A Detection Method Against Selfish Mining-Like Attacks Based On Ensemble Deep Learning in IoT
IEEE Internet of Things Journal(2024)
摘要
Cryptojacking is a new type of IoT (Internet of Things) attack, where an attacker hijacks the computing power of IoT devices such as wireless routers, smart TVs, set-top boxes, or cameras to mine cryptocurrencies, e.g., PyRoMineIoT. The attackers launch selfish mining-like (SM-like) attacks to obtain lucrative mining rewards with the stolen computing power, once the power exceeds a threshold. Generally, a single deep learning (DL) model with a single feature (e.g. fork height) is trained to detect SM-like attacks. However, the existing model fails to detect every SM-like attack since the model training ignores other distinctive features (e.g. mining rewards and blocking rate) of SM-like attacks. In this paper, SM-NEEDLE, an eNsEmblE Deep LEarning (NEEDLE) method is proposed to detect SM-like attacks. More specifically, the distinctive features are extracted from the blockchain system, where SM-like simulators emulate the strategies of SM-like attacks. Further, to circumvent the local optima problem caused by the single DL model (e.g. Back-Propagation Neural Network, BPNN), the SM-NEEDLE trains multiple BPNNs with these distinctive features. Evaluation results indicate the accuracy and false negative rate (FNR) of SM-NEEDLE for detecting SM-like attacks (including SM1 and its variants) are 98.9% and 1.48% respectively. That is, 98.9% of SM-like attacks are correctly identified and only 1.48% of attacks are undetectable.
更多查看译文
关键词
Blockchain,ensemble deep learning,selfish mining attack,back-propagation neural network
AI 理解论文
溯源树
样例
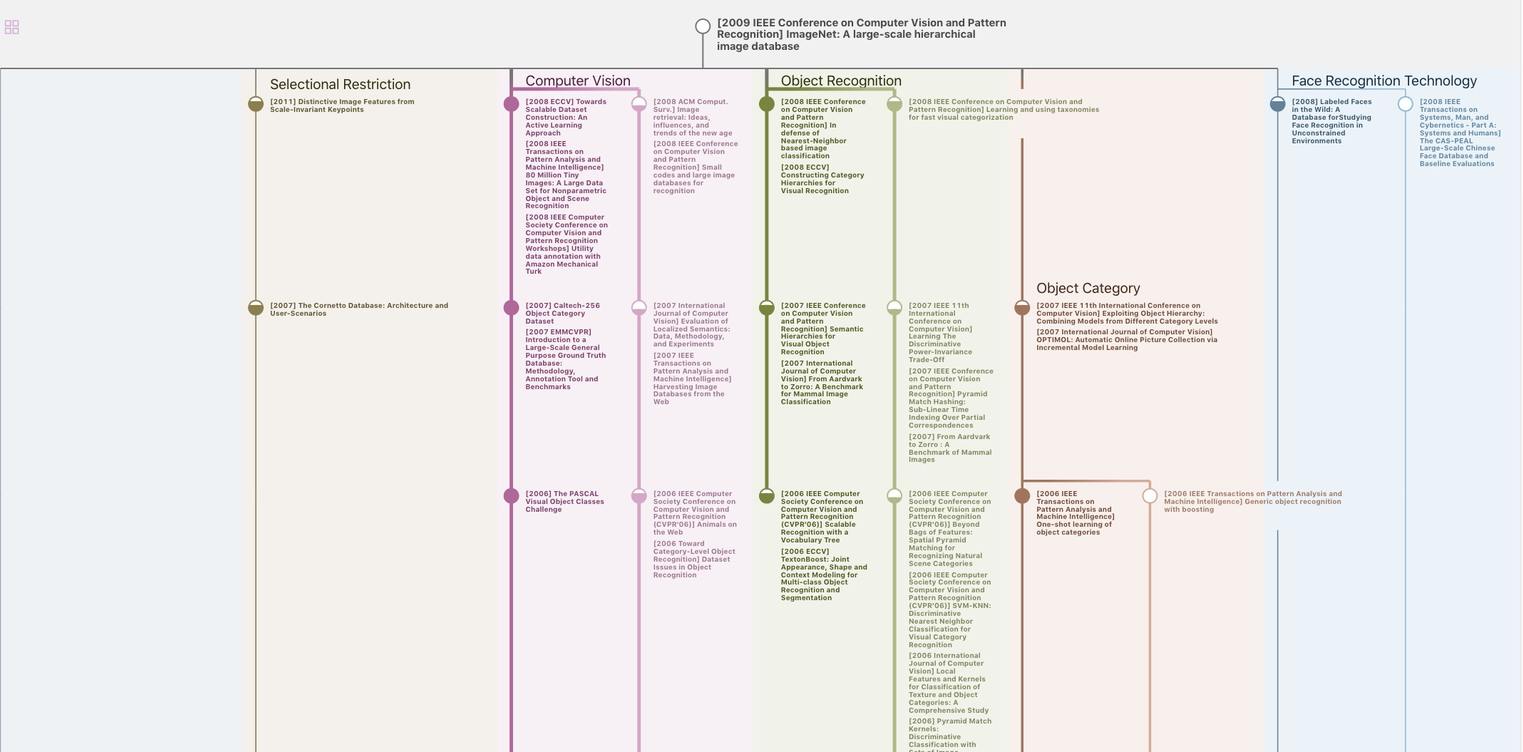
生成溯源树,研究论文发展脉络
Chat Paper
正在生成论文摘要