Multi-Task Learning Based Channel Estimation for Hybrid-Field STAR-RIS Systems
IEEE CONFERENCE ON GLOBAL COMMUNICATIONS, GLOBECOM(2023)
摘要
A joint cascaded channel estimation framework is proposed for simultaneously transmitting and reflecting reconfigurable intelligent surfaces (STAR-RIS) systems with hardware imperfection, in which practical the hybrid-field electromagnetic wave radiation with spatial non-stationarity is investigated. By exploiting the cascaded channel correlations in user domain and STAR-RIS element domain, we propose a multi-task network (MTN) with multi-expert branches to simultaneously reconstruct the high-dimensional transmitting and reflecting channels from the observed mixture channel with noise. In the proposed MTN architecture, a learnable shrinkage module is exploited to constrict the communication noise, and self-attention mechanism-based Transformer layers are utilized to extract the non-local feature of the non-stationary cascaded channel. Numerical results show that the proposed MTN achieves superior channel estimation accuracy with less training overhead compared with existing state-of-the-art benchmarks, in terms of required pilots, computations, and network parameters.
更多查看译文
关键词
Channel Estimation,Multi-task Learning,Channel Correlation,Reconfigurable Intelligent Surface,Training Overhead,Channel Estimation Accuracy,Multi-task Network,Spatial Non-stationarity,Denoising,Information Exchange,Convolutional Layers,Feature Maps,Lognormal,Least Squares Estimation,Time Slot,Channel Model,Path Loss,Adaptive Learning,Sparse Representation,Array Elements,Near Field Communication,Near-field Region,Multi-task Learning Framework,Array Response,Error Vector Magnitude,Normalized Mean Square Error,Shared Layers,Hardware Impairments,Shared Features,Linear Approximation
AI 理解论文
溯源树
样例
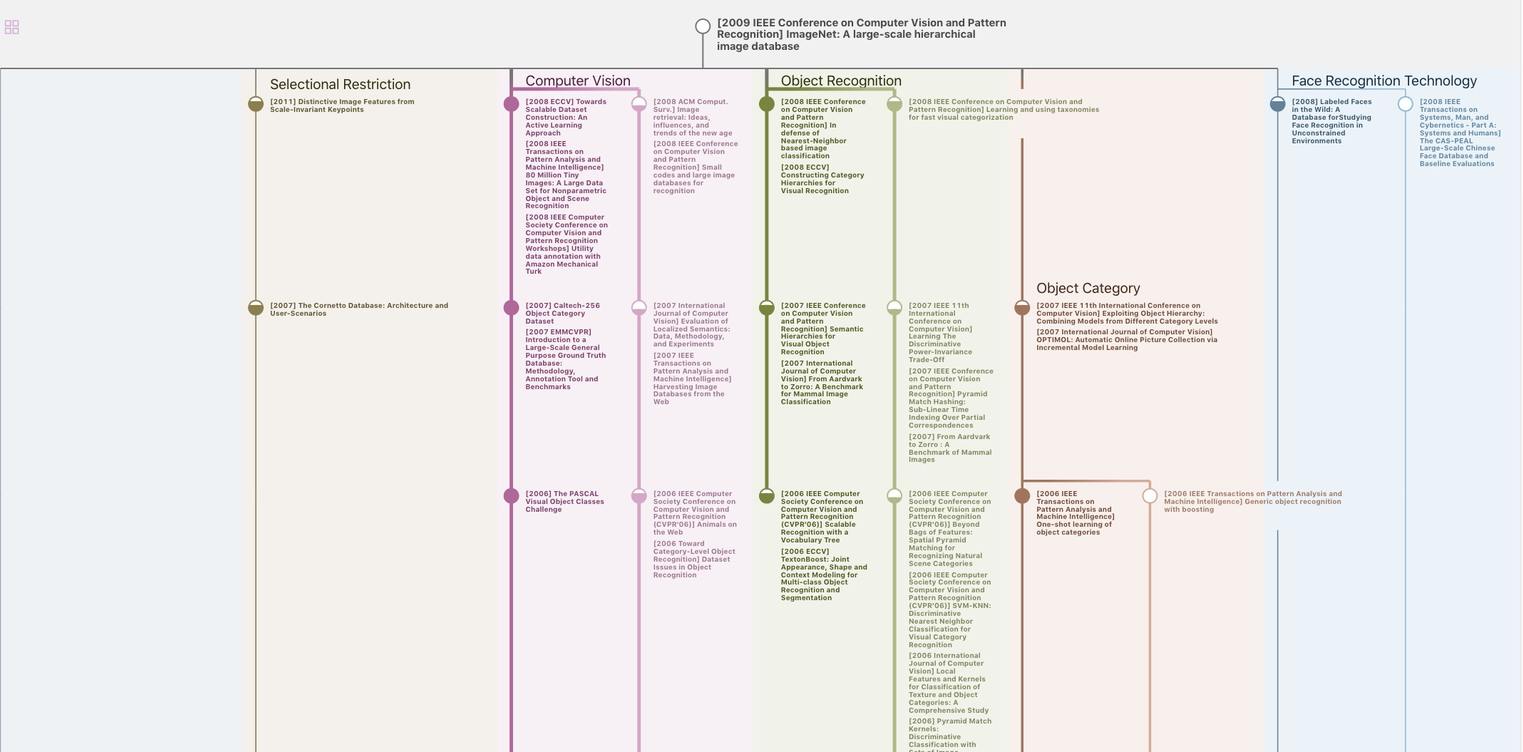
生成溯源树,研究论文发展脉络
Chat Paper
正在生成论文摘要