Reducing High-Frequency Artifacts for Generative Model Watermarking via Wavelet Transform
IEEE Internet of Things Journal(2024)
摘要
As generative models find broader applications in Internet of Things (IoT) image processing tasks, safeguarding the copyright of these models assumes increasing significance. Embedding watermarks on the output images generated by such models has been proposed by some researchers as a means of protecting intellectual property. However, prevailing methods for generating model watermarks inadvertently introduce significant high-frequency artifacts in high-frequency regions, compromising the imperceptibility and security of the watermarking system. In pursuit of enhancing the imperceptibility of generative model watermarking, we propose a framework based on discrete wavelet transform. This framework effectively mitigates the high-frequency artifact issue and enhances the frequency-domain concealment of watermarking. Specifically, we introduce an embedded watermarking network, a frequency separation layer, and a watermark extraction network after the output of the target model. We construct a wavelet frequency domain separation layer by wavelet decomposition to decompose the image generated by the embedding network into different frequency components, and embed the watermark into the low-frequency region of the target model output image through joint training and joint loss optimization of the embedding and extraction networks. Extensive experiments conducted on two image processing tasks, i.e., painting transfer and de-raining, demonstrate that our method exhibits no discernible traces of high-frequency artifacts in the frequency domain of the image in both cases, thus boasting superior invisibility. Furthermore, our method demonstrates robustness against pre-processing attacks, such as noise addition, resizing, and image cropping.
更多查看译文
关键词
Model watermarking,security,wavelet transform,high-frequency artifacts,imperceptibility
AI 理解论文
溯源树
样例
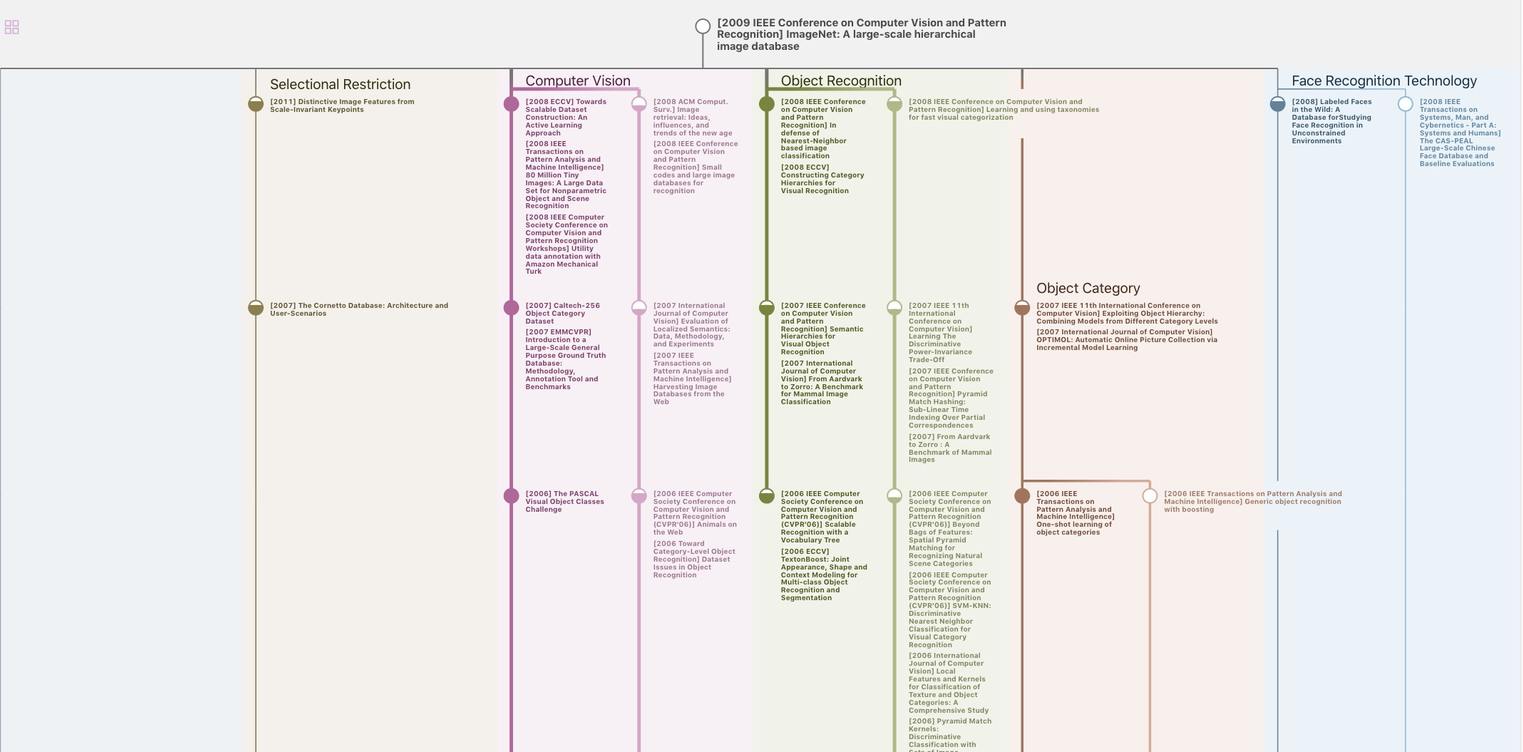
生成溯源树,研究论文发展脉络
Chat Paper
正在生成论文摘要