Infusion Prior Information Prismatic Generative Adversarial Learning Network for Multi-material Decomposition in Dual-bin Photon Counting Computed Tomography
2023 3rd International Conference on Electronic Information Engineering and Computer (EIECT)(2023)
摘要
Spectral computed tomography based on photon-counting detector (PCCT) is a promising technique with high spatial and energy resolution. Multi-material decomposition is a typical application of PCCT, which can provide accurate material identification. However, due to the finite photons in each energy bin, the number of energy bins cannot grow without limit while the image quality suffers from serious noise. Despite the quantitative capability for multi-material, existing methods require adequate energy bins corresponding to the number of materials. In this paper, an infusion prior information prismatic generative adversarial learning network is proposed based on generative adversarial learning for multi-material decomposition in dual-bin PCCT. First, to alleviate the pathological nature, prior images corresponding to full spectrum is introduced into the solution. Second, generative adversarial leaning is utilized to characterization the material with its nonlinear calculation capability. Thirdly, the idea of interactive structure is draw for multi-material decomposition task. Numerical experiments based on structured fibro-glandular tissue data were carried out to verify the effectiveness of the proposed method. The results demonstrate its accuracy on material quantification over other methods in this field under the condition of finite energy bins.
更多查看译文
关键词
Spectral Computed Tomography,Photon Counting Computed Tomography,Prior Image,Generative Adversarial Learning,Interactive Structure
AI 理解论文
溯源树
样例
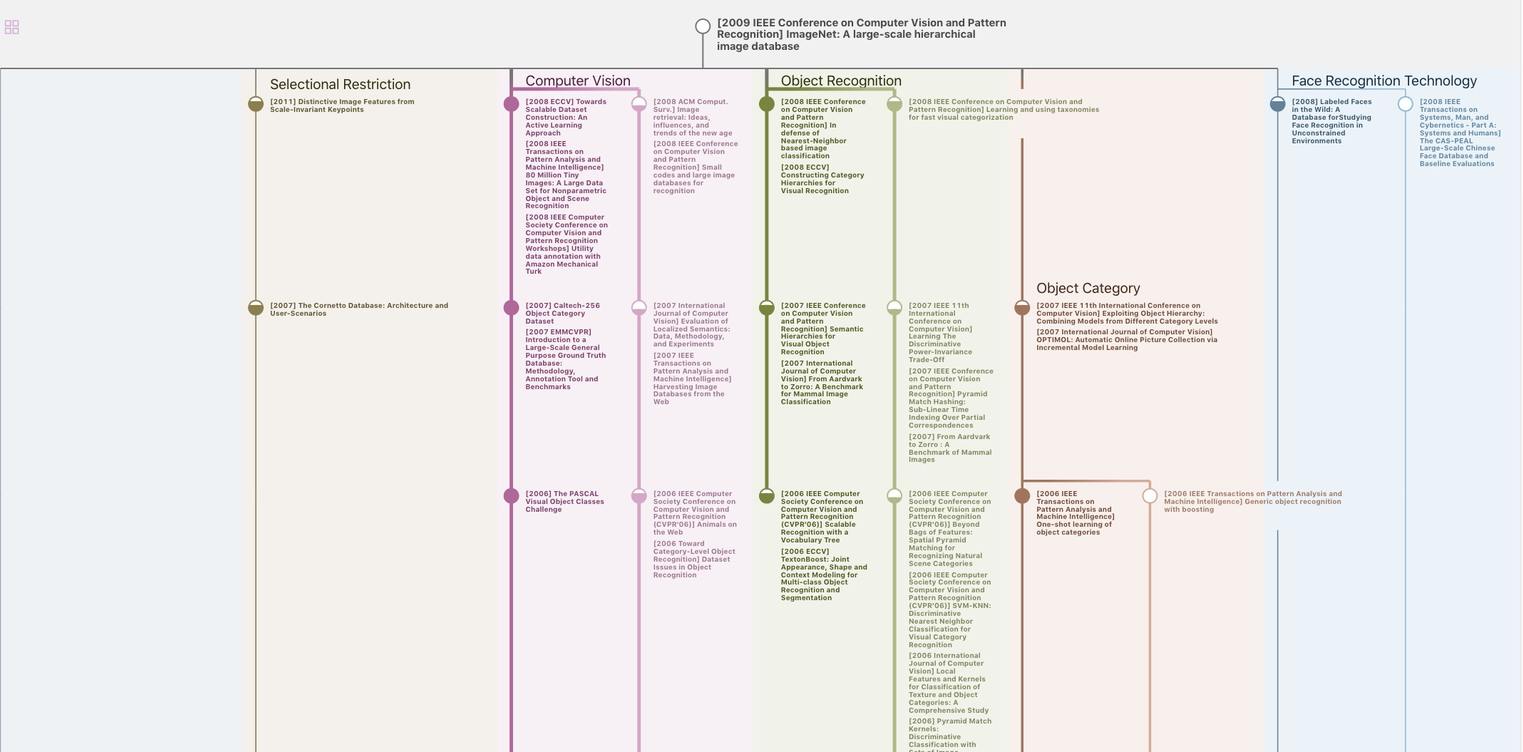
生成溯源树,研究论文发展脉络
Chat Paper
正在生成论文摘要