Training-Free Cost-Efficient Compression for Massive MIMO Channel State Feedback.
Global Communications Conference(2023)
摘要
Acquiring downlink channel state information (CSI) at basestation (gNB) is crucial for optimizing performance in massive MIMO FDD systems. Deep learning (DL) architectures have shown successes in enabling UE-side CSI feedback and gNB-side recovery, but often lack flexibility and/or require volumes of customized training data for specific RF channel environments and compression ratios. This work proposes a new CSI feedback architecture called zero-replacement (ZR). ZR is free from customized training and can be directly applied to new and unseen channel scenarios without pre-training and/or customization. It is also scalable and simple to implement, making it suitable for practical massive MIMO wireless deployment. We further generalize a Select-ZR algorithm, which switches between different sparse transformation techniques to enhance recovery performance. Our numerical results demonstrate that both proposed ZR and Select-ZR algorithms achieve competitive CSI recovery accuracy and feedback efficiency across various channels against highly complex data-driven DL models.
更多查看译文
关键词
Compressive feedback,model-free,massive MIMO,CSI recovery
AI 理解论文
溯源树
样例
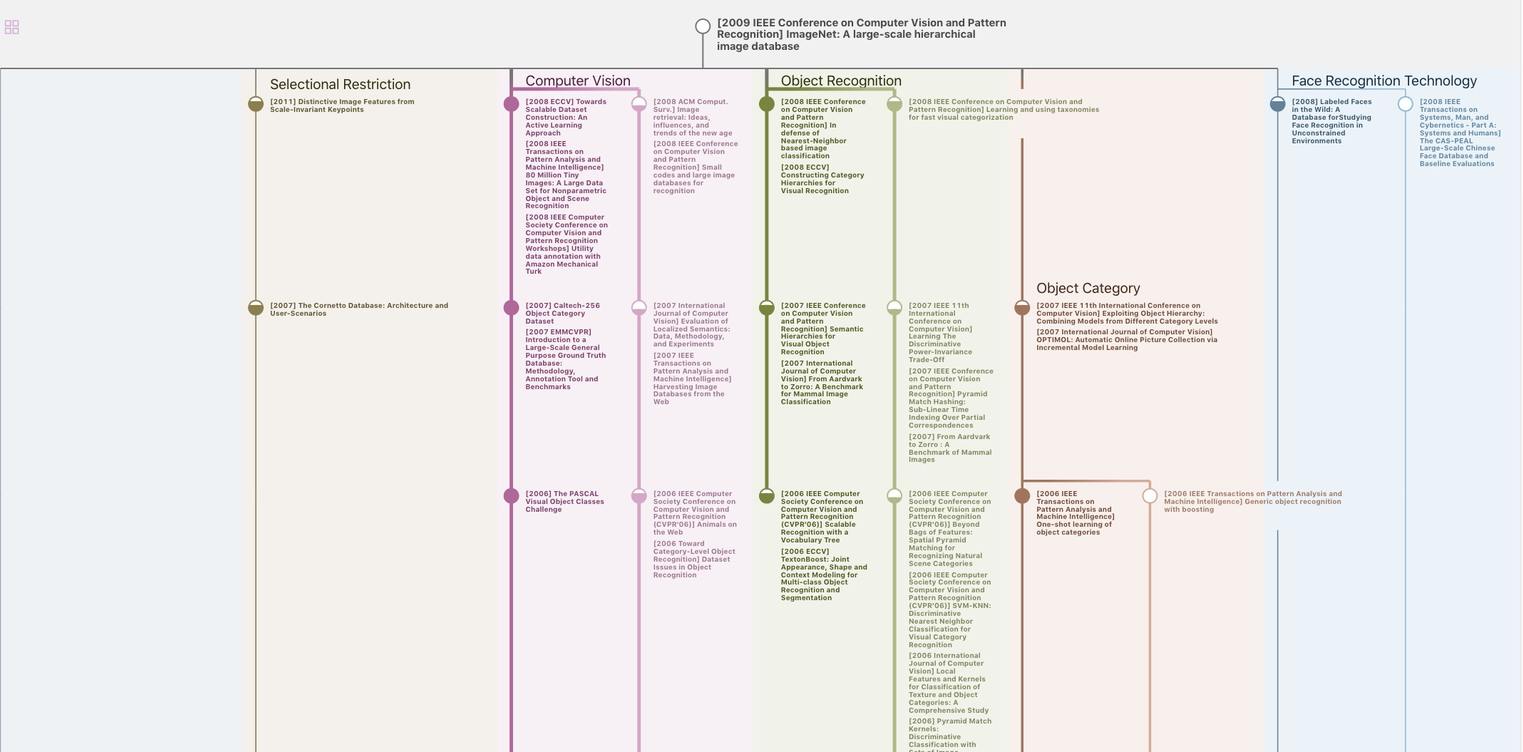
生成溯源树,研究论文发展脉络
Chat Paper
正在生成论文摘要