A Surrogate-Assisted Evolutionary Framework for Expensive Multitask Optimization Problems
IEEE Transactions on Evolutionary Computation(2024)
摘要
This paper proposes a surrogate-assisted evolutionary framework (called SELF) to solve expensive multitask optimization problems (ExMTOPs). SELF consists of two main phases: global knowledge transfer phase and local knowledge transfer phase. In the former, a multitask Gaussian process model (MTGP) is established by fusing previously evaluated solutions of multiple optimization tasks. MTGP can capture task-relevant information and the knowledge of landscapes. Then, differential evolution assisted with MTGP is proposed to preselect high-quality candidates. During the preselection, the knowledge of landscapes is transferred among multiple optimization tasks for locating promising regions quickly. In the latter, for each optimization task, Bayesian optimization is adopted to improve the quality of the best individual in the population. Moreover, the improved best individuals in the populations of multiple optimization tasks are adaptively transferred based on a transfer probability, which is computed through the task-relevant information provided by MTGP. By combining these two phases, SELF not only achieves the tradeoff between exploration and exploitation, but also utilizes the global and local knowledge transfer to improve the efficiency for solving ExMTOPs. We test SELF on seven benchmark test problems in the IEEE CEC2017 evolutionary multitask optimization competition. The results demonstrate that the performance of SELF is better than that of other seven advanced methods. In addition, we also apply SELF to deal with two real-world ExMTOPs. The designs provided by SELF exhibit the best performance among all the compared methods, verifying the potential of SELF in practical engineering applications.
更多查看译文
关键词
Expensive multitask optimization problems,evolutionary multitask optimization,knowledge transfer,surrogate model,Bayesian optimization
AI 理解论文
溯源树
样例
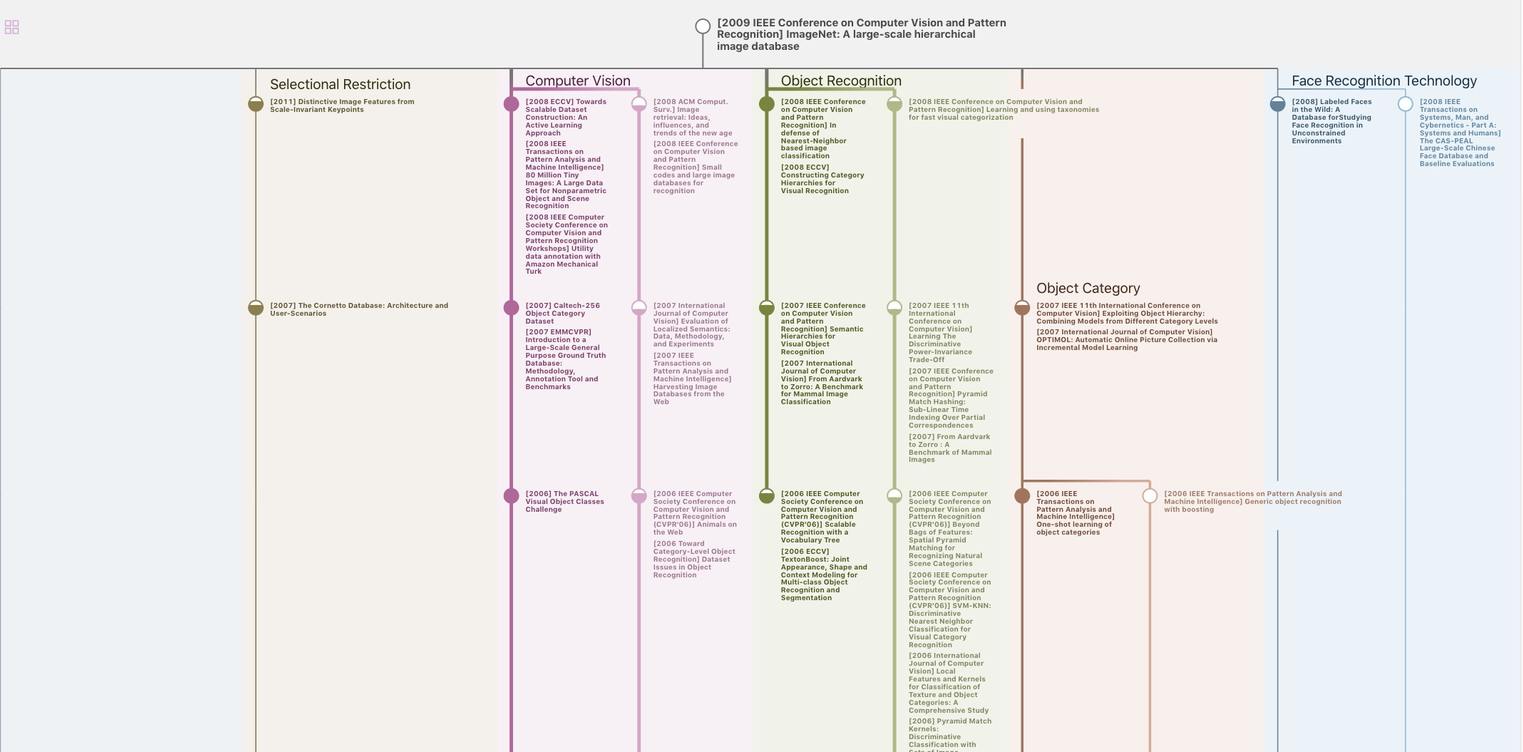
生成溯源树,研究论文发展脉络
Chat Paper
正在生成论文摘要