A Privacy-Preserving Federated Learning Scheme Against Poisoning Attacks in Smart Grid
IEEE Internet of Things Journal(2024)
摘要
Privacy preservation in federated learning (FL) has received considerable attention and many approaches have been proposed. However, these approaches rendered the uploaded gradients invisible to the server, which poses a significant challenge in defending against poisoning attacks. In poisoning attacks, malicious or compromised participants use poisoned training data or forged local updates to disrupt the training process. It is hard for cloud servers to defend against poisoning attacks due to the invisibility of gradients. To address this issue, we propose a privacy-preserving federated learning scheme (PFLS) against poisoning attacks to eliminate the impact of model poisoning attacks while protecting the privacy of participants. Specifically, a dynamic adaptive defense mechanism is designed to mitigate the impact of malicious gradients and locate malicious participants. To protect participants’ privacy, a multi-dimensional homomorphic encryption method is constructed with a hierarchical aggregation architecture. The security analysis illustrates that the PFLS scheme can ensure the privacy of FL participants. The experimental results demonstrate that a high detection rate of malicious participants and a balance between efficiency and robustness are achieved.
更多查看译文
关键词
Federated Learning,Privacy Protection,Model Poisoning Attack,Signature Authentication
AI 理解论文
溯源树
样例
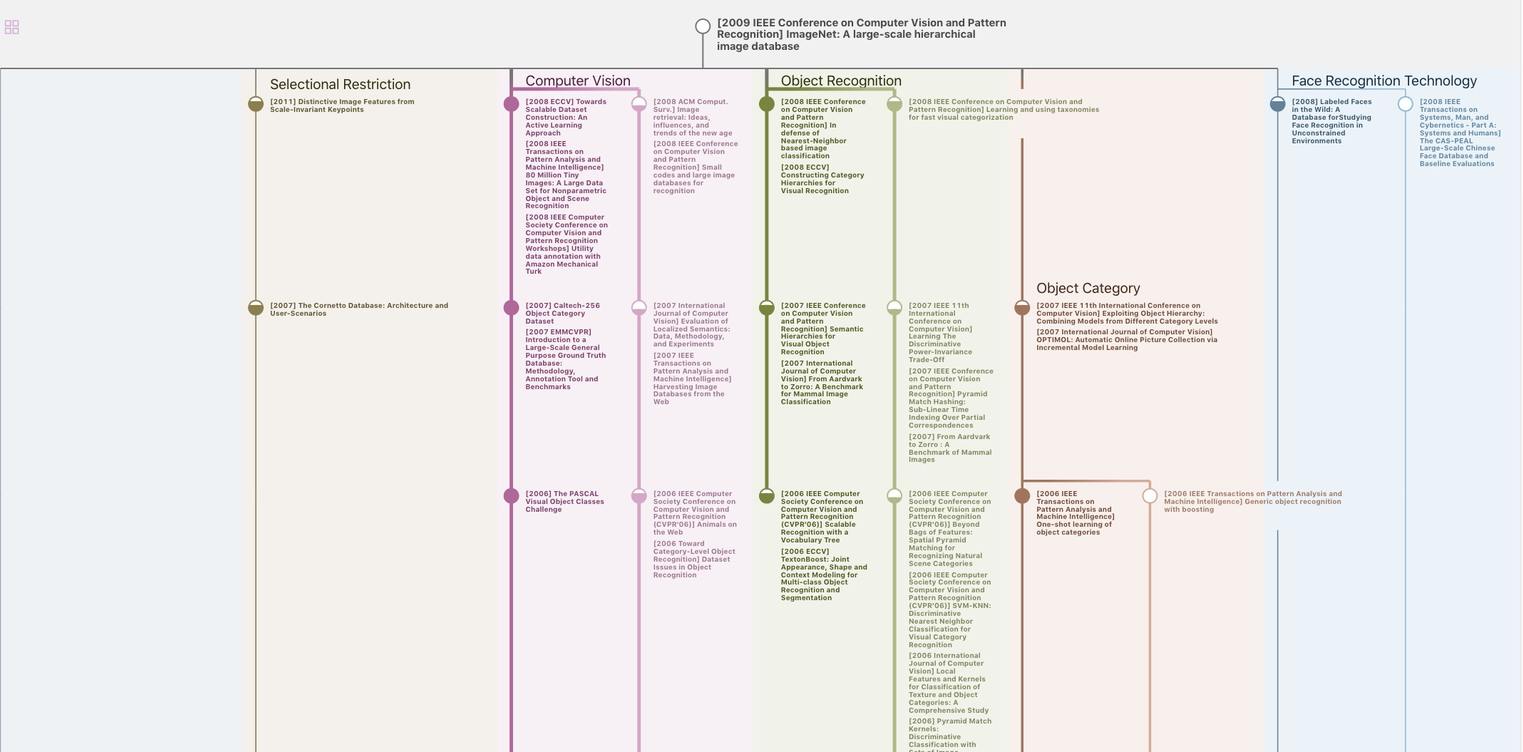
生成溯源树,研究论文发展脉络
Chat Paper
正在生成论文摘要