CN-BSRIQA: Cascaded network- blind super-resolution image quality assessment
ALEXANDRIA ENGINEERING JOURNAL(2024)
摘要
High resolution (HR) images consist of higher quality and more detail information in comparison to lowresolution images. But obtaining HR images entails higher costs and requires a larger workforce. The solution is using super -resolution (SR) images. Single image SR is the process of creating HR images from a single lowresolution image. Obtaining SR images from low -resolution images is a well-known problem in the domains of computer vision and image processing. Advancement in technology has given rise to many SR algorithms. The perceptual quality assessment of super -resolved images can be used to benchmark the techniques employed for image SR. In this work, a cascaded network -blind super -resolution image quality assessment (CN-BSRIQA) methodology is proposed. The proposed approach works under the cascaded architecture where a convolutional neural network (CNN) is cascaded with a deep belief network (DBN). CNN is employed as a shallow network in the proposed methodology to extract low-level information after partitioning the input image into patches. To assess the visual quality of the SR images, the features retrieved from CNN are incorporated into a DBN. Three databases are used to evaluate the performance of proposed CN-BSRIQA i.e., SR Quality Database (SRQD), SR Image Quality Database (SRID), and visual quality evaluation for super -resolved images (QADS). When compared to other state-of-the-art methodologies for assessing the visual quality of SR images, CN-BSRIQA outperforms them. For the perceptual quality assessment of SR images, the experimental results reveal that CNN -based techniques outperform techniques based on hand-crafted features. Furthermore, the shallow CNN proposed in the CN-BSRIQA can extract features that are content -independent i.e., they show better performance over cross -database evaluation in comparison to existing state-of-the-art techniques.
更多查看译文
关键词
Cascaded network,Deep belief network,Convolution neural network,Super-resolution,Quality assessment
AI 理解论文
溯源树
样例
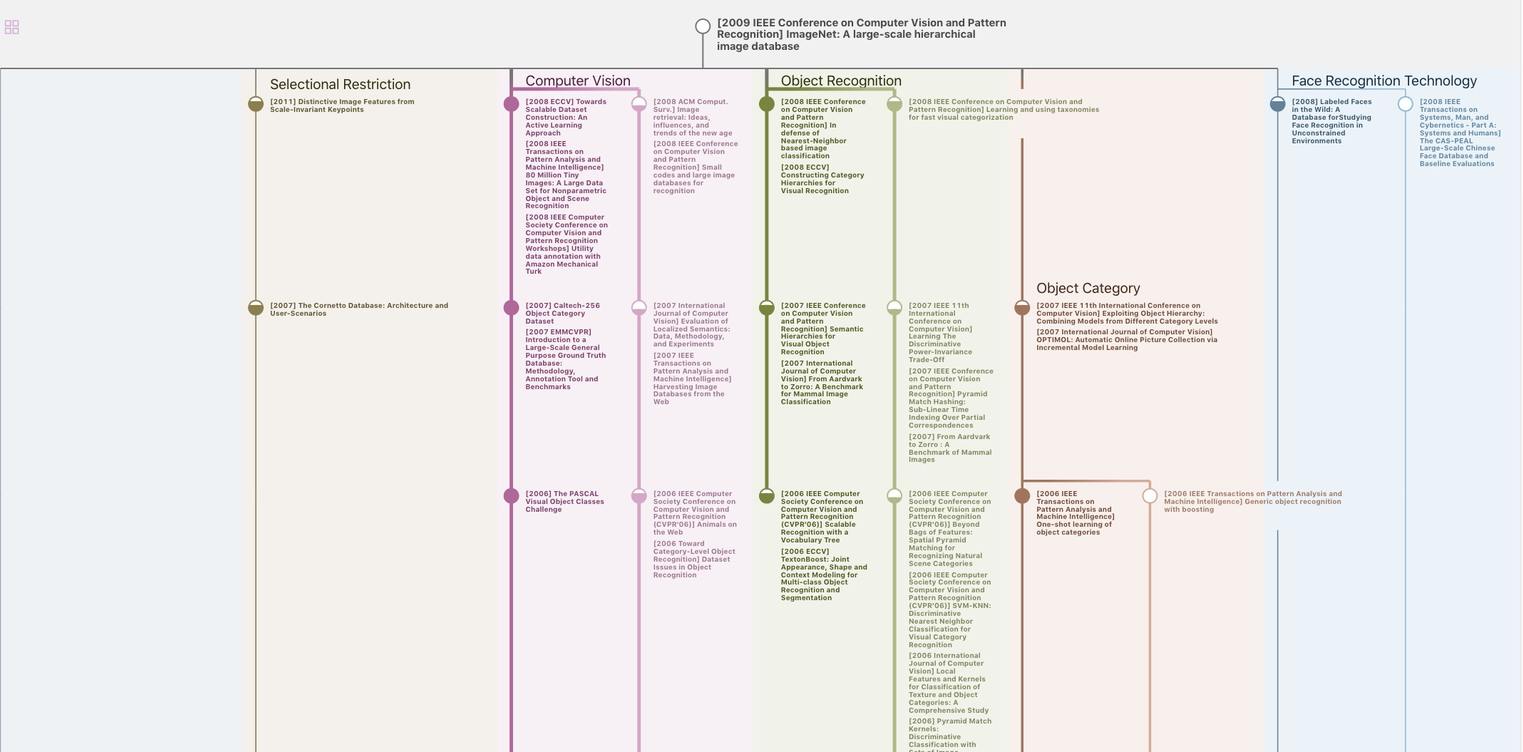
生成溯源树,研究论文发展脉络
Chat Paper
正在生成论文摘要