Multi-Modal Features and Accurate Place Recognition with Robust Optimization for Lidar-Visual-Inertial SLAM
IEEE Transactions on Instrumentation and Measurement(2024)
摘要
Lidar-Visual-Inertial SLAM (LVINS) provides a compelling solution for accurate and robust state estimation and mapping, integrating complementary information from multi-sensor data. However, in the front-end processing of existing LVINS systems, methods based on visual line feature matching typically suffer from low accuracy and are time-consuming. Additionally, the back-end optimization of current multi-sensor fusion SLAM systems is adversely affected by feature association outliers, which constrains further enhancements in localization precision. In the loop closure process, existing lidar loop closure descriptors, relying primarily on 2D information from point clouds, often fall short in complex environments. To effectively tackle these challenges, we introduce the Multi-Modal Feature-based Lidar-Visual-Inertial SLAM framework, abbreviated as MMF-LVINS. Our framework consists of three major innovations. Firstly, we propose a novel coarse-to-fine visual line matching method that utilizes geometric descriptor similarity and optical flow verification, substantially improving both efficiency and accuracy of line feature matching. Secondly, we present a robust iterative optimization approach featuring a newly proposed adaptive loss function. This function is tailored based on the quality of feature association and incorporates graduated non-convexity, thereby reducing the impact of outliers on system accuracy. Thirdly, to augment the precision of lidar-based loop closure detection, we introduce an innovative 3D lidar descriptor that captures spatial, height, and intensity information from the point cloud. We also propose a two-stage place recognition module that synergistically combines both visual and this new lidar descriptor, significantly diminishing cumulative drift. Extensive experimental evaluations on six real-world datasets, including EuRoc, KITTI, NCLT, M2DGR, UrbanNav and UrbanLoco, demonstrate that our MMF-LVINS system achieves superior state estimation accuracy compared to existing state-of-the-art methods. These experiments also validate the effectiveness of our advanced techniques in visual line matching, robust iterative optimization, and enhanced lidar loop closure detection.
更多查看译文
关键词
Lidar-Visual-Inertial SLAM,State Estimation,Robust Iterative Optimization,3D Lidar Loop Closure Descriptor,Two-Stage Loop Detection
AI 理解论文
溯源树
样例
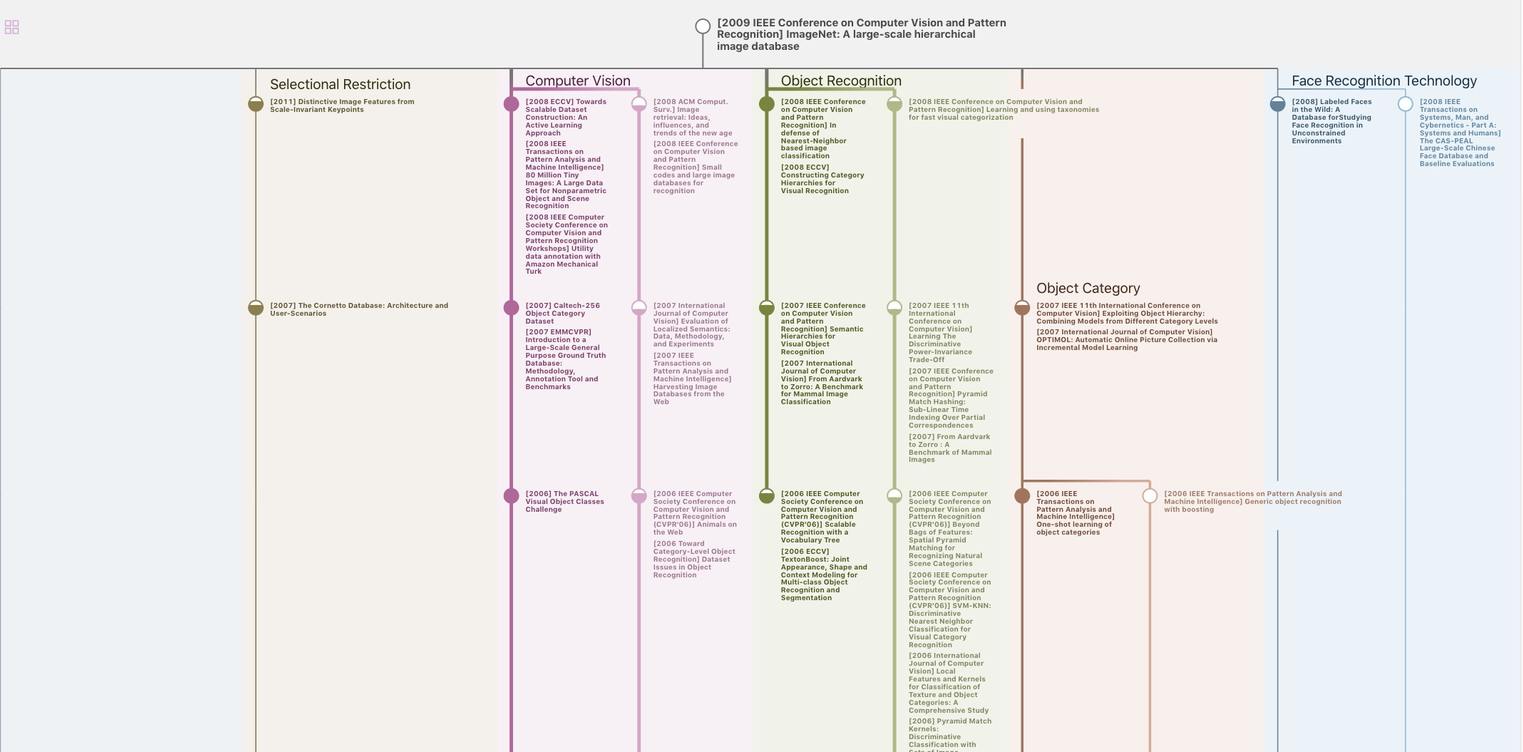
生成溯源树,研究论文发展脉络
Chat Paper
正在生成论文摘要