Hierarchical Multi-Granularity Interaction Graph Convolutional Network for Long Document Classification
IEEE-ACM TRANSACTIONS ON AUDIO SPEECH AND LANGUAGE PROCESSING(2024)
摘要
With the growing demand for text analytics, long document classification (LDC) has received extensive attention, and great progress has been made. To reveal the complex structure and extract the intrinsic feature, the current approaches focus on modeling a long sequence with sparse attention or representing word-sentence or word-section relations partially. However, the thorough hierarchical structure from words, sentences to sections of long documents remains relatively unexplored. For this purpose, we propose a novel Hierarchical Multi-granularity Interaction Graph Convolutional Network (HMIGCN) for long document classification, in which three different granularity graphs, i.e., section graph, sentence graph and word graph, are constructed hierarchically. The section graph encapsulates the macrostructure of a long document, while the sentence and word graphs delve into the document's microstructure. Notably, within the sentence graph, we introduce a Global-Local Graph Convolutional (GLGC) block to adaptively capture both global and local dependency structures among sentence nodes. Additionally, to integrate the three graph networks as a whole, two well-designed techniques, namely section-guided pooling block and transfer fusion block, are proposed to train the model jointly by promoting each other. Extensive experiments on five long document datasets show that our model outperforms the existing state-of-the-art LDC models.
更多查看译文
关键词
Transformers,Computational modeling,Convolutional neural networks,Adaptation models,Speech processing,Task analysis,Context modeling,Long document classification,hierarchical multi-granularity interaction graph convolutional network,hierarchical graph pooling,global-local graph convolution
AI 理解论文
溯源树
样例
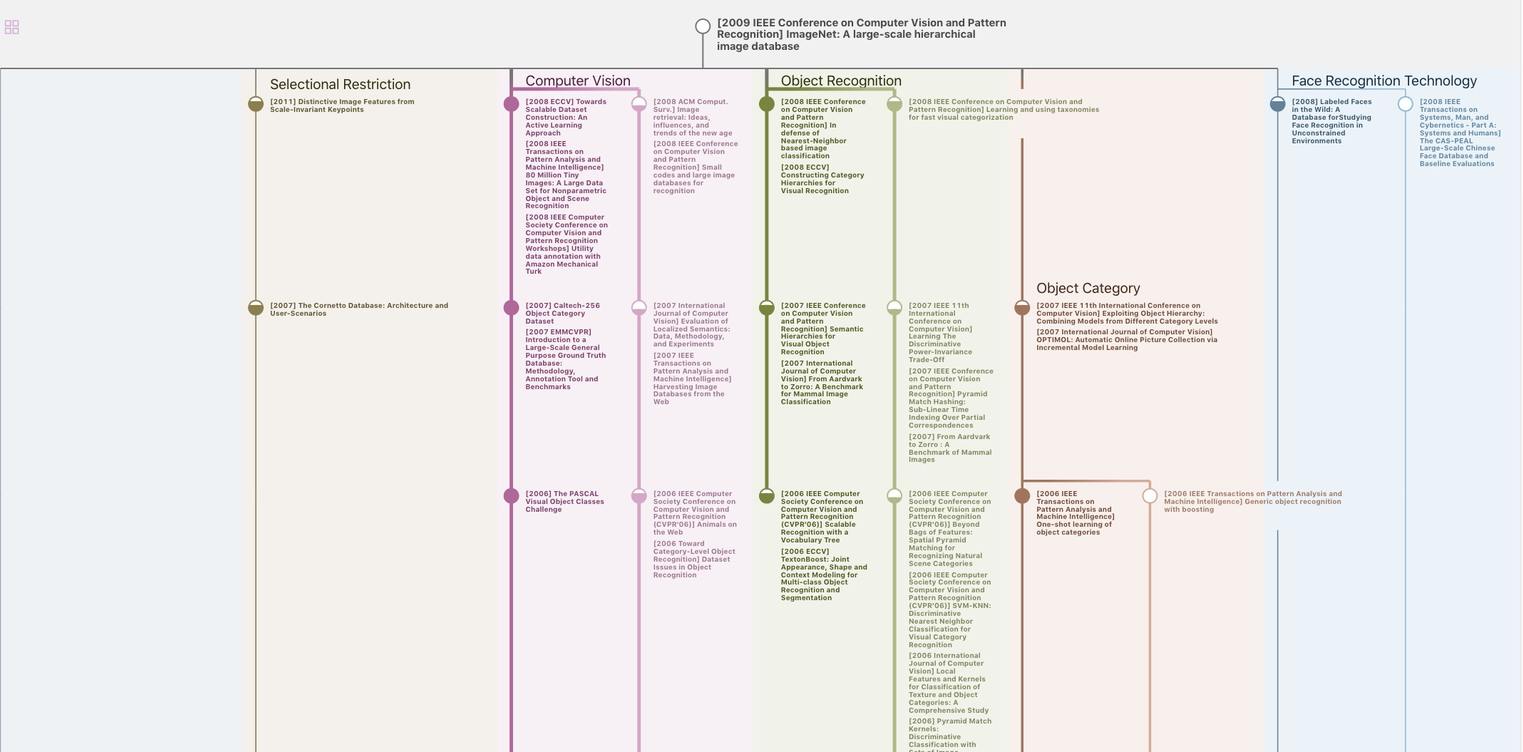
生成溯源树,研究论文发展脉络
Chat Paper
正在生成论文摘要