Multi-Agent Deep Reinforcement Learning for Dynamic Laser Inter-Satellite Link Scheduling
IEEE CONFERENCE ON GLOBAL COMMUNICATIONS, GLOBECOM(2023)
摘要
Laser inter-satellite links (LISLs) enable longerrange communication and cross-satellite dynamic links that bypass intermediate satellites. However, the utilization of narrow laser beams necessitates closed-loop control for alignment and consumes substantial energy even during idle periods. Therefore, we propose a dynamic LISL scheduling algorithm and a satellite link pattern with one dynamic LISL and three fixed LISLs to optimize energy consumption and reduce communication delay. To simplify the computation complexity of the optimization problem, a Markov decision process (MDP) is constructed, and the problem is divided into the independent decision process of each agent by breaking down the state space, action space, and reward function. Experimental results indicate that the proposed method reduces communication delay by approximately 2 hops and saves over 15% of energy consumption compared to fixed link patterns.
更多查看译文
关键词
Laser inter-satellite link,dynamic link,multiagent deep reinforcement learning,link scheduling
AI 理解论文
溯源树
样例
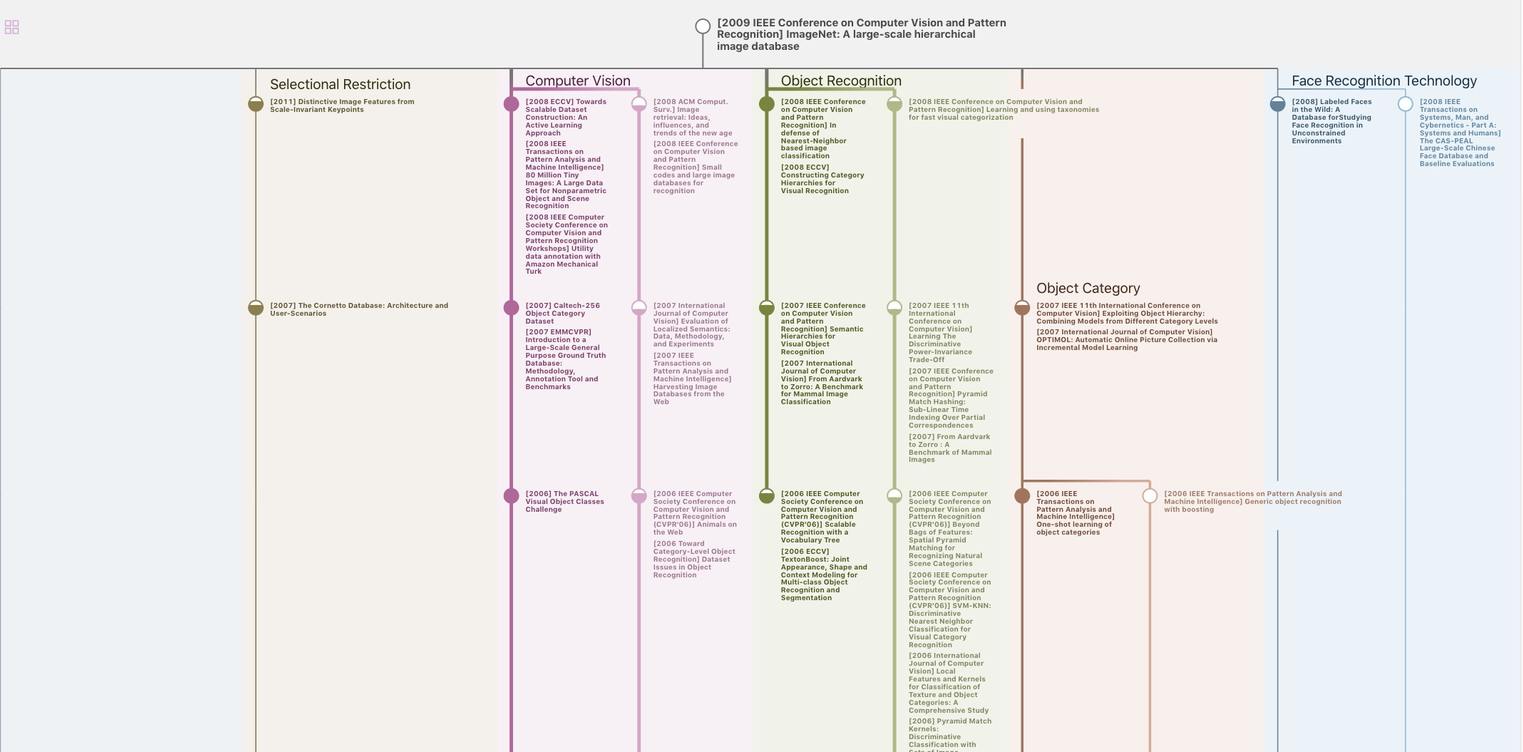
生成溯源树,研究论文发展脉络
Chat Paper
正在生成论文摘要