Reinforcement Learning and Sim-to-Real Transfer of Reorientation and Landing Control for Quadruped Robots on Asteroids
IEEE TRANSACTIONS ON INDUSTRIAL ELECTRONICS(2024)
摘要
In surface exploration missions, wheeled planetary vehicles have difficulty traveling on asteroids due to their weak gravitational fields. With the rapid development of hardware performance and control methods, quadruped robots have great potential to serve in asteroid exploration. When deploying or controlling the jumping motions of a quadruped robot on an asteroid, landing stability must be guaranteed. Under the weak and irregular gravitational fields of asteroids, the robot should be reoriented to an appropriate attitude for a smooth and stable landing. To achieve this objective, a model-free control method based on reinforcement learning (RL) was proposed. Sim-to-real transfer methods including domain randomization and transfer learning were proposed to address the sim-to-real problem. The original control model trained by RL was transferred to a new version that could be applied and tested on a real quadruped robot. An equivalent asteroid's weak gravitational environment experimental platform was for the first time designed and employed to test the performance of the proposed control scheme. Both the simulation and experimental results validated the effectiveness of the proposed controller training and sim-to-real transfer methods.
更多查看译文
关键词
Asteroid exploration,quadruped robots,reinforcement learning (RL),sim-to-real
AI 理解论文
溯源树
样例
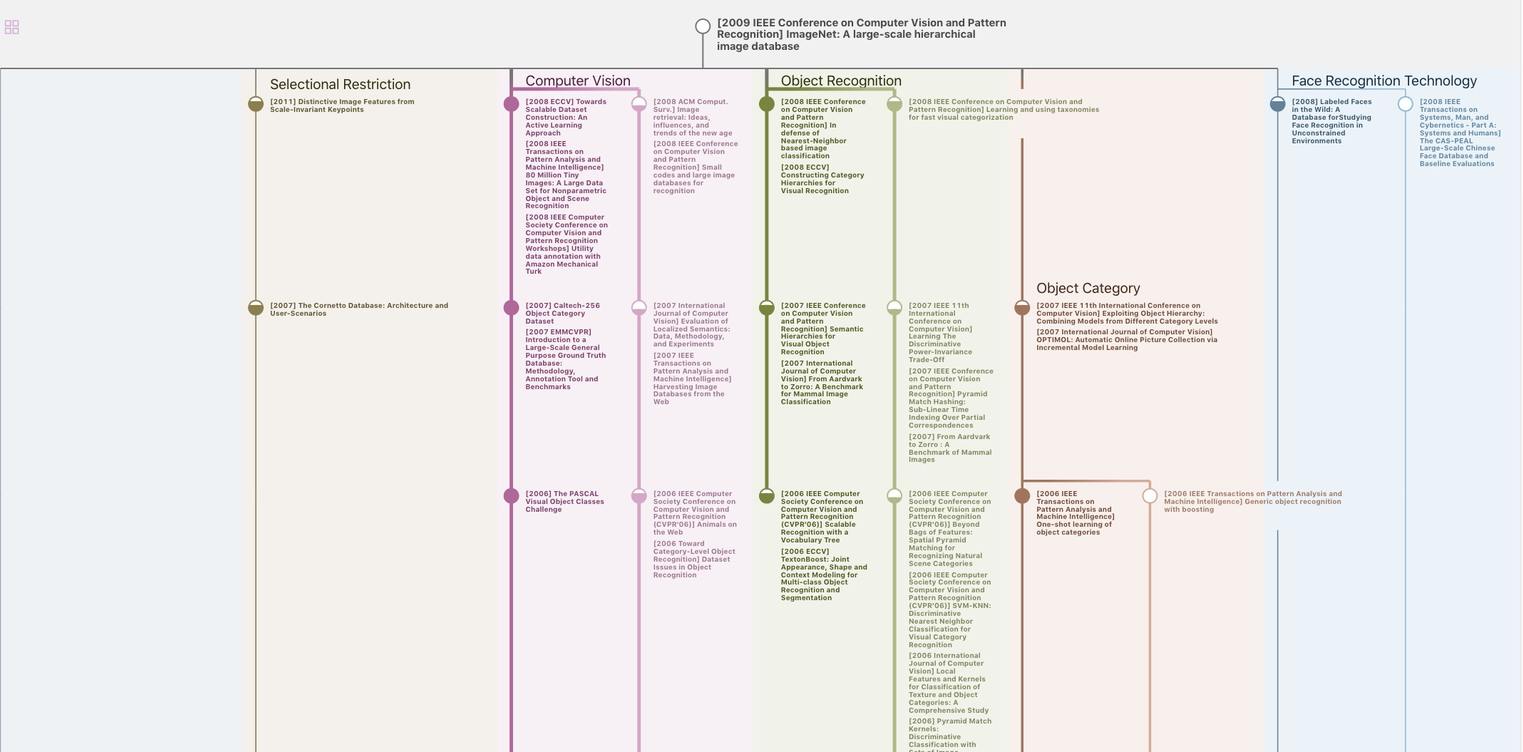
生成溯源树,研究论文发展脉络
Chat Paper
正在生成论文摘要