Hyperspectral Image Classification via Multi-Scale Multi-Angle Attention Network
IEEE Transactions on Geoscience and Remote Sensing(2024)
摘要
Hyperspectral images (HSIs) provide a large amount of spatial and spectral information to characterize ground objects. However, they also contain a lot of redundant information, which makes it difficult to extract complex local and global spatial-spectral features. Considering that HSIs present multi-scale similarity and anisotropic image features, multi-scale and multi-angle information can be used to effectively model local and global features and reduce the complexity of self-attention. This paper proposes a new multi-scale multi-angle attention network (MMAN) for HSI classification that models the internal relationship between image features at local and global scales. Firstly, three spectral-spatial feature extraction modules (at different scales) are constructed to extract the low-level features of the image. These modules are first used by a 3D convolutional layer for spectral feature extraction, and then input to a 2D convolutional layer for spatial feature extraction. Next, the serialized tokens are input to the multi-angle attention module. Finally, the learnable labels are identified through a linear layer, and the features of different scales are fused through a fully connected layer to realize the classification of samples. Experimental results on four standard datasets show that the proposed exhibits comparable or superior classification performance than other state-of-the-art methods.
更多查看译文
关键词
Hyperspectral images (HSIs),cross-scale similarity,anisotropy,multi-scale,multi-angle attention,transformer
AI 理解论文
溯源树
样例
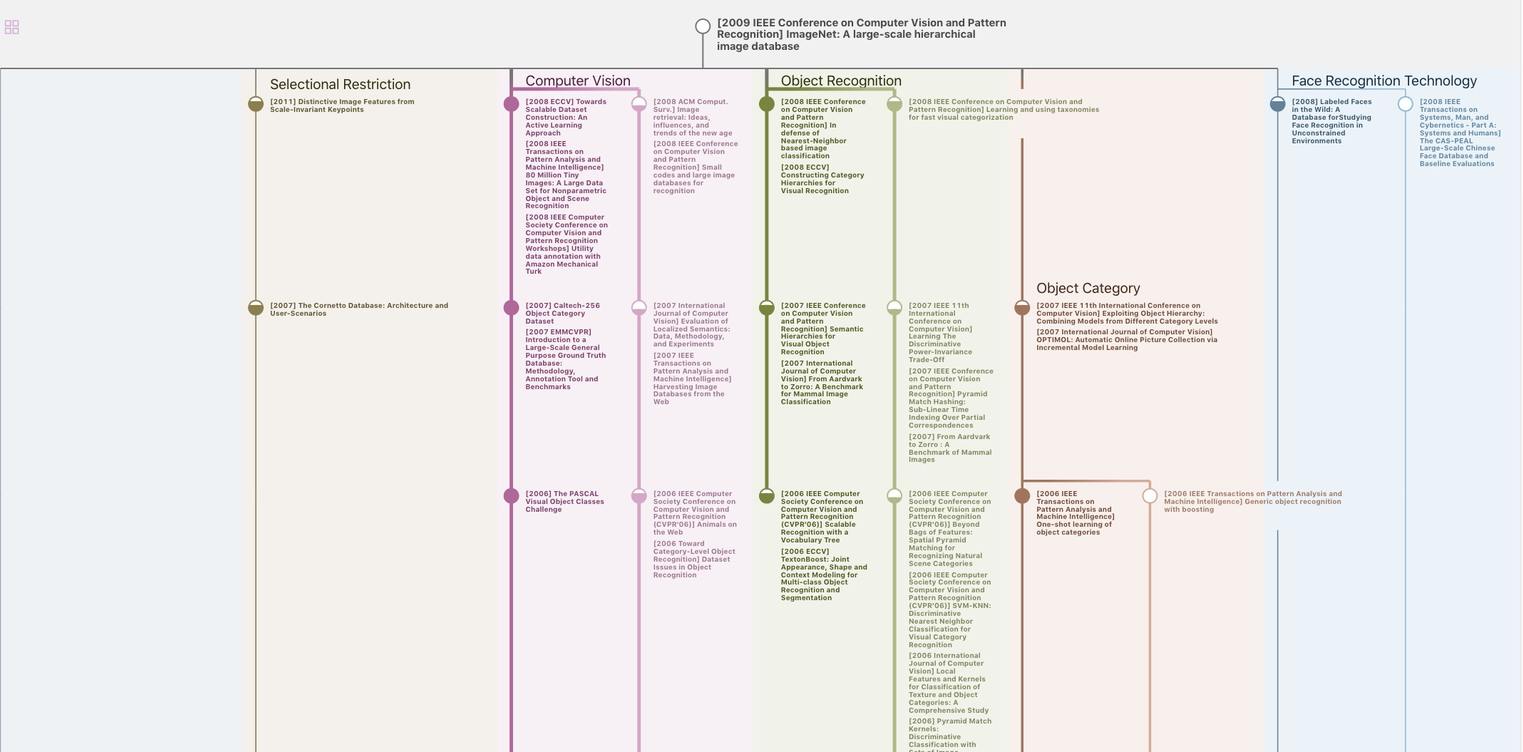
生成溯源树,研究论文发展脉络
Chat Paper
正在生成论文摘要