Data Poisoning Attacks and Defenses to LDP-based Privacy-Preserving Crowdsensing
IEEE Transactions on Dependable and Secure Computing(2024)
摘要
In this paper, we explore data poisoning attacks and their defenses in local differential privacy (LDP)-based crowdsensing systems. First, we construct data poisoning attacks launched by corrupted workers to subvert crowdsensing results by tampering information reported. Specifically, the attacks are formulated as a bi-level optimization problem where attackers strive to conceal their malicious behavior by delicately exploiting noise perturbation introduced by LDP protocols. In this way, the attacks can not be detected, even with the weight-based truth discovery methods. Due to the NP-hard nature of the bi-level problem, we decompose it into upper-level and lower-level sub-problems and employ the augmented Lagrangian method to iteratively solve them, ultimately identifying optimal attack strategies. Second, we propose corresponding countermeasures to defend against the attacks. The countermeasures are formulated as a minimization problem, with the objective of minimizing disruptions caused by attacks through the identification and removal of corrupted workers from crowdsensing systems. To solve the problem, we utilize a differential evolution algorithm instead of gradient-based methods since the objective function of the problem is not differentiable. Extensive experiments on real-world datasets are conducted to evaluate the performance of the proposed attacks and defenses. The evaluation results demonstrate that LDP perturbation indeed facilitates the success of data poisoning attacks, and the proposed defenses can accurately distinguish malicious behaviors disguised.
更多查看译文
关键词
Data poisoning attacks,local differential privacy,crowdsensing,truth discovery,optimization-based defenses
AI 理解论文
溯源树
样例
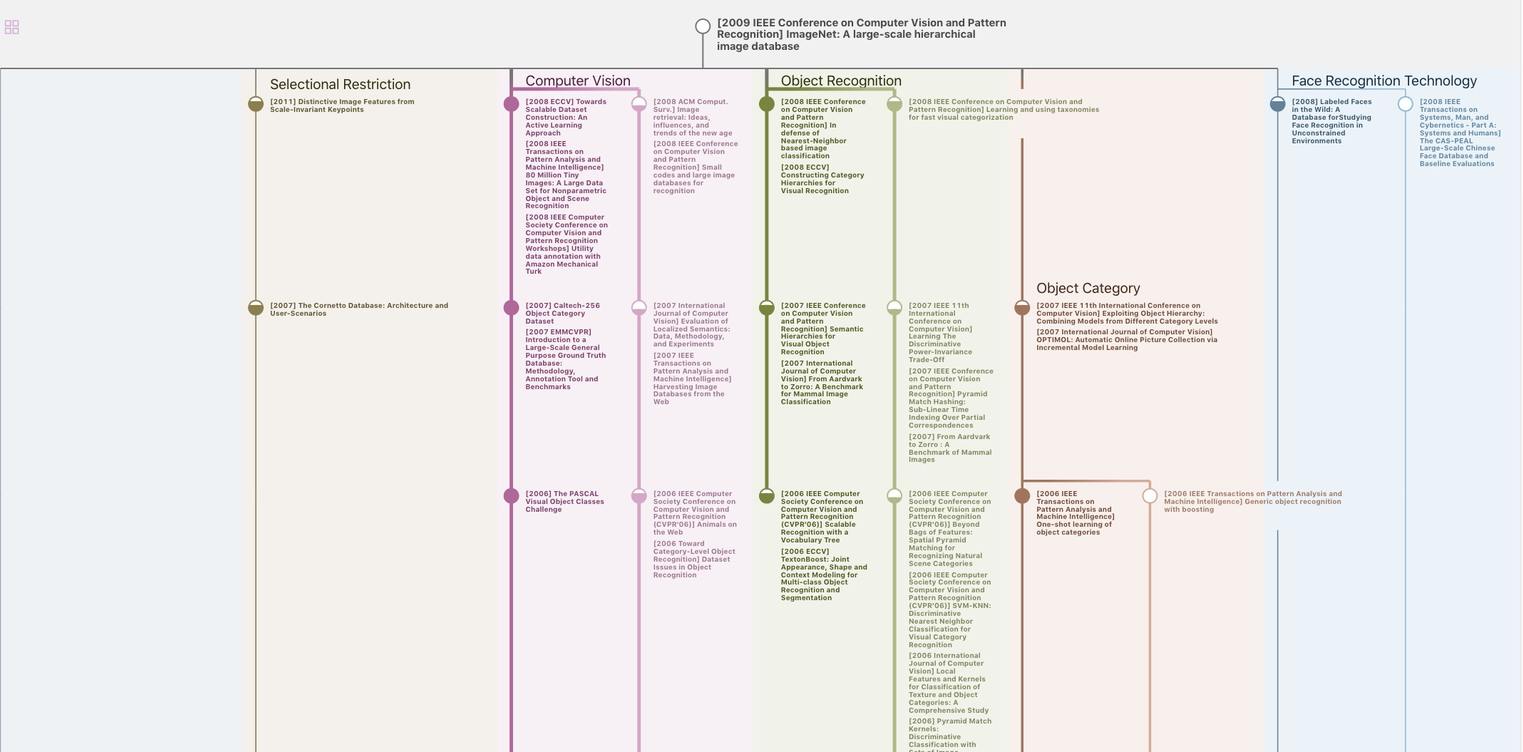
生成溯源树,研究论文发展脉络
Chat Paper
正在生成论文摘要