Cortical Network Transformer for Classification of Structural Connectivity in Cerebral Cortex
IEEE TRANSACTIONS ON INSTRUMENTATION AND MEASUREMENT(2024)
摘要
Analyzing the structural connectivity of the cerebral cortex is of great help to the diagnosis and tracking of mental illnesses such as major depression disorder (MDD), and it can also be used to study the internal factors that affect brain function by exploring the changes in the topological structure of the cerebral cortex. However, most current methods cannot be applied to non-Euclidean domains to capture the topological information of structural connectivity. Therefore, in this article, we construct structural connectivity networks using features of the cerebral cortex and propose a cortical network transformer (CNT) to classify the networks. For the first time, we introduce Graph Transformer into the study of cortical structural connectivity, using the random walk position encoding (RWPE), and relative position encoding based on self-attention scores of positive definite kernels on the graph. Compared with general graph neural networks (GNNs), Graph Transformers can better capture long-distance dependencies on graphs. In addition, our model can better capture the positional relationship and correlation of nodes. Experimental results show that the CNT model achieves good results in gender classification and MDD diagnosis tasks on human connectome project (HCP) and MDD datasets. On the HCP dataset, the accuracy, specificity, and sensitivity are 83.38%, 84.84%, and 81.65%. In addition, the accuracy of the MDD dataset also reaches 74.71%. The proposed method is superior or comparable to the state-of-the-art models.
更多查看译文
关键词
Transformers,Cerebral cortex,Brain modeling,Depression,Task analysis,Feature extraction,Encoding,graph representation learning,graph transformer,major depressive disorder (MDD),structural connectivity
AI 理解论文
溯源树
样例
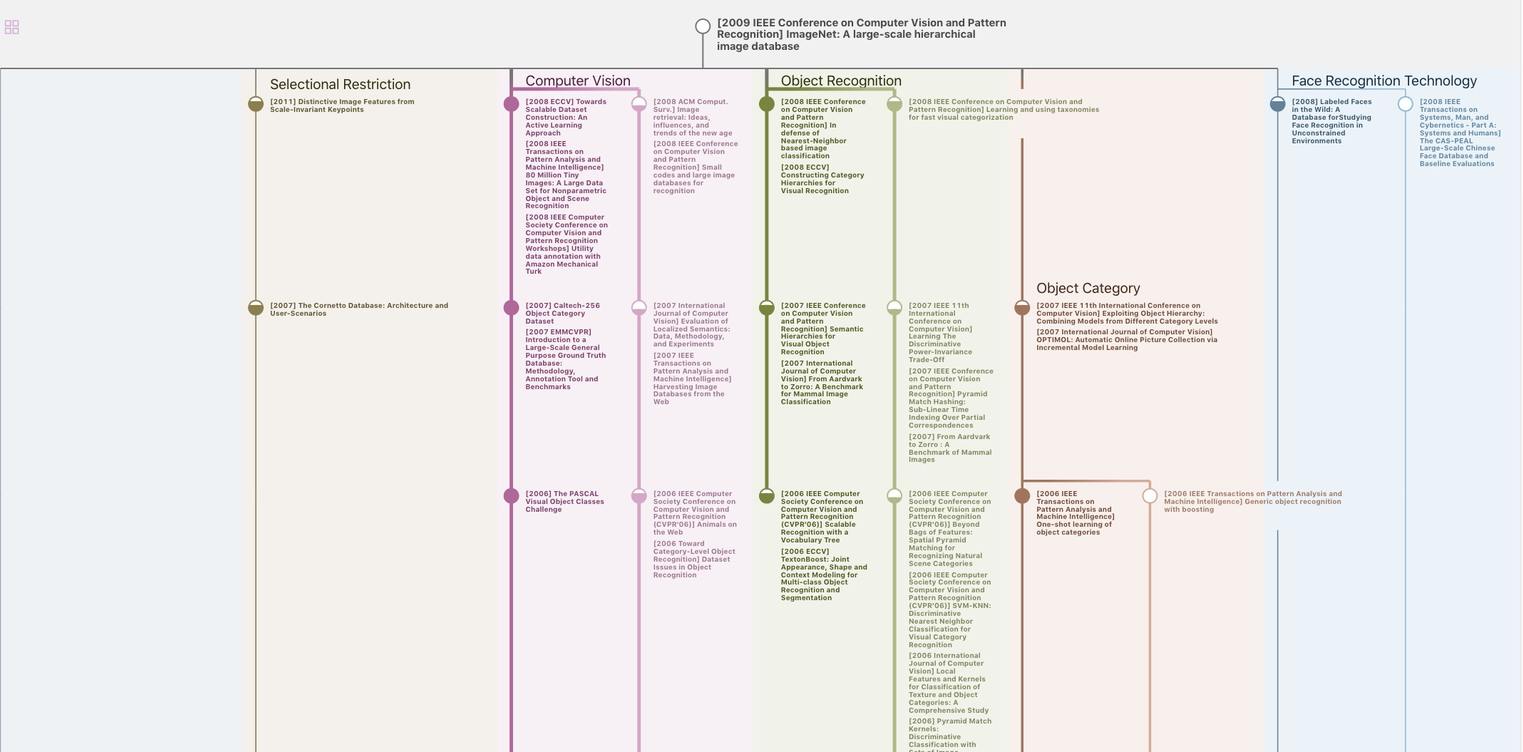
生成溯源树,研究论文发展脉络
Chat Paper
正在生成论文摘要