Enhanced Federated Reinforcement Learning for Mobility-Aware Node Selection and Model Compression
IEEE CONFERENCE ON GLOBAL COMMUNICATIONS, GLOBECOM(2023)
摘要
Federated Learning (FL) is an emerging distributed learning architecture that allows multiple agents to share knowledge in machine learning. However, in the scenario with mobile agents, the mobility of agents significantly affects the learning performance. Besides, frequent exchange of local models could result in high communication overhead. In this paper, we propose a Mobility-aware Federated Reinforcement Learning (MFRL) framework. In MFRL, we model the influences of agent mobility on communication quality and data correlation, and devise a mobility-aware node selection algorithm, so as to accelerate the training procedure and improve the learning performance, taking learning quality, wireless channel quality, and data correlation of agents into consideration. A knowledge distillation (KD) based model compression method is integrated into the MFRL to reduce the communication overhead as well as accelerate the inference process. Finally, taking deep reinforcement learning (DRL) based collision avoidance of intelligent vehicles as a study case, the effectiveness of MFRL is verified. Numerical results demonstrate that the proposed MFRL can accelerate the training process and improve the learning performance.
更多查看译文
关键词
Federated reinforcement learning,mobility awareness,model compression
AI 理解论文
溯源树
样例
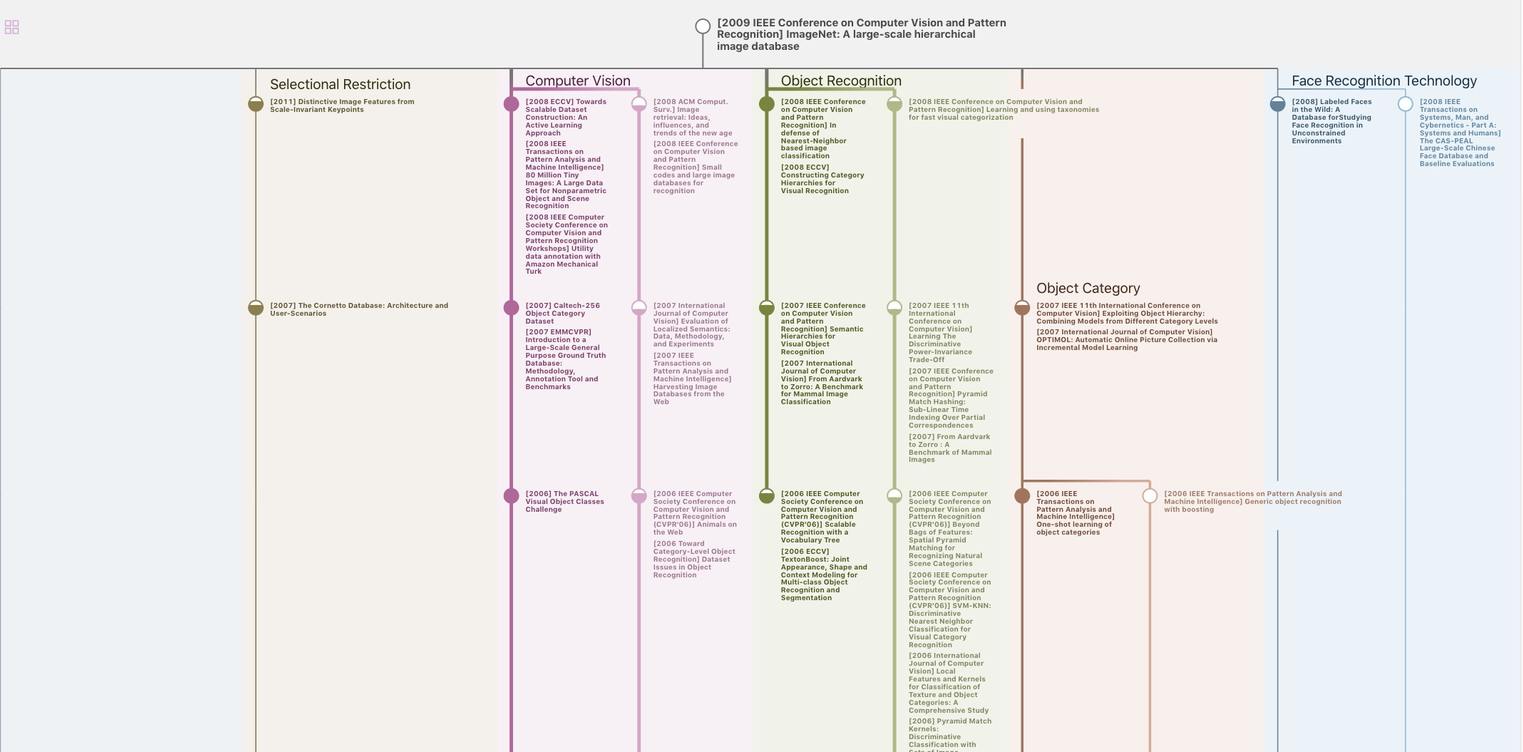
生成溯源树,研究论文发展脉络
Chat Paper
正在生成论文摘要