Lightweight Federated Graph Learning for Accelerating Classification Inference in UAV-assisted MEC Systems
IEEE Internet of Things Journal(2024)
摘要
With flexible mobility and broad communication coverage, Unmanned Aerial Vehicles (UAVs) have become an important extension of Multi-access Edge Computing (MEC) systems, exhibiting great potential for improving the performance of Federated Graph Learning (FGL). However, due to the limited computing and storage resources of UAVs, they may not well handle the redundant data and complex models, causing the inference inefficiency of FGL in UAV-assisted MEC systems. To address this critical challenge, we propose a novel LightWeight FGL framework, named LW-FGL, to accelerate the inference speed of classification models in UAV-assisted MEC systems. Specifically, we first design an adaptive Information Bottleneck (IB) principle, which enables UAVs to obtain well-compressed worthy subgraphs by filtering out the information that is irrelevant to downstream classification tasks. Next, we develop improved tiny Graph Neural Networks (GNNs), which are used as the inference models on UAVs, thus reducing the computational complexity and redundancy. Using real-world graph datasets, extensive experiments are conducted to validate the effectiveness of the proposed LW-FGL. The results show that the LW-FGL achieves higher classification accuracy and faster inference speed than state-of-the-art methods.
更多查看译文
关键词
Multi-access Edge Computing,Unmanned Aerial Vehicle,Federated Graph Learning,classification inference,lightweight model
AI 理解论文
溯源树
样例
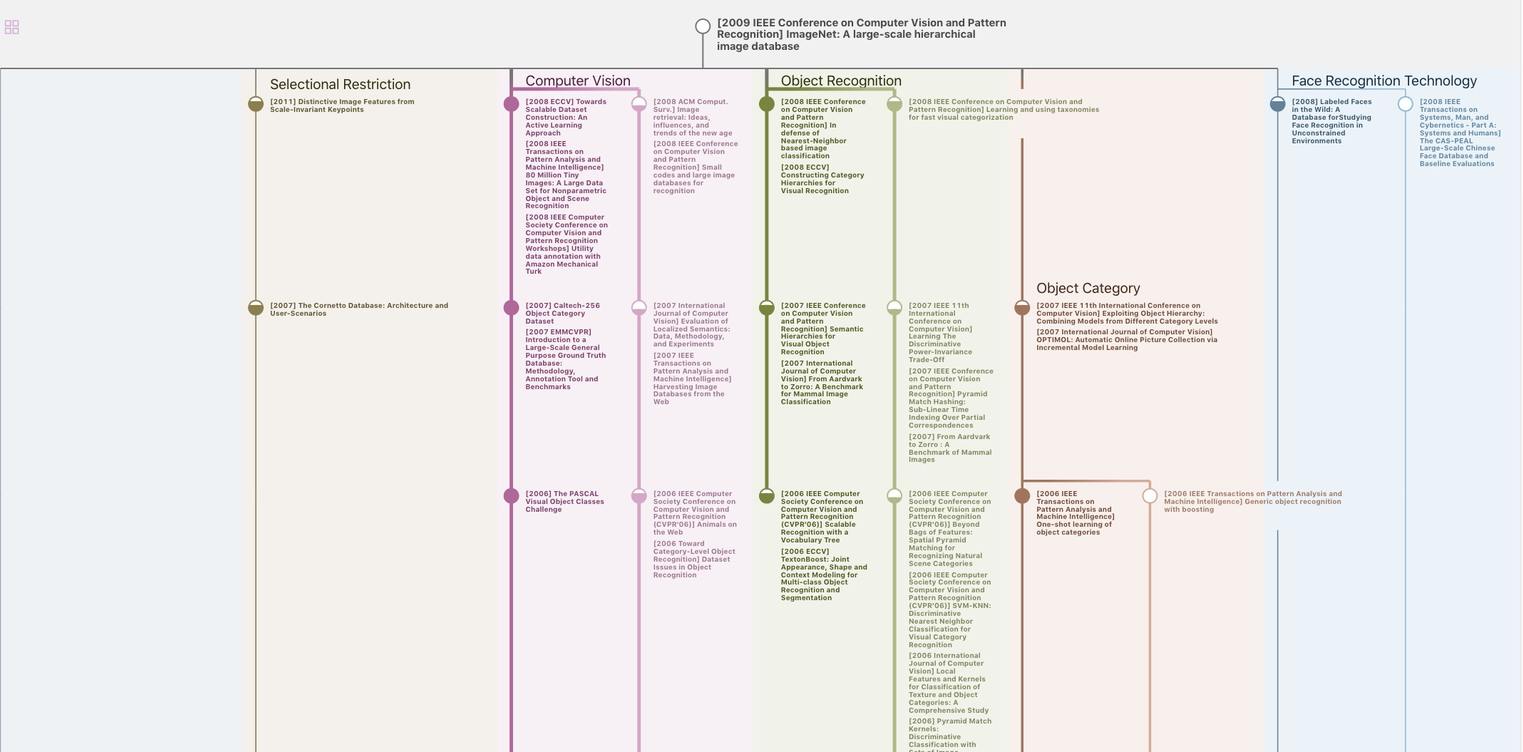
生成溯源树,研究论文发展脉络
Chat Paper
正在生成论文摘要