Caching for Edge Inference at Scale: A Mean Field Multi-Agent Reinforcement Learning Approach
IEEE CONFERENCE ON GLOBAL COMMUNICATIONS, GLOBECOM(2023)
摘要
To enable AI-empowered Internet-of-things (AIoT) applications, it is crucial to achieve real-time data inference (e.g., prediction, control) at network edge. However, resource-constrained Internet-of-things devices (IoTDs) may be incapable of accomplishing those computation-intensive and latency-sensitive inference tasks. To address this issue, it is promising to incorporate mobile edge computing (MEC) systems and let IoTDs offload their inference tasks to edge servers that have already cached the associated neural network model required for inference. In this work, we take into account the limited storage and computing capacity of edge servers and formulate a neural network model caching problem for an MEC system with edge inference, in order to maximize the inference accuracy and reduce the task delay. To handle the exponential growth of signaling overhead and the learning difficulty under huge number of widely-deployed edge servers, we propose a cooperative mean field multi-agent reinforcement learning framework and a mean field actor-critic algorithm to solve the aforementioned problem. Simulation results show that our proposed algorithm outperforms several benchmarks, especially in large-scale edge networks.
更多查看译文
关键词
Mobile edge computing,task offloading,model caching,mean field multi-agent reinforcement learning
AI 理解论文
溯源树
样例
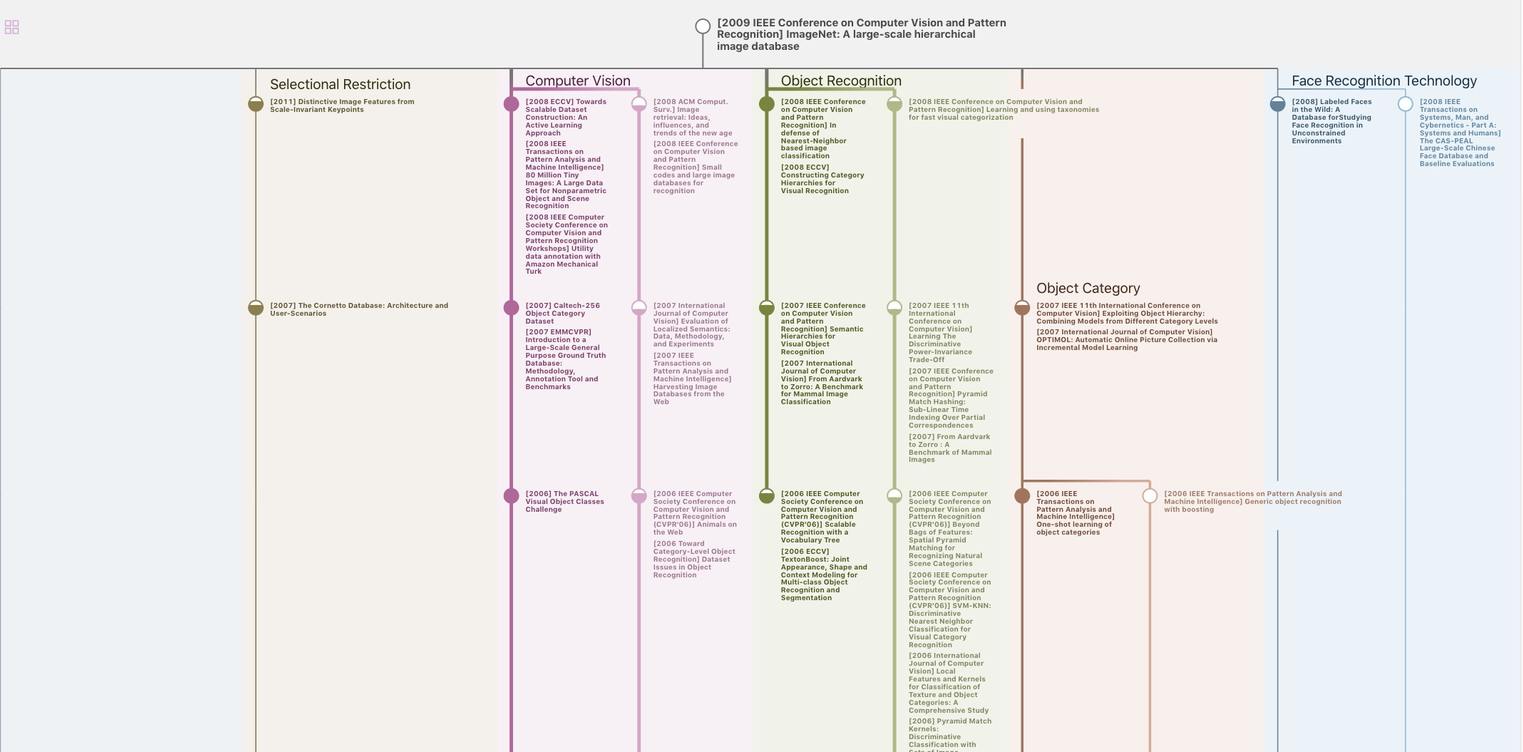
生成溯源树,研究论文发展脉络
Chat Paper
正在生成论文摘要