Adaptive Iterative Learning Control for Nonlinear Multiagent Systems with Initial Error Compensation
IEEE Transactions on Artificial Intelligence(2024)
摘要
In this paper, the consensus tracking problem of neural network (NN)-based adaptive iterative learning control (AILC) scheme is investigated for the nonlinear multiagent systems (MASs) with repetitive control tasks. To achieve the better tracking performance, the NN is developed to iteratively learn the uncertain nonlinearity. Meanwhile, the gradient descent algorithm is utilized to train NN weights, which is different from the traditional method based on σ-modification. For initial error problems of AILC, an initial error compensation method is developed to deal with the initial consensus tracking error. During the AILC design using the dynamic surface control technique, an auxiliary control signal is introduced to handle the residual term. Then, the boundedness of all signals and the iterative convergence of tracking errors are analyzed by the Lyapunov theory. Finally, a simulation of the multi-manipulators is provided to validate the effectiveness of the proposed control algorithm.
更多查看译文
关键词
Adaptive iterative learning control (AILC),consensus tracking,initial error compensation,multiagent systems (MASs),neural network (NN)
AI 理解论文
溯源树
样例
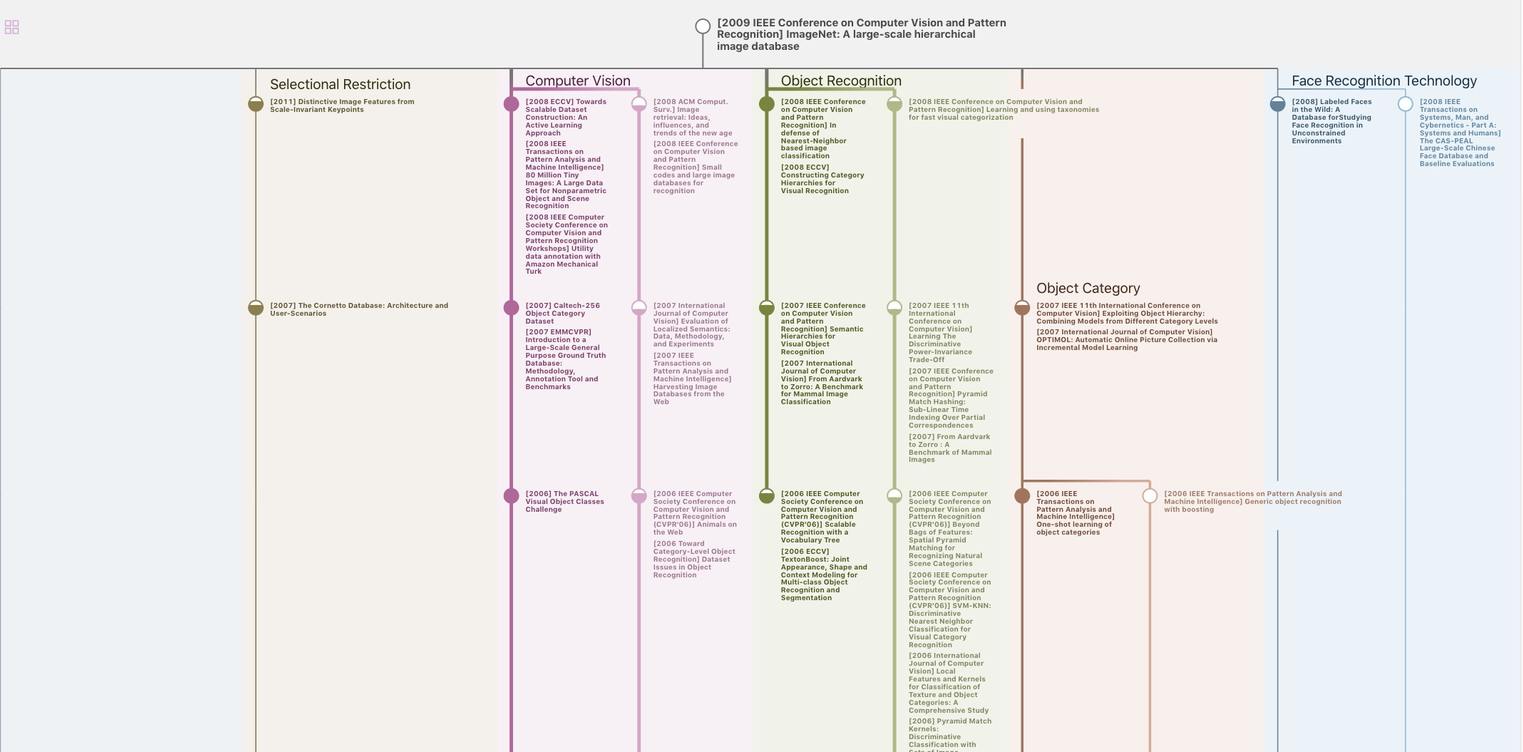
生成溯源树,研究论文发展脉络
Chat Paper
正在生成论文摘要