Privacy Inference Attack and Defense in Centralized and Federated Learning: A Comprehensive Survey
IEEE Transactions on Artificial Intelligence(2024)
摘要
The emergence of new machine learning methods has led to their widespread application across various domains, significantly advancing the field of artificial intelligence. However, the process of training and inferring machine learning models relies on vast amounts of data, which often includes sensitive private information. Consequently, the privacy and security of machine learning have encountered significant challenges. Several studies have demonstrated the vulnerability of machine learning to privacy inference attacks, but they often focus on specific scenarios, leaving a gap in understanding the broader picture. We provide a comprehensive review of privacy attacks in machine learning, focusing on two scenarios: centralized learning and federated learning. This paper begins by presenting the architectures of both centralized learning and federated learning, along with their respective application scenarios. It then conducts a comprehensive review and categorization of related inference attacks, providing a detailed analysis of the different stages involved in these attacks. Moreover, the paper thoroughly describes and compares the existing defense methods. Finally, the paper concludes by highlighting open questions and potential future research directions, aiming to contribute to the ongoing competition between privacy attackers and defenders.
更多查看译文
关键词
Privacy inference attack,Privacy defense,Centralized and Federated learning,Machine learning security
AI 理解论文
溯源树
样例
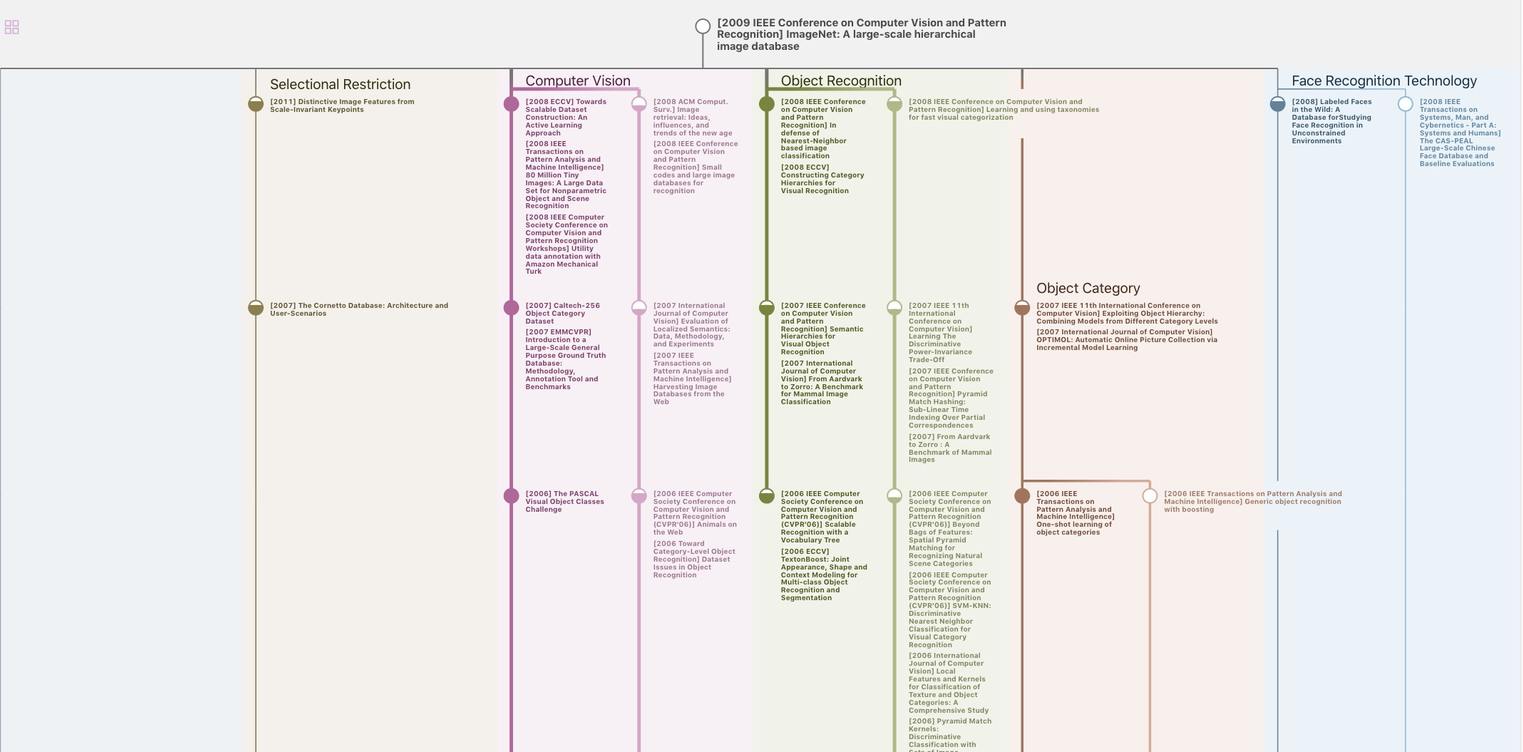
生成溯源树,研究论文发展脉络
Chat Paper
正在生成论文摘要