An Innovative Information Theory-based Approach to Tackle and Enhance The Transparency in Phishing Detection
arxiv(2024)
摘要
Phishing attacks have become a serious and challenging issue for detection,
explanation, and defense. Despite more than a decade of research on phishing,
encompassing both technical and non-technical remedies, phishing continues to
be a serious problem. Nowadays, AI-based phishing detection stands out as one
of the most effective solutions for defending against phishing attacks by
providing vulnerability (i.e., phishing or benign) predictions for the data.
However, it lacks explainability in terms of providing comprehensive
interpretations for the predictions, such as identifying the specific
information that causes the data to be classified as phishing. To this end, we
propose an innovative deep learning-based approach for email (the most common
phishing way) phishing attack localization. Our method can not only predict the
vulnerability of the email data but also automatically learn and figure out the
most important and phishing-relevant information (i.e., sentences) in the
phishing email data where the selected information indicates useful and concise
explanations for the vulnerability. The rigorous experiments on seven
real-world diverse email datasets show the effectiveness and advancement of our
proposed method in selecting crucial information, offering concise explanations
(by successfully figuring out the most important and phishing-relevant
information) for the vulnerability of the phishing email data. Particularly,
our method achieves a significantly higher performance, ranging from
approximately 1.5
by the combined average performance of two main metrics Label-Accuracy and
Cognitive-True-Positive.
更多查看译文
AI 理解论文
溯源树
样例
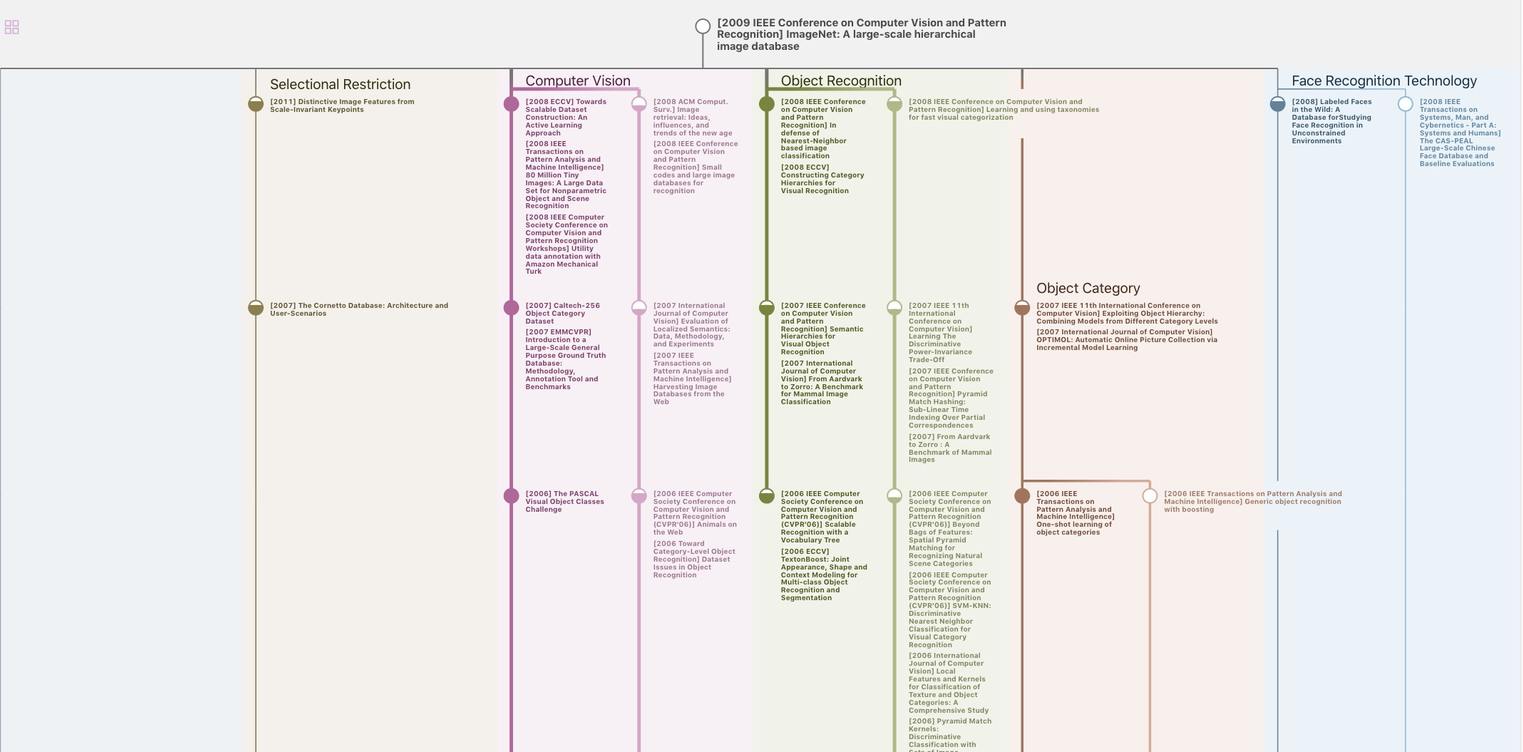
生成溯源树,研究论文发展脉络
Chat Paper
正在生成论文摘要