Estimating Black Carbon Levels With Proxy Variables and Low-Cost Sensors
IEEE Internet of Things Journal(2024)
摘要
We develop a portable and affordable solution for estimating personal exposure to black carbon (BC) using low-cost sensors and machine learning. Our approach uses other pollutants and environmental variables as proxies for estimating the concentrations of BC and combines this with machine learning based sensor calibration to improve the quality of the inputs that are used as proxies in the modeling. We extensively validate the feasibility of our approach and demonstrate its benefits with benchmarks conducted on real world data from two different urban locations with different population densities and characteristics. Our results demonstrate that our approach can accurately estimate BC (R2 higher than 0.9) without relying on a dedicated sensor. The results also highlight how calibration is essential for ensuring accurate modeling on low-cost sensor measurements. Our results offer a novel affordable and portable solution that can be used to estimate personal exposure to BC and, more generally, demonstrate how low-cost sensors and proxy modeling can increase the spatiotemporal scale at which information about BC level is available.
更多查看译文
关键词
black carbon,air quality,low-cost sensor,proxy,machine learning
AI 理解论文
溯源树
样例
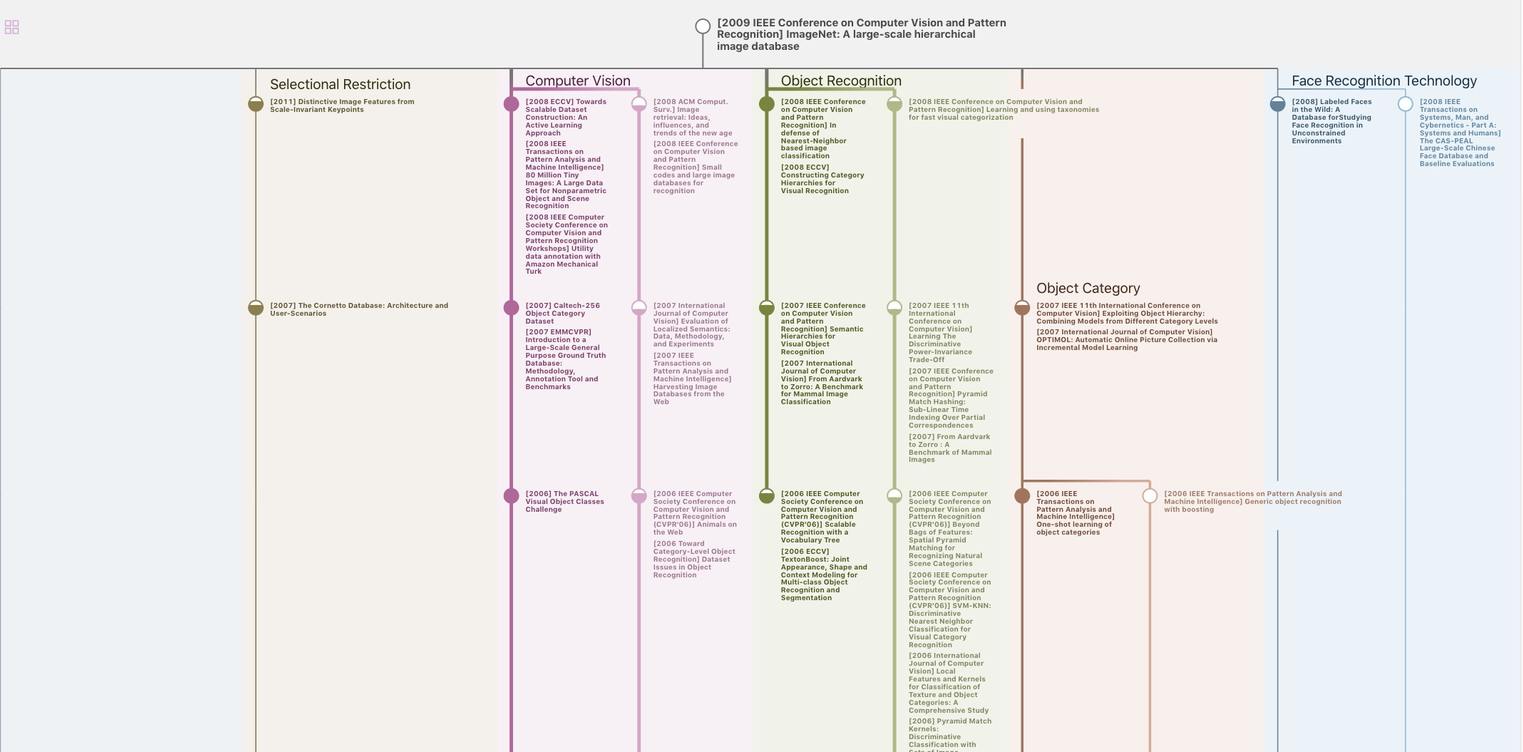
生成溯源树,研究论文发展脉络
Chat Paper
正在生成论文摘要