Network-Friendly Sequential Recommendation with Quality Constraints: A Safe Deep Reinforcement Learning Approach
IEEE CONFERENCE ON GLOBAL COMMUNICATIONS, GLOBECOM(2023)
摘要
Network-friendly recommendation have emerged as a promising approach to relieve data traffic congestion without sacrificing user preference. Most of existing works focus on one-stage recommendations, maximizing recommendation quality and reducing network latency for the next request. In this paper, we focus on policy optimization for Network-friendly Sequential Recommendation (NSR), towards maximizing the recommendation quality as well as network performance for the whole session with hard quality constraints. To achieve this goal, we first formulate the NSR problem as a Markov Decision Process (MDP) problem. To characterize the fundamental performance limit, we consider the offline solution by assuming the distributional knowledge of user behavior is known as a prior. In this case, we solve the offline problem through policy iteration. However, user behavior in real-world scenarios is unpredictable, which makes it difficult to know the distributional knowledge of users. To handle this issue, we propose a proximal policy optimization-based algorithm with a safe layer, NSR-PPOSL, to seek NSR online solution. Through extensive simulations, we show that the proposed online method achieves over 80.0% performance of the offline method, under the condition of unknown user behavior. Moreover, our proposed online method outperforms representative benchmark by 13.5% under various network conditions and user behaviors.
更多查看译文
关键词
Network-Friendly Recommendation,Policy Iteration,Deep Reinforcement Learning,Safe Layer
AI 理解论文
溯源树
样例
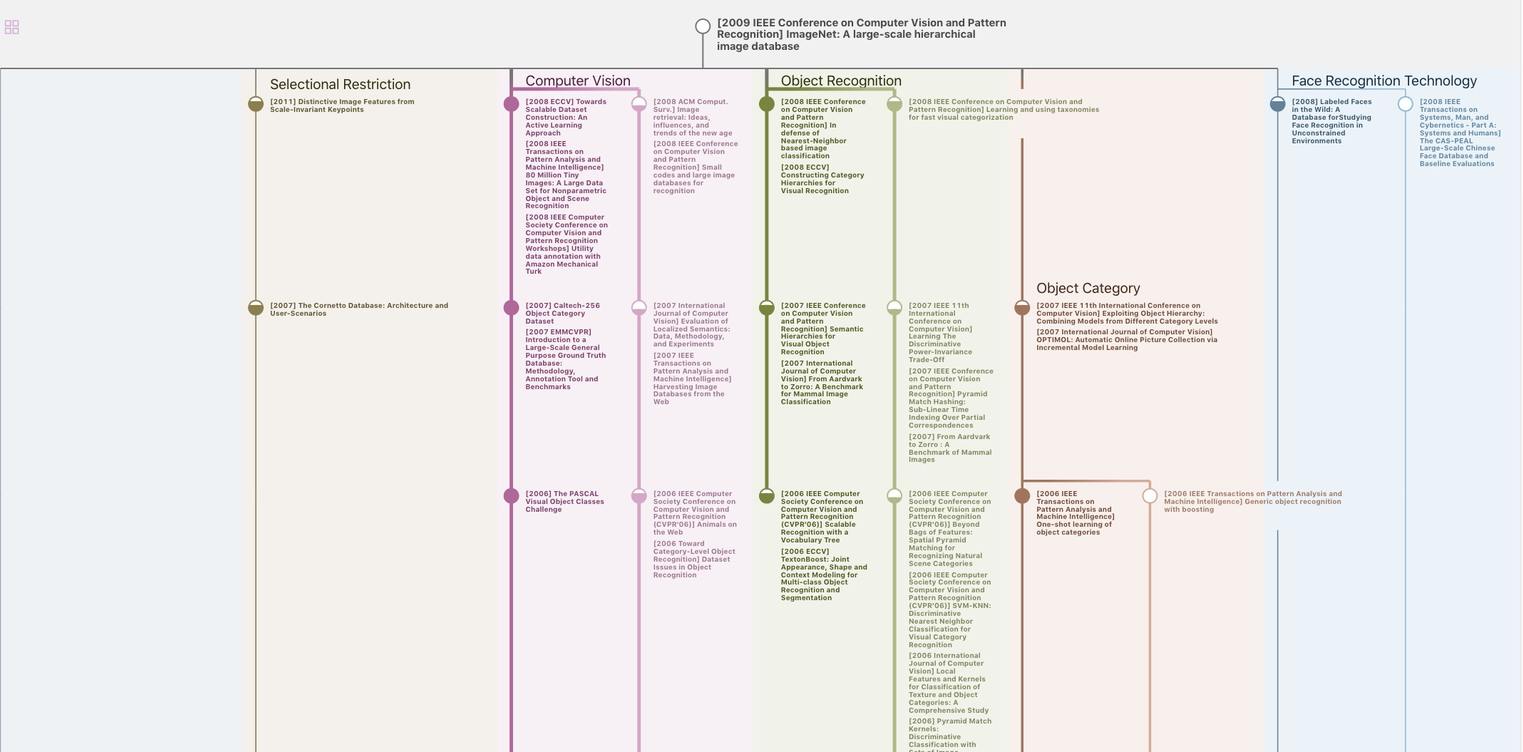
生成溯源树,研究论文发展脉络
Chat Paper
正在生成论文摘要