Prediction of specific surface area of metal–organic frameworks by graph kernels
The Journal of Supercomputing(2024)
摘要
Metal–organic frameworks (MOFs) are networks of metal ions or clusters bonded by organic ligands. Their exceptional micro-porosity and vast surface area make them versatile in various applications. Despite MOFs’ extensive potential, much remains unknown regarding their properties and applications across fields. Tunable micro-porosity depends on metal and ligand combinations, but determining the optimal pairings presents challenges. We aim to identify potential optimal candidates, employing graph kernels to study organic ligand structural characteristics. This machine learning approach enhances MOF development efficiency without the need for synthesis. The atomic graph accurately represents structural features, with graph kernels assessing similarities. A unified kernel, utilizing a weighted RBF kernel, measures MOF similarity and predicts ideal metal and ligand combinations. A support vector machine classification algorithm facilitates metal similarity assessment. This method can yield high specific surface area MOFs. Experiments using data from the CoRE MOF dataset demonstrate accurate predictive model generation for six of the seven well-known graph kernels in the literature.
更多查看译文
关键词
Graph kernel,Metal–organic framework,Machine learning
AI 理解论文
溯源树
样例
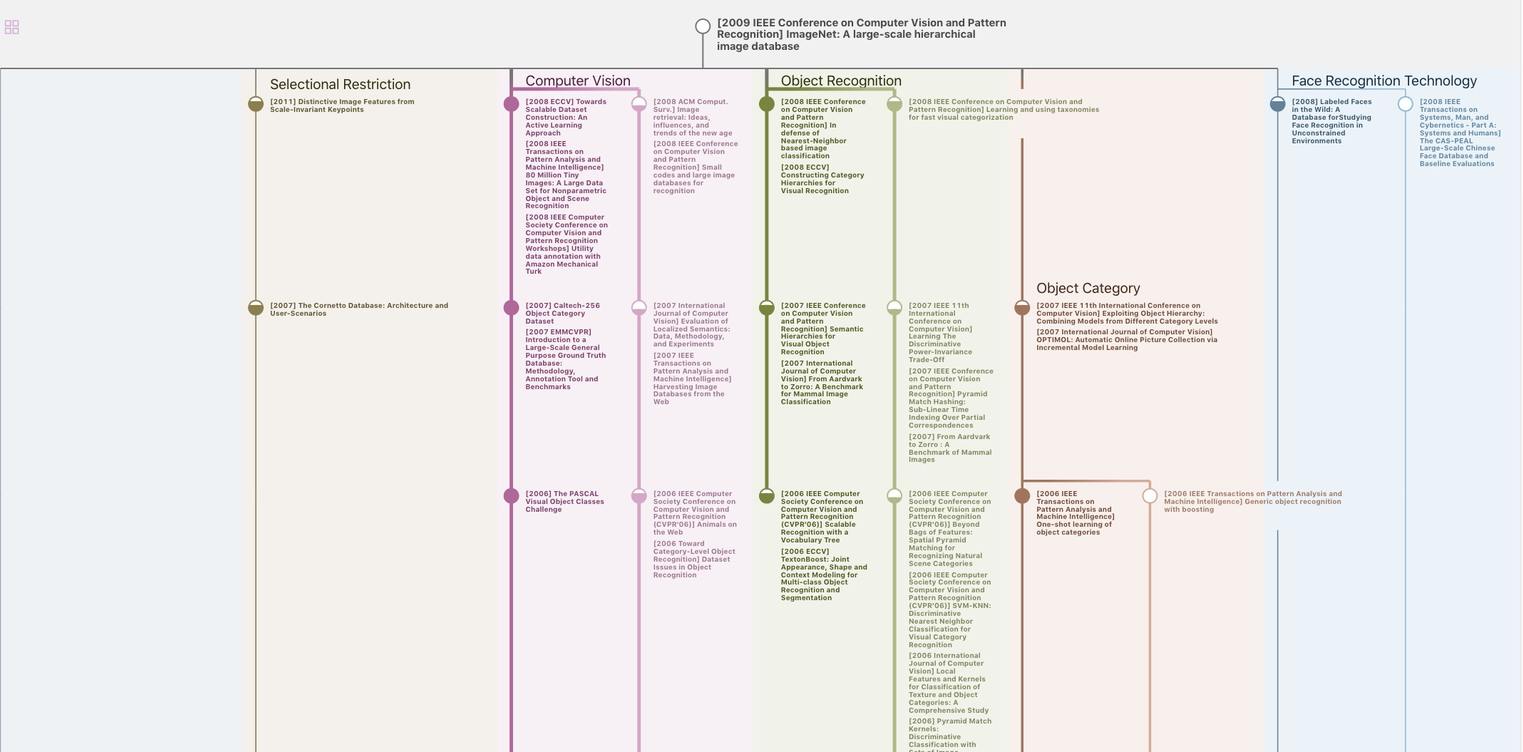
生成溯源树,研究论文发展脉络
Chat Paper
正在生成论文摘要