RL-Edge Relocator: a Reinforcement Learning based approach for Application Relocation in Edge-enabled 5G System
IEEE CONFERENCE ON GLOBAL COMMUNICATIONS, GLOBECOM(2023)
摘要
The convergence of 5G and Edge computing has revolutionized the technology landscape, ushering in a new era of innovative use cases and accelerating the realization of an intelligent, fully connected digital world at an unprecedented pace. However, the demanding and ever-changing specifications of these emerging applications present significant challenges in terms of orchestration, requiring innovative solutions and efficient management strategies to ensure optimal performance and resource utilization. The mobility of end-users is a critical factor expected to significantly impact Edge operations. As a result, Edge-enabled 5G systems face the daunting task of tracking moving users and meeting their Quality of Experience (QoE) demands simultaneously, which presents a formidable technical challenge that must be addressed for the realization of a truly seamless and ubiquitous user experience. In this paper, we put forward a novel solution based on Reinforcement Learning to optimize the relocation of applications between Edge Hosts while meeting their demands in terms of latency and resource requirements. Our proposed solution aims to achieve a balance between two key objectives: maintaining the Quality of Service (QoS) of Edge Applications and optimizing the utilization of infrastructure resources. By achieving this balance, we can ensure that Edge-enabled 5G systems operate efficiently, with minimal resource wastage and maximal QoS satisfaction for end-users. The intensive simulations and evaluation results demonstrate its effectiveness in terms of QoS assurance and resource load balancing.
更多查看译文
关键词
5G,Edge Computing,Edge Relocation,Migration,Orchestration,Reinforcement Learning,Machine Learning
AI 理解论文
溯源树
样例
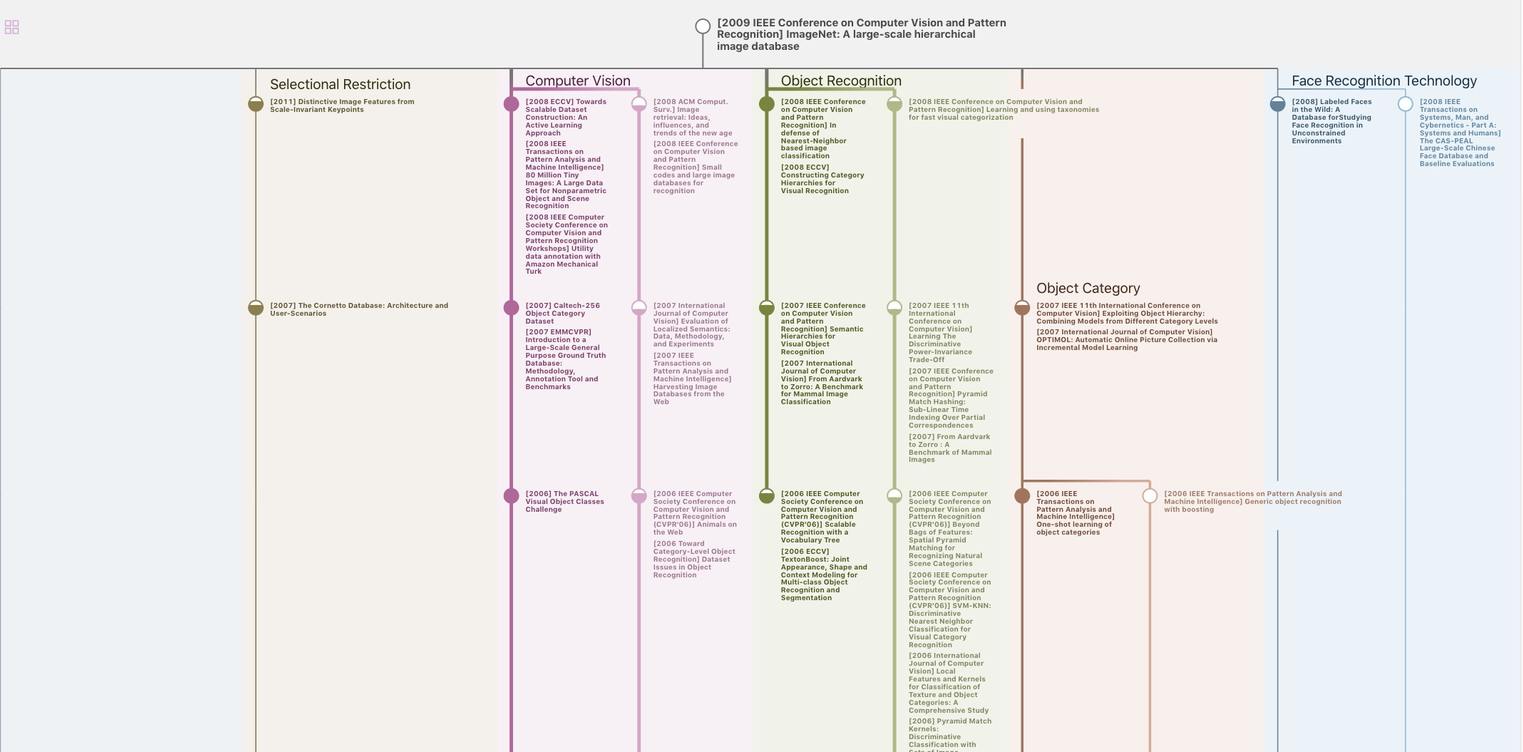
生成溯源树,研究论文发展脉络
Chat Paper
正在生成论文摘要