Multi-Task Data Collection With Limited Budget in Edge-Assisted Mobile Crowdsensing
IEEE Internet of Things Journal(2024)
摘要
Due to the swift advancement of edge computing and mobile crowdsensing (MCS), edge-assisted mobile crowdsensing (EAMCS) has emerged as a promising paradigm, leveraging sensor-embedded mobile devices for the collection and sharing of environmental data. As the sensing scale increases in the modern urban, the application scenario becomes more and more complex, and the budget of users and platform is limited. Therefore, it is indispensable to study the effective task allocation mechanism with considering the multiple budget constraints in the EAMCS system. However, a majority of the existing studies unilaterally focus on either the users’ time budget or the platform’s budget, disregarding the crucial aspect of the users’ energy budget. In this paper, we design a joint user movement, sensing, offloading and computation framework adopting the computation offloading strategy called binary processing strategy. In addition, the multi-task data collection with a limited budget (MDCB) problem considering time, energy and platform budget in EAMCS is formulated, which is proved to be non-deterministic polynomial-hard. In order to maximize the amount of data collected by the users in the MDCB problem, we first verify the submodularity of the objective function, then propose the global maximum data first search algorithm and task sequence-based genetic algorithm to solve the problem. The extensive experiments are conducted on both synthetic and real-world datasets to demonstrate the effectiveness of our proposed schemes.
更多查看译文
关键词
Edge-assisted mobile crowdsensing,data collection,time budget,energy budget,multi-task allocation
AI 理解论文
溯源树
样例
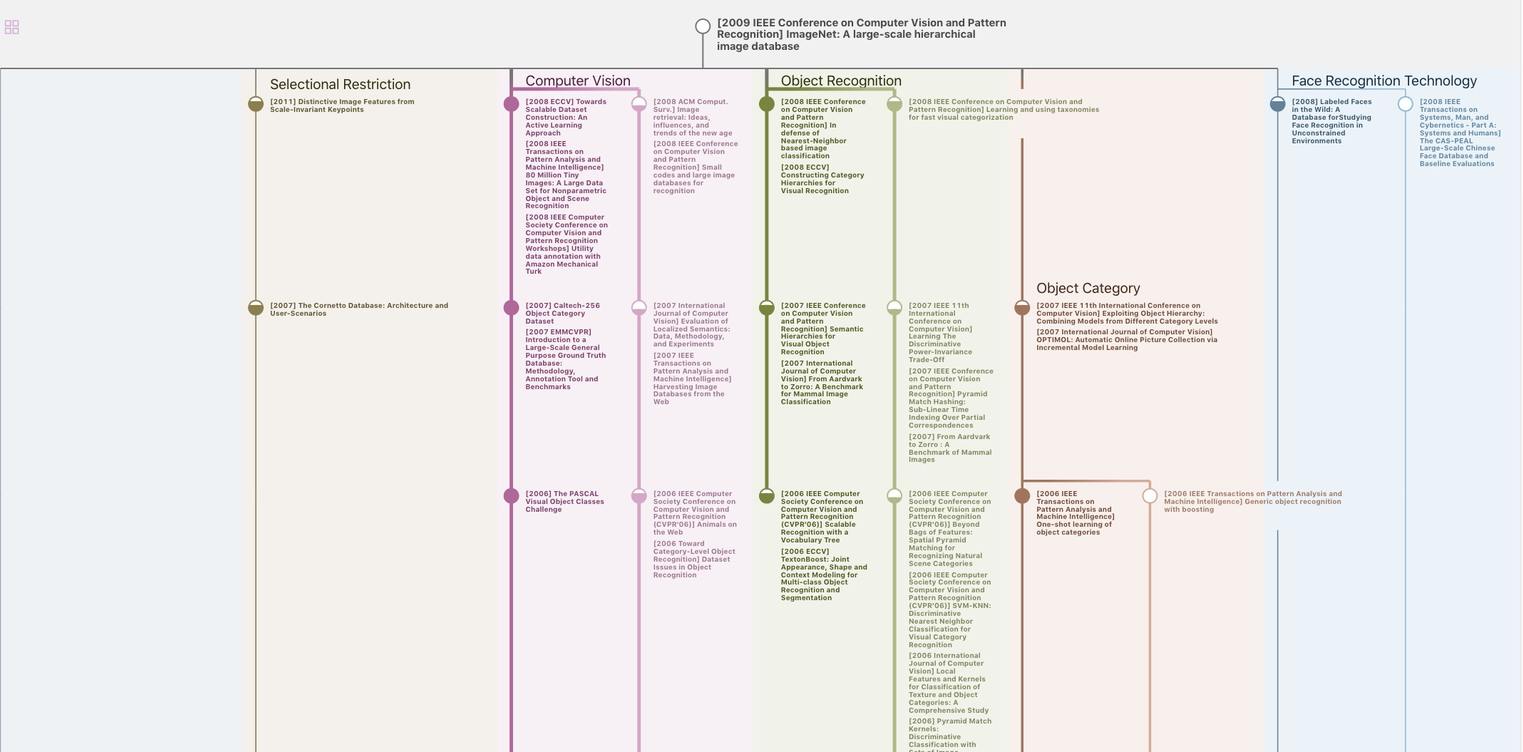
生成溯源树,研究论文发展脉络
Chat Paper
正在生成论文摘要