An Automated Few-Shot Learning for Time Series Forecasting in Smart Grid Under Data Scarcity
IEEE Transactions on Artificial Intelligence(2024)
摘要
Micro-grid can improve greenhouse gas emissions and reduce operational costs. To forecast both energy generation and load demand, time series prediction has been a key tool in real-time control and optimization. Developing an adequate predictive model is difficult when there is a lack of historical data. Moreover, hyperparameters have a tangible impact on the performance of machine learning models. Bearing these considerations in mind, this paper develops a BiLO-Auto-TSF/ML framework that automates the optimal design of a few-shot learning pipeline from a bi-level programming perspective. Specifically, a lower-level meta-learner helps mitigate the small data challenge, whereas an upper-level optimization optimizes both hyperparameters for lower- and upper-level learners. Note that the proposed framework is designed to accommodate a wide range of machine learning methods, allowing for easy integration through a plug-in mechanism. Comprehensive experiments demonstrate the effectiveness of our proposed BiLO-Auto- TSF/ML to search for a high-performance few-shot learning pipeline for various energy sources.
更多查看译文
关键词
Micro-grid design,bi-level programming,few-shot learning,time series forecasting,hyperparameter optimization
AI 理解论文
溯源树
样例
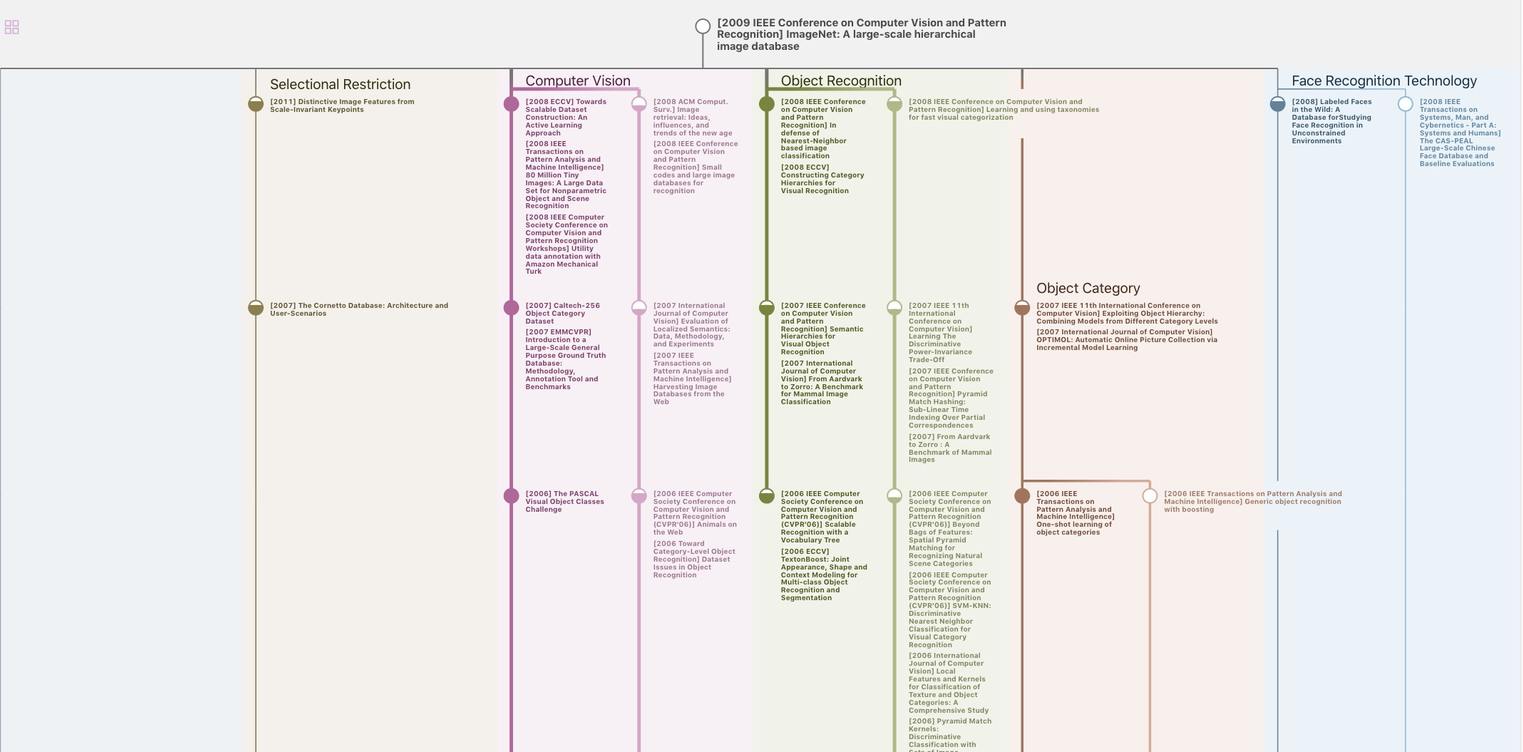
生成溯源树,研究论文发展脉络
Chat Paper
正在生成论文摘要