SVCA: Secure and Verifiable Chained Aggregation for Privacy-Preserving Federated Learning
IEEE Internet of Things Journal(2024)
摘要
Federated learning (FL), as a distributed machine learning paradigm, enables multiple users to train machine learning models locally using individual data and then update global model in a privacy-preserving aggregated manner. However, in FL, the users model parameters are at risk of a privacy breach. Furthermore, the aggregation server may forge aggregated results. To address these problems, in this paper, we propose SVCA, a secure and verifiable chained aggregation for privacy-preserving federated learning (PPFL) scheme. Specifically, we first group users and construct a chained aggregation structure, then employ secret sharing to prevent the entire group of users dropout, and finally propose a scheme for secure verification of the aggregation result to ensure the result correctness and the security of the verification process. The security analysis shows that SVCA not only protects the privacy of users but also ensures the training integrity. Extensive experimental results demonstrate the practical performance of SVCA without compromising classification accuracy.
更多查看译文
关键词
Federated learning,privacy-preserving,chained aggregation,secure verification
AI 理解论文
溯源树
样例
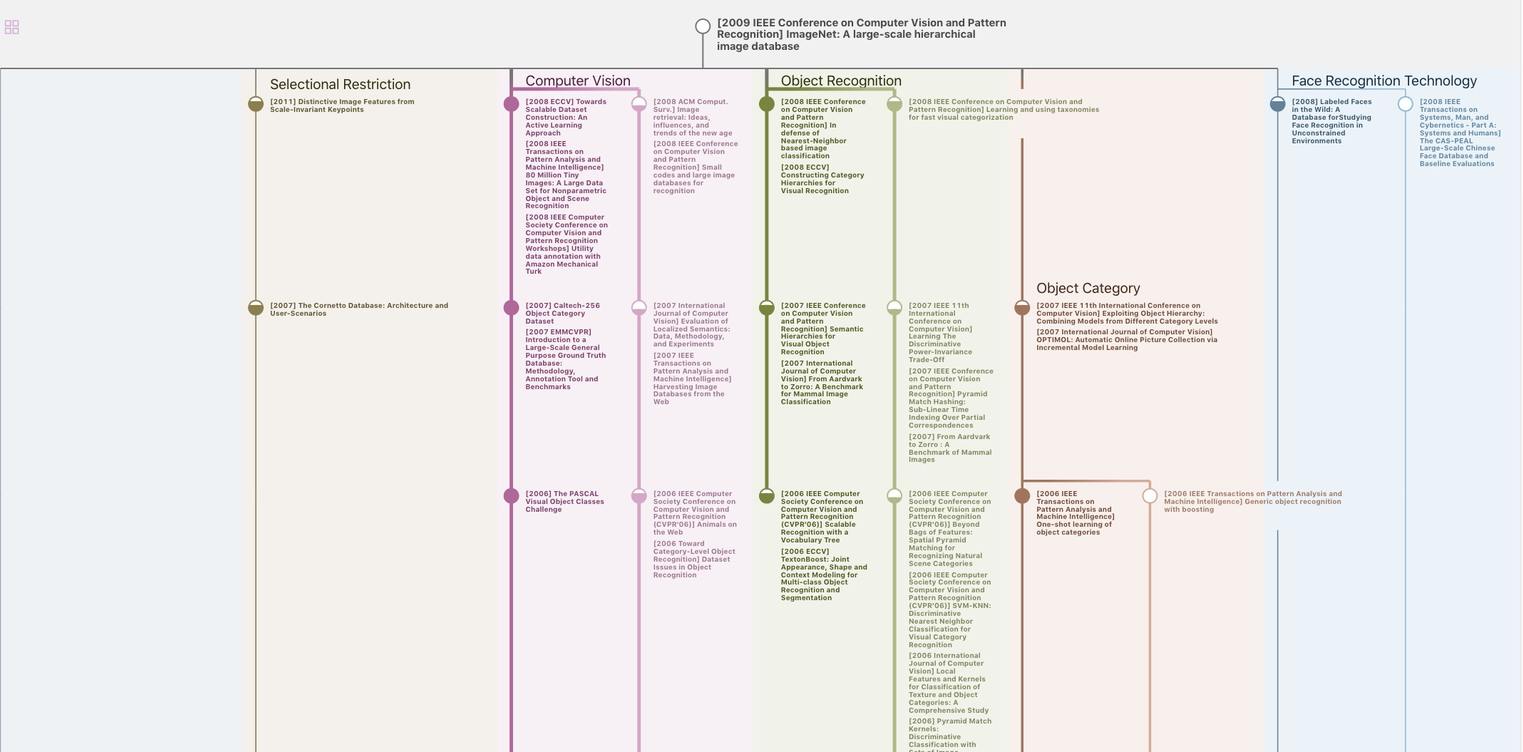
生成溯源树,研究论文发展脉络
Chat Paper
正在生成论文摘要