Lightweight Machine Learning-based Diagnosis for Power Electronic Systems Subject to Imbalanced Data
IEEE Journal of Emerging and Selected Topics in Industrial Electronics(2024)
摘要
Machine learning-based fault diagnosis methods have gained extensive applications in power electronic systems. One of the key challenges for field implementation is the rarity of fault history data in practical systems. It leads to an imbalanced ratio between normal and fault state data, causing high accuracy for the majority but severely reducing that of the minority class. To address this issue, a method integrating of oversampling and ensemble learning is proposed in this paper. First, the feature extraction and hybrid selection schemes are designed to obtain the most critical features, thereby enhancing the performance of the sampling process. The safe-level synthetic minority oversampling technique (safe-level SMOTE) is then applied to sample the minority classes to balance the historic database. An ensemble random vector functional link network (RVFL) learning model, which is computationally light, is developed as the diagnostic model to further improve the accuracy. The method is demonstrated by an experimental study on the sensor and open-circuit faults of single-phase rectifiers.
更多查看译文
关键词
Fault diagnosis,imbalanced data,hybrid feature selection scheme,safe-level SMOTE,ensemble learning
AI 理解论文
溯源树
样例
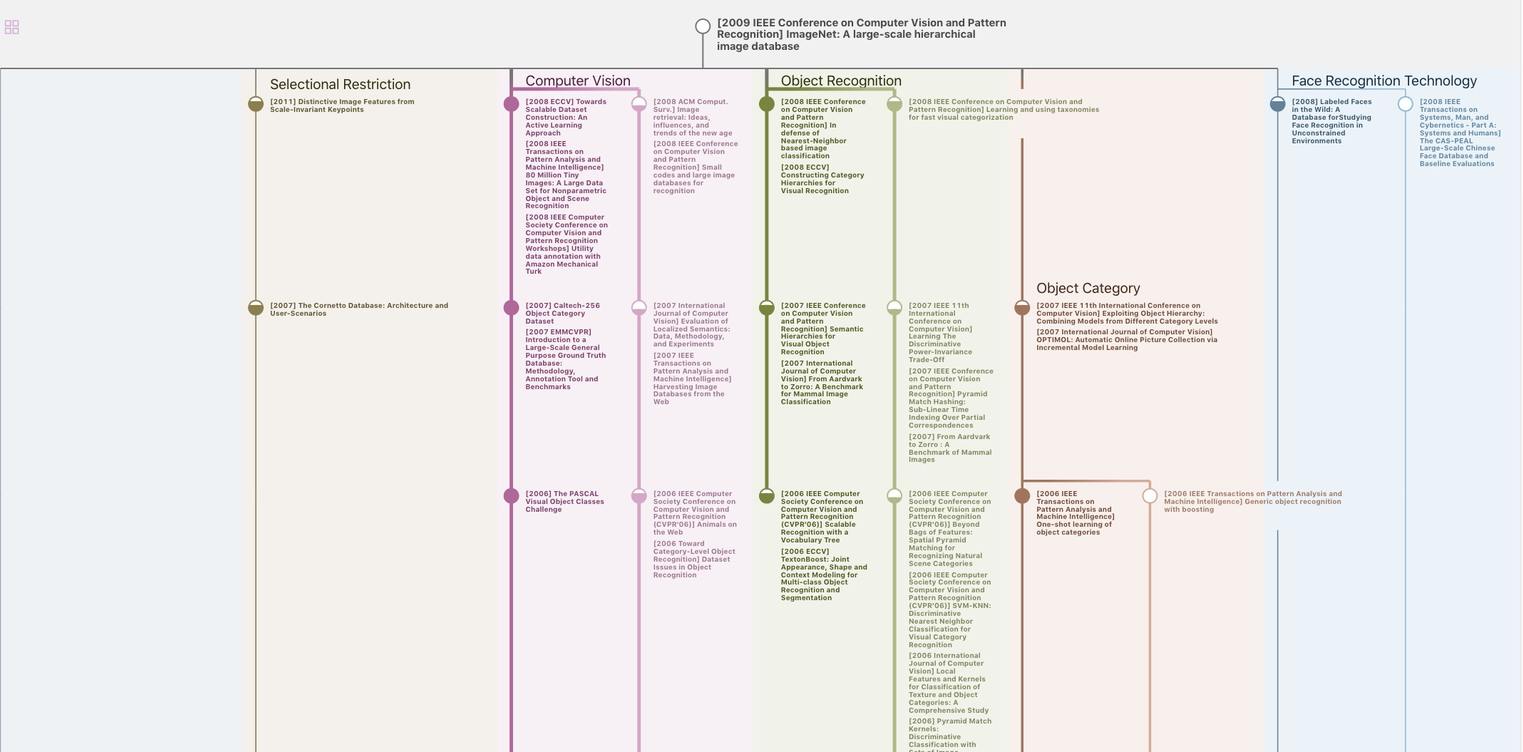
生成溯源树,研究论文发展脉络
Chat Paper
正在生成论文摘要