Latency and Privacy Aware Convolutional Neural Network Distributed Inference for Reliable Artificial Intelligence Systems
IEEE Transactions on Artificial Intelligence(2024)
摘要
Reliable artificial intelligence systems not only propose a challenge on providing intelligent services with high quality for customers, but also require customers’ privacy to be protected as much as possible during process of the services. Given the ultra-high computing load brought by deep learning based intelligent services to edge devices and the ultra-long distance between edge and cloud, low-latency requirement of intelligent services is hard to meet in single edge computing or cloud computing. Edge-cloud collaborative inference of deep neural networks is considered a feasible solution to the problem. However, former work has not reduced the inference latency to the greatest extent and has not considered privacy protection in distributed systems. To solve the problem, we first establish a novel queue mechanism. Then, the convolution layer split decisions are made based on deep reinforcement learning to realize the parallel inference of convolutional neural networks (CNNs) for inference latency reduction. Next, for each CNN, the partition decision is made based on brute force algorithm to further reduce inference latency and protect customers’ privacy. Finally, simulation results show that our method performs better than existing other methods.
更多查看译文
关键词
Reliable artificial intelligence system,CNN parallelism,CNN partition,inference latency,privacy protection
AI 理解论文
溯源树
样例
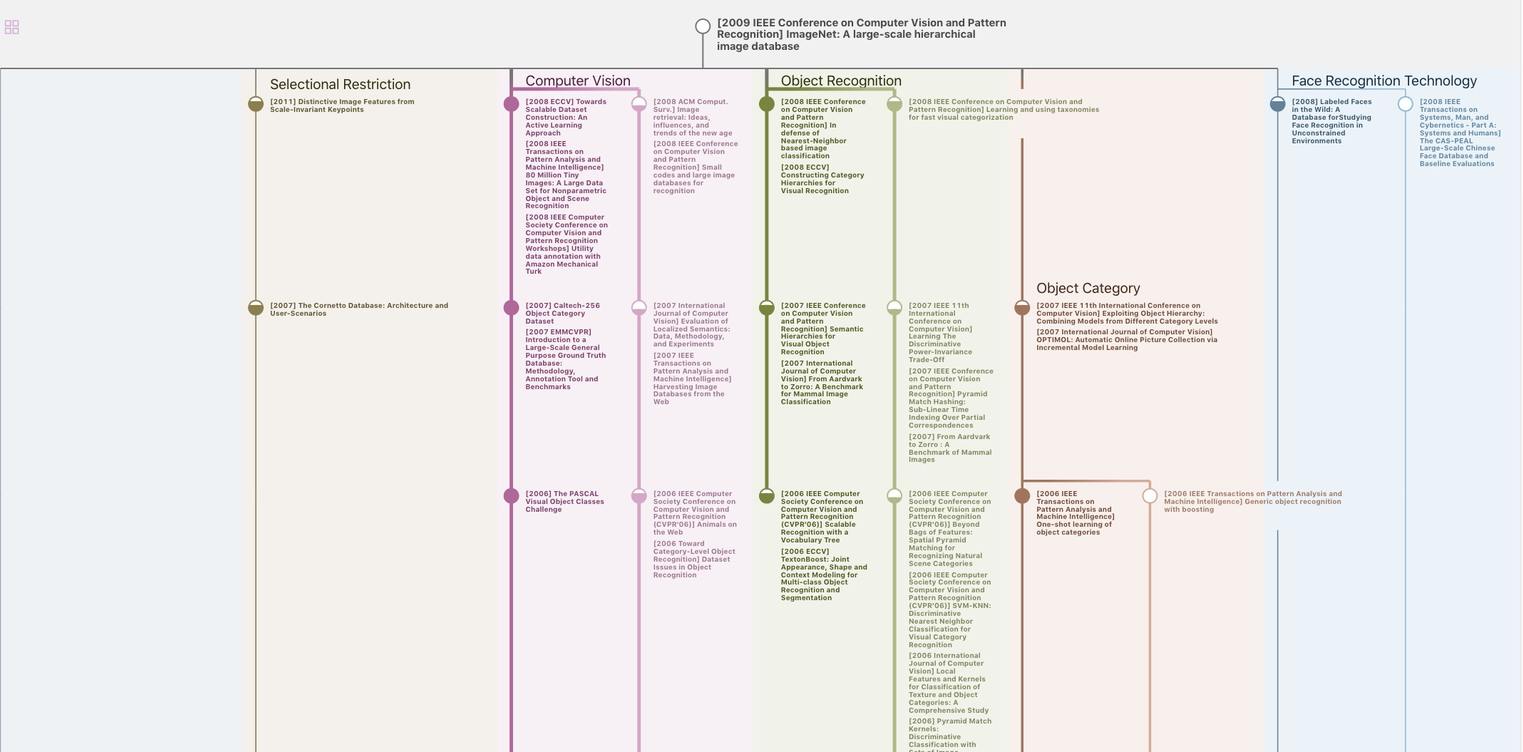
生成溯源树,研究论文发展脉络
Chat Paper
正在生成论文摘要