Deep Reinforcement Learning for Enhancing the Secrecy of a MU-MISO UOWC Network.
Global Communications Conference(2023)
摘要
In this paper, we propose a Deep Reinforcement Learning (DRL) framework to optimize the secrecy performance of a Multi-User (MU)-Multiple-Input Single-Output (MISO) Underwater Optical Wireless Communication (UOWC) system. The network consists of several light-emitting diodes connected with various underwater users through optical beams. The legitimate transmission is threatened by several eavesdroppers attempting to overhear the confidential message sent to each user. Thus, digital precoding is employed to cancel the inter-user interference and maximize the per-user secrecy rate and, consequently, the secrecy sum rate (SSR). Leveraging the developed DRL algorithm, the MU-MISO precoding matrix is optimized for enhancing the system's SSR. Numerical results show the superiority of the proposed DRL framework compared to the baseline zero-forcing and random pre coding schemes, even with corrupted CSI at the transmitter due to seawater dynamics and estimation errors.
更多查看译文
关键词
Deep Reinforcement Learning,Underwater Wireless Optical Communication,Estimation Error,Wireless,Light-emitting Diodes,Light Beam,Wireless Communication Systems,Deep Reinforcement Learning Algorithm,Multiple-input Single-output,Inter-user Interference,Deep Reinforcement Learning Framework,Secrecy Performance,Optical Wireless Communication,Model System,Time Step,Visible Light,Deep Neural Network,Photodetector,Multiple-input Multiple-output,Optimal Policy,Imperfect Channel State Information,Proximal Policy Optimization,Discrete Action,Thermal Noise,Precoding Vector,Optical Signal,Markov Decision Process,Legitimate Users,Non-negativity Constraints,Reinforcement Learning Agent
AI 理解论文
溯源树
样例
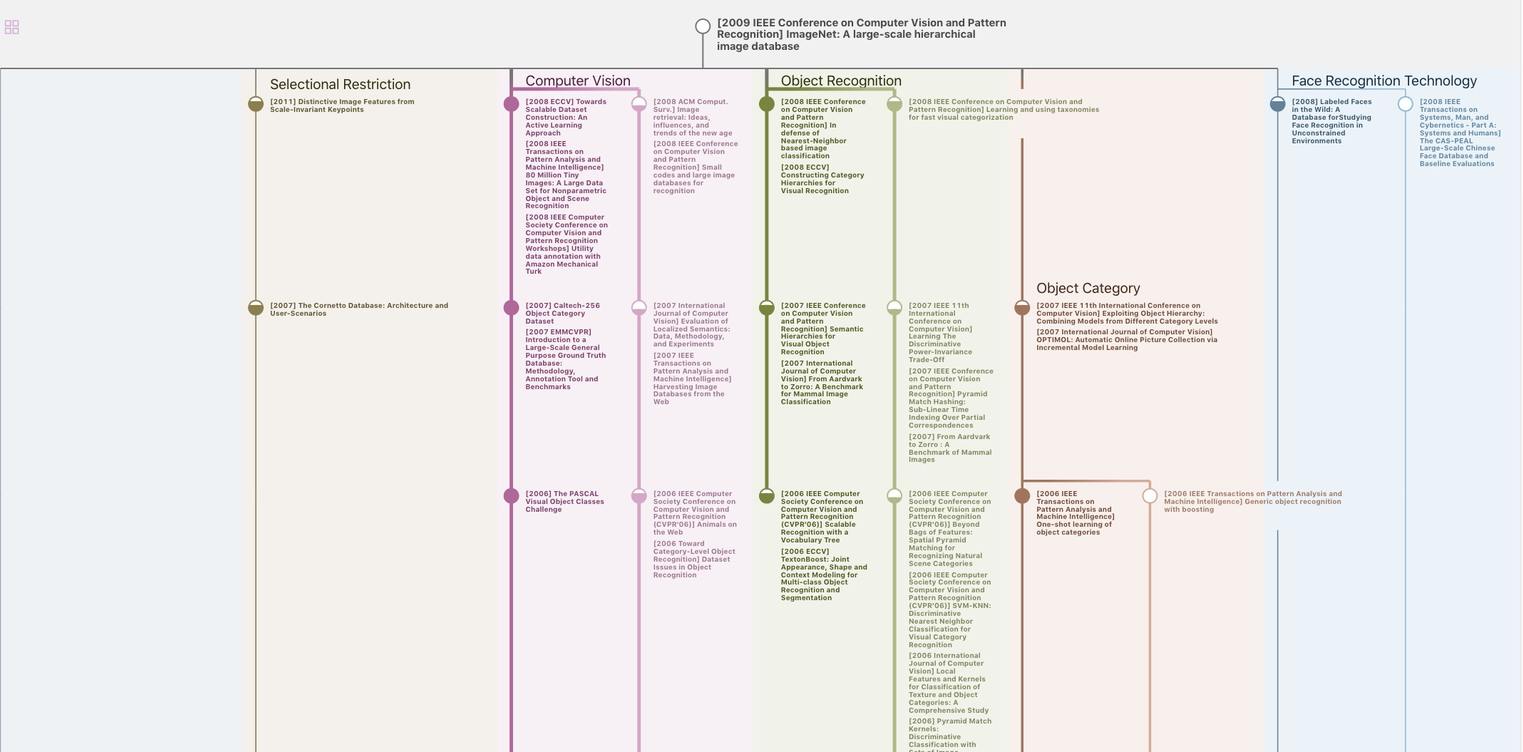
生成溯源树,研究论文发展脉络
Chat Paper
正在生成论文摘要