UIE-Convformer: Underwater Image Enhancement Based on Convolution and Feature Fusion Transformer
IEEE TRANSACTIONS ON EMERGING TOPICS IN COMPUTATIONAL INTELLIGENCE(2024)
摘要
Due to the light scattering and absorption of impurities, the quality of underwater imaging is poor, which seriously affects underwater exploration and research. To address the problem, a novel underwater image enhancement method integrating the convolutional neural network (CNN) with a feature fusion Transformer (UIE-Convformer) is proposed. Specifically, the proposed UIE-Convformer adopts a multi-scale U-Net structure to fully mine rich texture information and semantic information. Firstly, considering that CNN is more efficient and comprehensive in extracting local feature information of underwater images, the ConvBlock module based on CNN is proposed to extract local features of images and ensure the efficiency and integrity of feature extraction. Furthermore, considering the serious color deviation caused by the absorption and scattering of light in water, as well as the large-scale blur and diffusion effects in the underwater environment, the feature fusion transformer module (Feaformer) for global information fusion and reconstruction is proposed to establish long-distance feature dependency. Additionally, the Jump Fusion Connection Module (JFCM) is built between the encoder and the decoder to fuse multi-scale features through effective bidirectional cross-connection and weighted fusion, which helps to provide richer feature information for the reconstruction of the decoder. Finally, the refinement module is designed to further optimize the details of the underwater images and achieve better visual effects. Experimental results on available datasets show the effectiveness of the proposed UIE-Convformer.
更多查看译文
关键词
Convolutional neural network,feature fusion transformer,underwater image enhancement
AI 理解论文
溯源树
样例
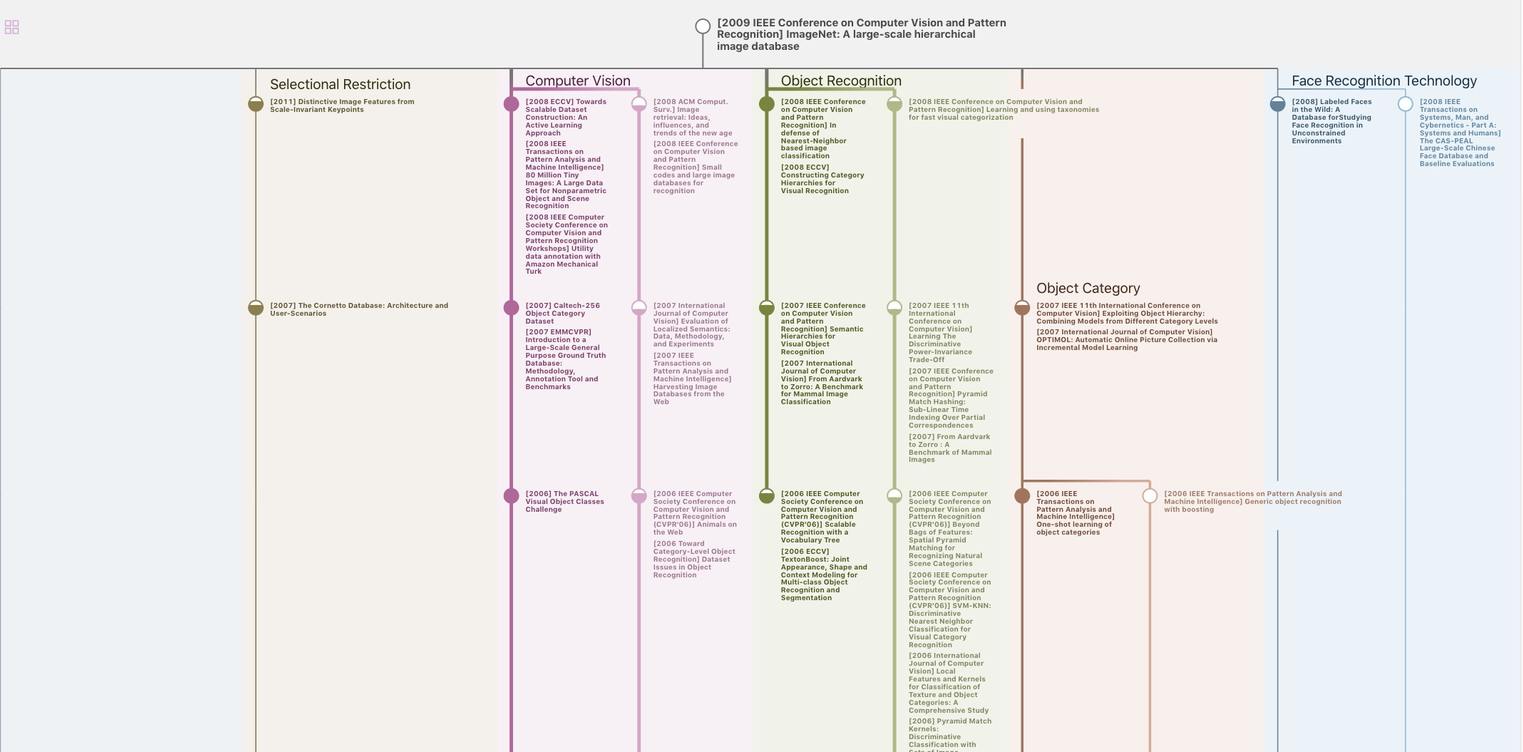
生成溯源树,研究论文发展脉络
Chat Paper
正在生成论文摘要