EUICN: An Efficient Underwater Image Compression Network
IEEE Transactions on Circuits and Systems for Video Technology(2024)
摘要
Thriving ocean applications bring explosive growth of underwater images (UWIs), which urgently demand to be compressed efficiently for transmission in the narrow underwater acoustic channel. However, existing image compression networks achieve suboptimal performance on UWIs. More efficient UWI compression can be achieved by utilizing characteristics of UWIs: (1) Within an UWI, the details distribution is associated with the underwater imaging transmission map (T-map); (2) Different UWIs have higher correlation than terrestrial images because they often present gauzy-covered indistinct appearance and share some universal ocean objects that widely appear in different underwater scenes. This paper fully exploits the two characteristics in terms of quantization and entropy coding, two key components of image compression network. Specifically, we propose an efficient underwater image compression network (EUICN) including underwater T-map-based quantization (UTMQ) and mixture entropy coding (MEC). In which, UTMQ extracts the imaging features from T-map, which are integrated with latent features by a novel dual-spatial attention module (DSAM) to generate a feature reserved mask, adaptively reserving reasonable numbers of features for different regions. For more efficient entropy coding, MEC is designed, which includes three correlation information extraction modules (
i.e
., hyperprior, local and novel universal information) and a probability prediction module. Especially, the universal information extraction module utilizes a comprehensive underwater feature dictionary, which covers various universal ocean objects, to match with latent features to select the universal correlation features. After that, the probability prediction module is designed to consolidate the hyperprior, local, and universal information to predict more accurate probability of latent features. Extensive experiments show that our EUICN achieves better performance than SOTA learned and conventional codecs in terms of PSNR and MS-SSIM.
更多查看译文
关键词
Underwater image compression,Underwater imaging model,Convolution neural network,Quantization,Entropy model
AI 理解论文
溯源树
样例
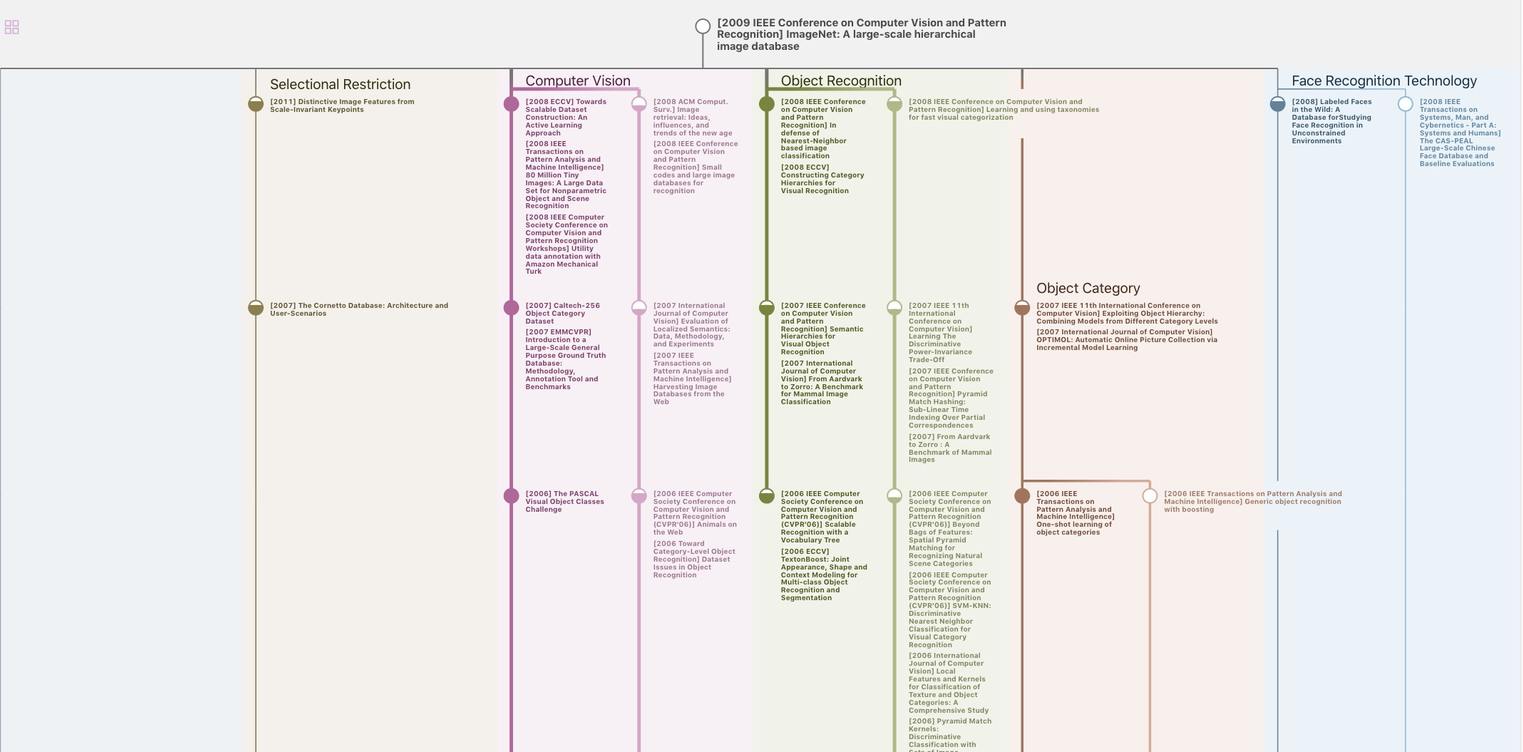
生成溯源树,研究论文发展脉络
Chat Paper
正在生成论文摘要