Spectral-Spatial Large Kernel Attention Network for Hyperspectral Image Classification
IEEE TRANSACTIONS ON GEOSCIENCE AND REMOTE SENSING(2024)
摘要
Due to its ability to capture long-range dependencies, self-attention mechanism-based transformer models are introduced for hyperspectral image (HSI) classification. However, the self-attention mechanism has only spatial adaptability but ignores channel adaptability, thus cannot well extract complex spectral-spatial information in HSIs. To tackle this problem, in this article, we propose a novel spectral-spatial large kernel attention network (SSLKA) for HSI classification. SSLKA consists of two consecutive cooperative spectral-spatial attention blocks with large convolution kernels, which can efficiently extract features in spectral and spatial domains simultaneously. In each cooperative spectral-spatial attention block, we employ the spectral attention branch and the spatial attention branch to generate the attention maps, respectively, and then fuse the extracted spatial features with the spectral features. With large kernel attention (LKA), we can enhance the classification performance by fully exploiting local contextual information, capturing long-range dependencies, as well as being adaptive in the channel dimension. Experimental results on widely used benchmark datasets show that our method achieves higher classification accuracy in terms of overall accuracy (OA), average accuracy (AA), and Kappa than several state-of-the-art methods.
更多查看译文
关键词
Feature extraction,Convolution,Kernel,Hyperspectral imaging,Convolutional neural networks,Task analysis,Image classification,Hyperspectral image (HSI) classification,large kernel attention (LKA),spectral-spatial attention
AI 理解论文
溯源树
样例
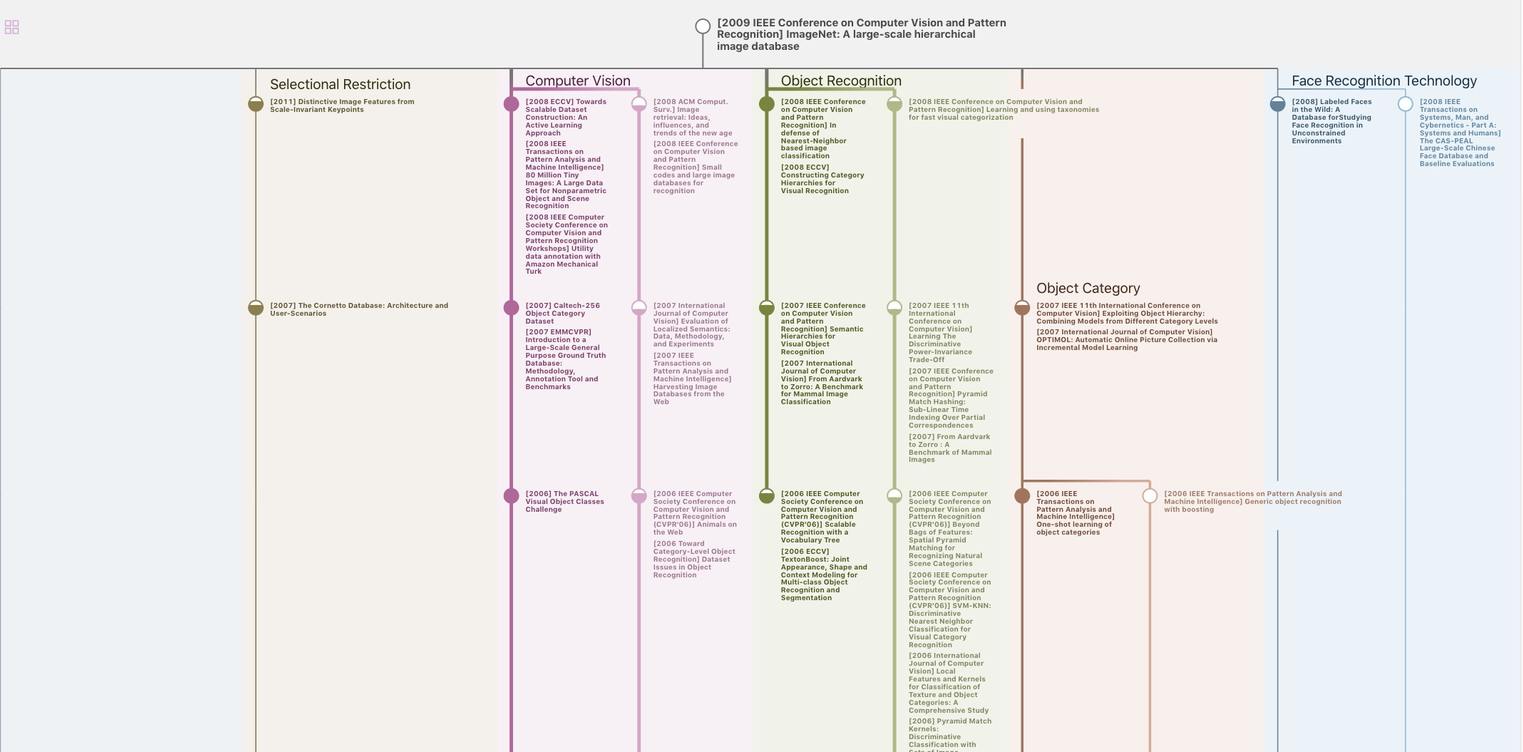
生成溯源树,研究论文发展脉络
Chat Paper
正在生成论文摘要