Long-Term Throughput Maximization in Wireless Powered Communication Networks: A Multi-Task DRL Approach
IEEE Internet of Things Journal(2024)
摘要
In this paper, we study a wirelessly-powered communication network (WPCN) composed of several wireless devices (WDs) which rely on an energy access point (EAP) for their supply of energy which is used to transfer data to a data access point (DAP). While the dominant focus of the literature in this area has been on frame-based data rate optimization, it has been known that this approach is greedy and only optimal in additive white Gaussian noise (AWGN) channels. Hence, in this work, we propose an online algorithm that, based on the current state of the batteries and channels, adaptively calculates the transmission time and power allocations to maximize the long-term performance of this system in fading channels. In order to accomplish such a goal, we employ the Twin Delayed DDPG (TD3) approach, which is a deep reinforcement learning (DRL) technique designed for continuous state and action spaces. Additionally, we use the multi-task learning (MTL) techniques to train a DRL agent that creates a composite policy capable of dynamically optimizing not just one specific WPCN configuration, but a continuum of WPCN problems, which we call a composite environment. Each of these environments has different parameters such as WDs’ distances to the DAP and EAP, batteries’ size, rectifying antenna (rectenna) energy harvesting (EH) model linearity, as well as different uplink (UL) and downlink (DL) channel specular components. Simulation results confirm that the TD3 algorithm can easily learn to achieve a much higher common throughput compared to the traditional optimization schemes.
更多查看译文
关键词
Deep reinforcement learning,wireless power transfer (WPT),energy harvesting (EH),transmission scheduling,ergodic throughput,deep deterministic policy gradient (DDPG)
AI 理解论文
溯源树
样例
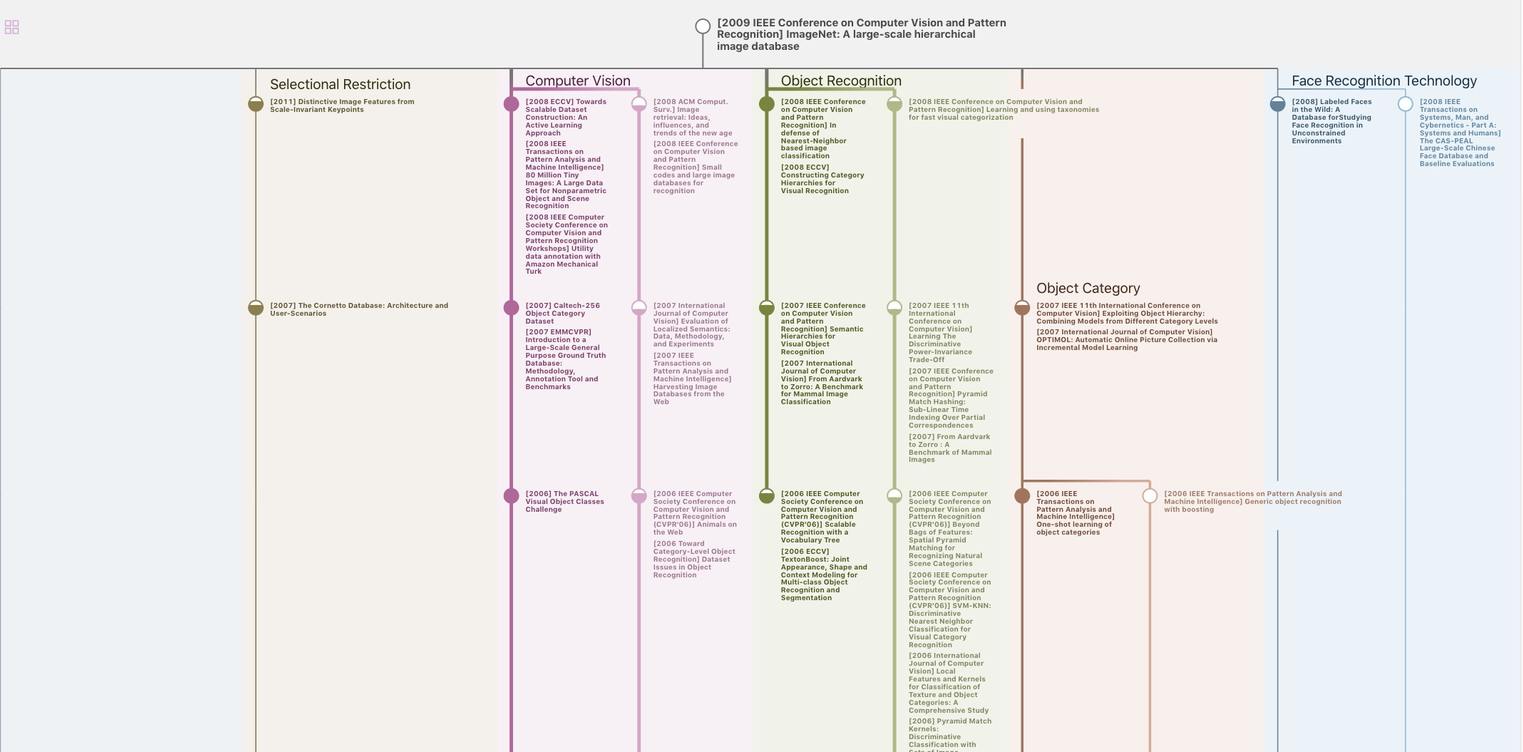
生成溯源树,研究论文发展脉络
Chat Paper
正在生成论文摘要