Long-Term Prediction of Remaining Useful Life for Industrial IoT
IEEE CONFERENCE ON GLOBAL COMMUNICATIONS, GLOBECOM(2023)
摘要
Industrial Internet of Things (IIoT), a branch of the Internet of Things (IoT) for the industrial sector, plays a vital role in integrating industrial equipment, monitoring equipment health, and improving the overall efficiency of industrial production process. Accurately predicting the remaining useful life (RUL) of IIoT equipment is a crucial task in prognostic health management (PHM), which analyzes the degradation trend of industrial equipment to schedule maintenance activities in a timely manner. Artificial Intelligence (AI) techniques, such as Recurrent Neural Networks (RNNs) and Long Short-Term Memory Networks (LSTMs), have been widely used in RUL prediction. However, these techniques face challenges in incorporating long-sequence information to capture degradation trends and predicting long-term RUL values. In this paper, we propose an Informer-based method, Co-Informer, for long-term RUL prediction. Co-Informer utilizes a series of sensor data to provide the predicted RUL values during an upcoming time window. In our research, extensive experiments are carried out with C-MAPSS, a widely used turbofan engine degradation dataset provided by NASA. Our experimental results indicate that Co-Informer outperforms the state-of-the-art schemes for RUL prediction in terms of Mean Squared Error (MSE), Root Mean Squared Error (RMSE), and Mean Absolute Error (MAE).
更多查看译文
关键词
Remaining Useful Life Prediction,Informer,Industrial IoT,Turbofan Engine
AI 理解论文
溯源树
样例
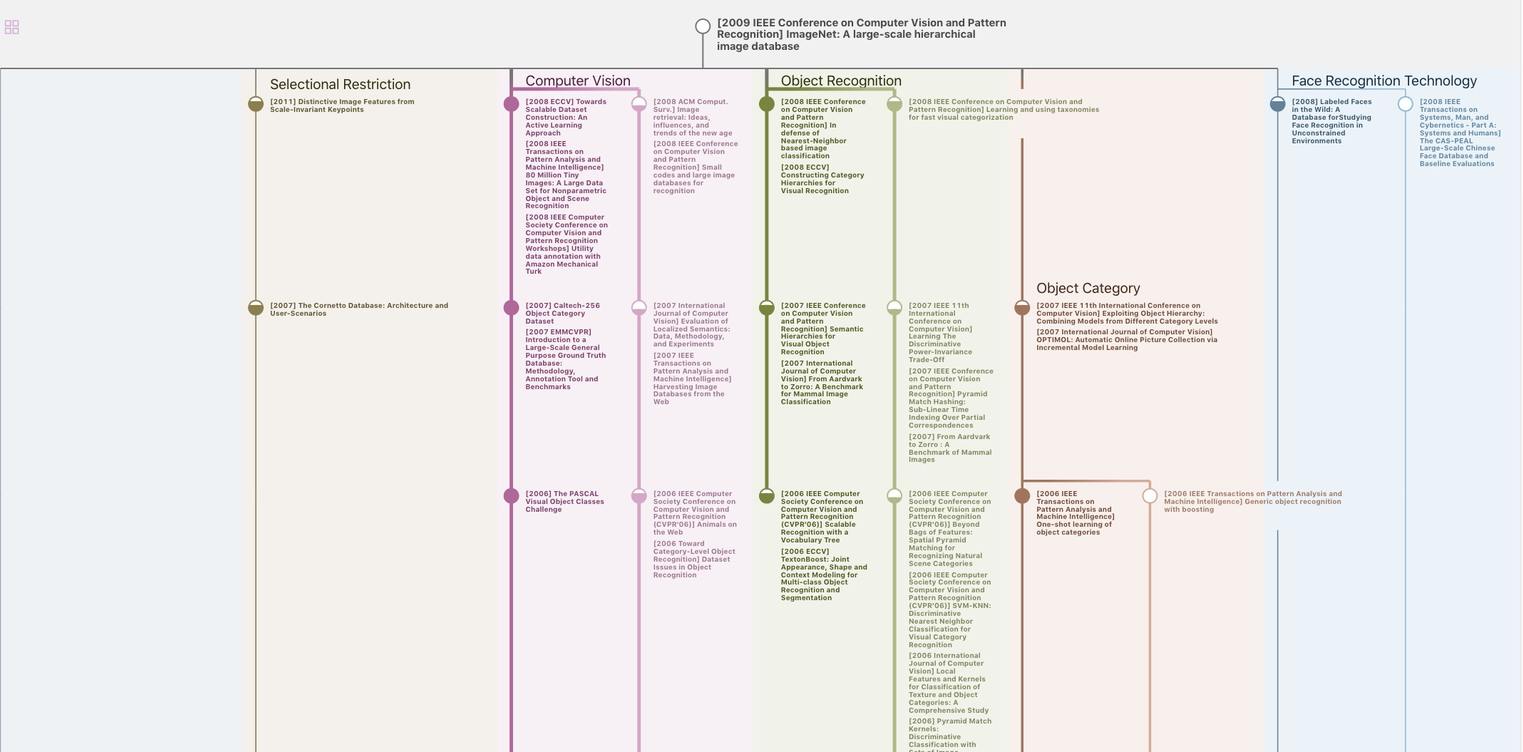
生成溯源树,研究论文发展脉络
Chat Paper
正在生成论文摘要