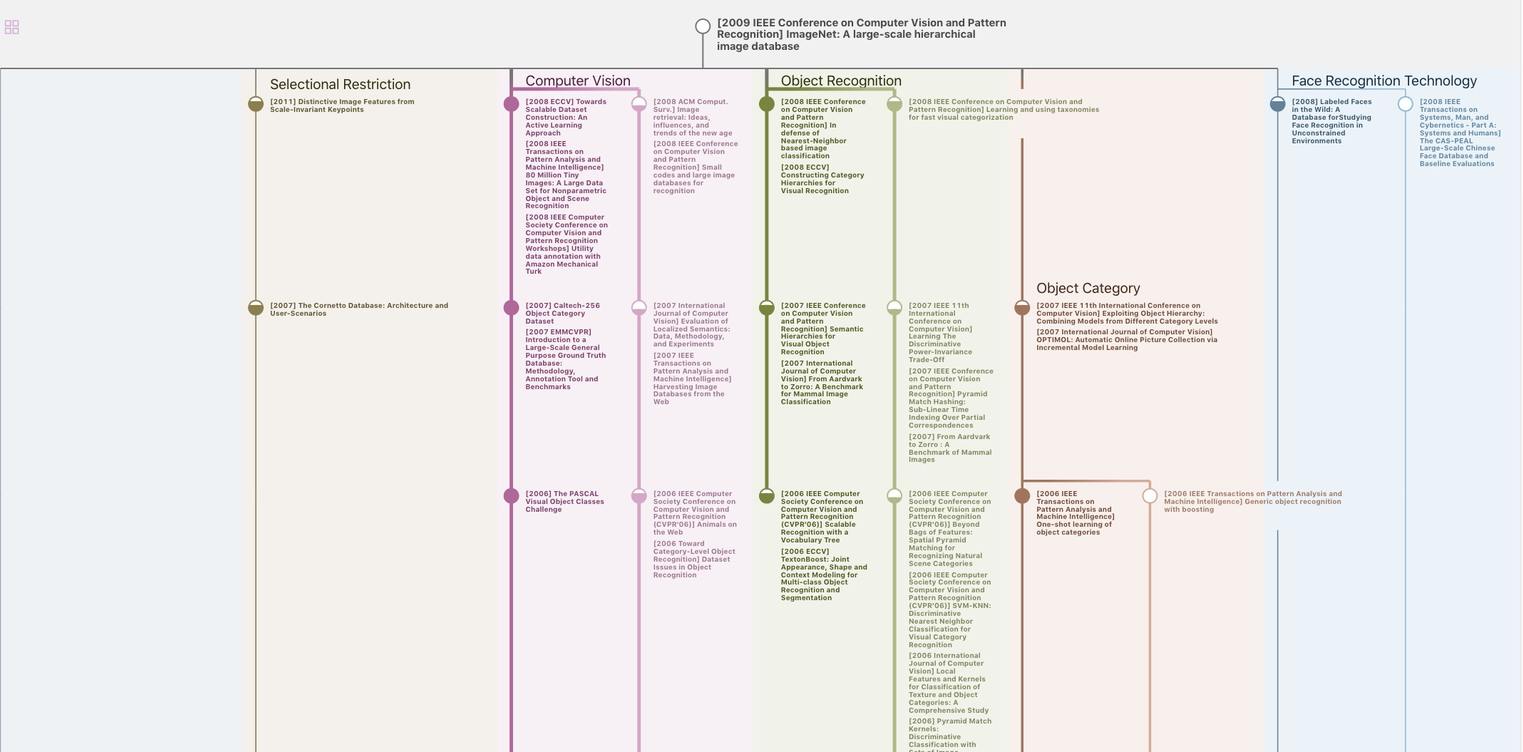
生成溯源树,研究论文发展脉络
Chat Paper
正在生成论文摘要