Efficient and Privacy-Preserving Logistic Regression Prediction over Vertically Partitioned Data
IEEE CONFERENCE ON GLOBAL COMMUNICATIONS, GLOBECOM(2023)
摘要
The explosive growth of data scales has recently drawn extensive attention to machine learning (ML) prediction services for achieving effective and efficient decision-making. However, the potential risk of model or data leakage, as well as vertically partitioned query data in real-world scenes, bring many challenges to ML prediction. Therefore, this paper proposes an efficient and privacy-preserving logistic regression prediction scheme, through which query institutions with vertically partitioned data can enjoy secure, fast, and high-accuracy prediction services from one cloud service provider. Specifically, we take the efficiency advantage of the trusted execution environments (TEE) technique under a semi-honest model, and design a series of masking and recovery methods based on homomorphic encryption to guarantee privacy inside and outside the enclave. Moreover, the ciphertext packing technique is integrated into our scheme for processing predictions parallelly, which further reduces communication and computational overhead. As a result, our scheme will not introduce additional strong security assumptions to TEE; meanwhile, it achieves a trade-off between security and efficiency. Based on the simulation-based paradigm, we formally prove the selective security of our scheme. Extensive experiments on real-world and synthetic datasets show that our prediction accuracy is comparable with plaintext prediction, and our scheme has at least a 30x running speedup compared to the existing representative schemes.
更多查看译文
关键词
Logistic Regression Prediction,Prediction Accuracy,Plaintext,Real-world Datasets,Information Leakage,Computational Overhead,Prediction Scheme,Recovery Method,Communication Overhead,Query Data,Machine Learning Prediction,Real-world Scenes,Fast Prediction,Fast Service,Masking Method,Model Parameters,Model System,Decoding,Characteristics Of Data,Public Key,Well-trained Model,Security Model,Random Number,Yield Prediction,Secret Key,Multi-party Computation,Running Time,Chosen-plaintext,Key Generation
AI 理解论文
溯源树
样例
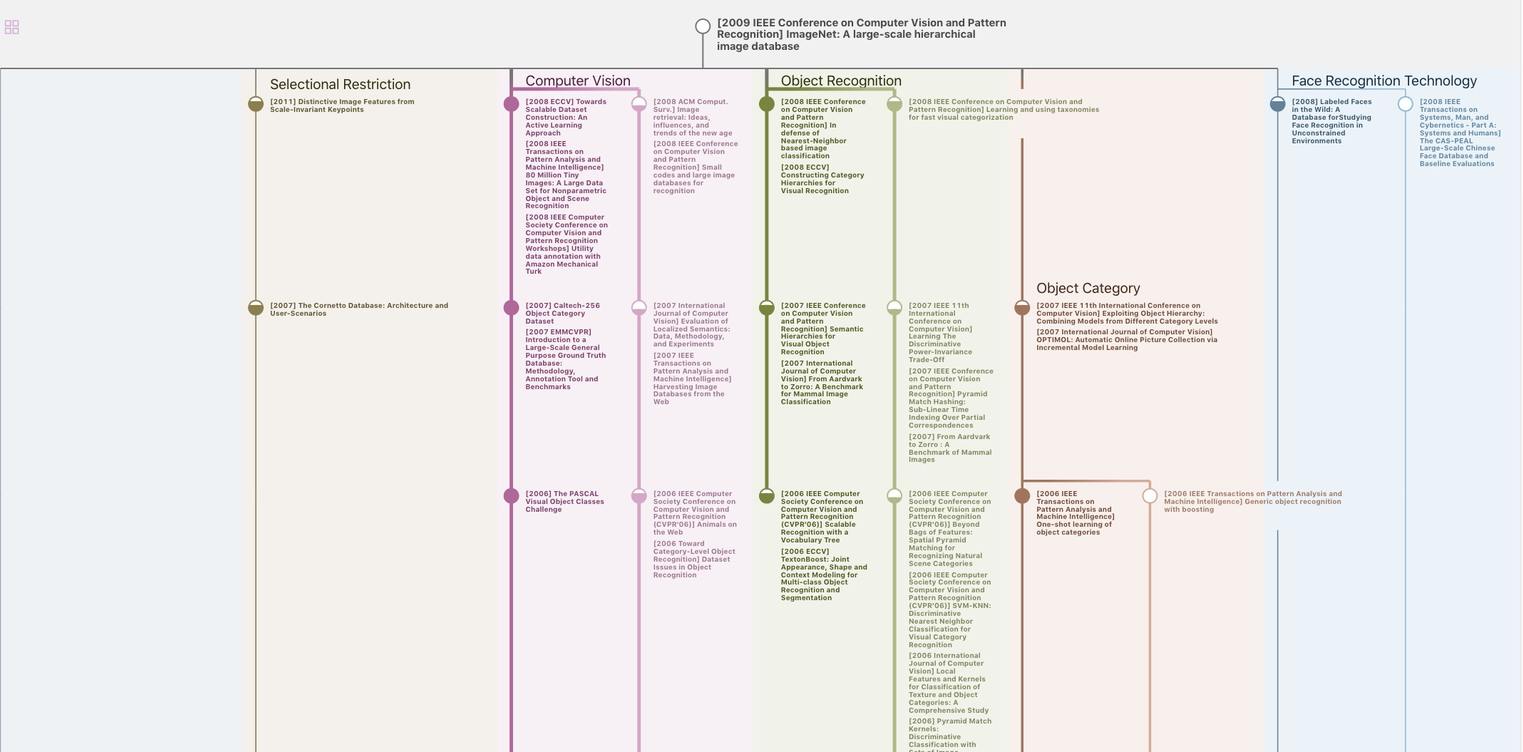
生成溯源树,研究论文发展脉络
Chat Paper
正在生成论文摘要